Introduction
Many regions in developing countries lack adequate access to formal information sources, which is why information is spread through social networks (Saint Ville et al. 2016; Songsermsawas et al. 2016; Rink & Wong-Grünwald 2017). This channel of knowledge dissemination is especially important for resource-poor small-scale farmers. They rely on informal information sources since sharing information through word-of-mouth communication is convenient, reduces transaction costs, and is easily accessible (Feder, Just & Zilberman 2004; Matuschke 2008). Access to information is particularly important in the context of adopting innovations, which have high potential to improve farmers’ productivity and adaptation abilities (Pratiwi & Suzuki 2017; Vasilaky & Leonard 2018). Farmers might decide to adopt an innovation only if they are aware of it and have sufficient knowledge about its benefits and application. Consequently, access to information increases the likelihood of innovation adoption (Rogers 1983; Khonje 2015; Xiong et al. 2018).
However, when designing interventions to disseminate knowledge, policy-makers and program-implementers often face limited resources that obstruct the provision of information to every farmer individually. Consequently, they commonly target only a subset of farmers who receive the information initially. They then rely on the initially informed farmers, who are referred to as ‘seeds’, to spread the knowledge within their community. Because all other farmers depend on the seeds to obtain the information through their network, seeds are crucial to the success of the diffusion process (Genius et al. 2014, Magnan et al. 2015; D’Angelo, Severini & Velaj 2017). Choosing a subgroup of farmers as seeds therefore raises the question of how to identify the actors with the highest diffusion potential (Erlandsson, Bródka & Borg 2017).
Many studies examine the diffusion of innovations and thereby acknowledge information as an essential prerequisite for adoption, but research addressing the improvement of information spread remains limited. Some studies focus on the role of seed selection on effective information dissemination, but they predominantly analyse centrality measures as seed selection criteria exclusively, which account for local hierarchical structures only indirectly, or develop approximation algorithms to maximize spread. Furthermore, due to the paucity of empirical data many studies follow a theoretical approach. Empirical research is particularly limited in the context of developing countries despite the high relevance of information spread through word-of-mouth communication in rural economies (Muller & Peres 2019).
To address these limitations in research, this paper focusses on the diffusion of information as a precondition of innovation adoption. By systematically evaluating performance of different seed sets, the study aims to improve information dissemination through optimising seed selection. Specifically, the present research evaluates information of low complexity, such as awareness of the existence of an agricultural innovation, which can be transmitted via single encounters between farmers and thus spreads like a simple contagion (Centola 2018). We focus on word-of-mouth communication as the mean for information sharing between farming households. By adjusting an agent-based model (ABM) to a case study in rural Zambia, the study provides empirical evidence while accounting for context-specific dynamics and heterogeneity of farmers. The ABM systematically assesses the outcomes of various seeding strategies à priori to support the design of diffusion processes (Scheller, Johanning & Bruckner 2018; Barbuto, Lopolito & Santeramo 2019; Dijkxhoorn et al. 2019). Thus, this study provides policy-makers and development practitioners with insights on the importance of seeding strategies for diffusion processes prior to the intervention implementation. Results are specifically relevant for applications in rural areas of developing countries where social networks are the main source of information and projects depend on seeds to disseminate knowledge. In particular, the paper takes the following steps. Firstly, it systematically assesses centrality measures as seed selection criteria with respect to their impact on the speed and reach of information diffusion. Additionally, it tests hierarchy (village heads) as a seed selection criterion. Secondly, the paper examines the influence of the number of seeds on the diffusion process. Thirdly, a robustness check analyses how the success of the seed selection criteria depends on the number of seeds. The analysis confirms that optimising the seeding strategy with respect to selection criteria and size strongly impacts the diffusion success.
The remainder of this paper is structured as follows: Section 2 presents the state of the literature. The consequent section describes the study area and data, followed by the outline of the ABM in Section 4. Section 5 presents and discusses the simulations results, and Section 6 summarizes and concludes.
Literature Review
A number of studies confirm that social networks affect farmers’ decisions to adopt innovations (e.g., Foster & Rosenzweig 1995; Conley & Udry 2001; Matuschke & Qaim 2009; Mekonnen, Gerber & Matz 2018; Xiong et al. 2018). Whereas most investigations focus on the process of adoption diffusion, some researchers also assess the information effect of social networks (e.g., Cadger et al. 2016; Songsermsawas et al. 2016; Mekonnen, Gerber and Matz 2018; Xiong et al. 2018; Shikuku 2019). Studies such as Anderson & Feder (2004) confirm that endowment with human capital is frequently linked to farmers’ performance because innovation adoption requires access to information. Especially in rural areas of developing countries, farmers rely on their social networks to access knowledge which in turn influences adoption decisions (Foster & Rosenzweig 1995; Conley & Udry 2001; Bandiera & Rasul 2006; Saint Ville et al. 2016). Social learning between farmers influences the diffusion process in all phases, but is particularly important in the early stages (Xiong et al. 2018). Therefore, social networks are of major relevance for information and innovation diffusion processes due to their function as informal communication channels (Isaac et al. 2007; Matuschke 2008; Banerjee et al. 2013; Muange, Schwarze & Qaim 2014; Magnan et al. 2015).
The extent to which a social network supports information flow depends on its characteristics (Qiao et al. 2019) as network structure influences information spread (Morone & Taylor 2004a; Manson et al., 2016). Watts & Strogatz (1998), Cowan & Jonard (2004), and Morone & Taylor (2004b) find that information spreads quickest if the network topology assimilates a small world. In contrast, Jang et al. (2019) state that a random network has the optimal structure for knowledge diffusion. Besides, network size, density, and degree distribution can influence diffusion processes (Morone & Taylor 2004a; Delre, Jager & Janssen 2007; Mueller et al. 2017; Bogner, Müller & Schlaile 2018). Several authors also emphasize the role of weak ties for connecting communities and thereby preventing local trapping of information (Granovetter 1973; Zhao et al. 2010). Overall, the specific network structure is an important factor for the diffusion of information (Barbuto, Lopolito & Santeramo 2019; Qiao et al. 2019).
Besides network characteristics, traits at the individual farmer level such as gender, age, education, and land size affect the exchange of information (van den Broeck & Dercon 2011; Kabunga, Dubois, and Qaim 2012; Muange, Schwarze & Qaim 2014; Cadger et al. 2016; Mekonnen, Gerber and Matz 2018). Generally, group homogeneity intensifies information transmission due to homophily effects (van den Broeck & Dercon 2011; Shikuku 2019).
In addition, the likelihood of receiving relevant information depends on the farmer’s centrality in the network (Hinz et al. 2011; Muange, Schwarze & Qaim 2014; Pratiwi & Suzuki 2017). To identify well-connected actors with high abilities to exchange information, social network theory provides several centrality measures such as degree, closeness, betweenness, and eigenvector centrality (Lü et al. 2016; Borgatti, Everett & Johnson 2018). Degree centrality equals the number of connections an actor has in the network. The inverse sum of distances of an actor to all other actors in the network describes closeness centrality, which represents how close an actor is to all others in the network. Betweenness centrality indicates the frequency with which an actor is on the shortest path between any combination of two actors in the network. Actors with high betweenness function as bridges between communities and are more likely to influence people from different groups. Eigenvector centrality is based on the idea that each actor’s centrality depends on the weighted average of the actors with whom it is connected (Hussain et al. 2013; Borgatti, Everett & Johnson 2018). A variety of studies confirms that network centrality is positively associated with enhanced information sharing capabilities (Hinz et al. 2011; Muange, Schwarze & Qaim 2014; Pratiwi & Suzuki 2017; Muller & Peres 2019).
Because diffusion success depends on the initially informed farmers, network centrality is an essential aspect for seed selection (Valente & Davis 1999; Akbarpour, Malladi & Saberi 2018; Kowalska-Styczen, Malarz & Paradowski 2018; Barbuto, Lopolito & Santeramo 2019). The literature has proposed algorithms to identify actors that maximize spread (e.g. Kempe, Kleinberg & Tardos 2003; Wang et al. 2014; D’Angelo, Severini & Velaj 2017). In practice, however, well-connected or popular persons are commonly selected as seeds (Delre et al. 2010; Mochalova & Nanopoulos 2013; Banerjee et al. 2014). Seeding strategies have been analysed in contexts such as diffusion of microfinance (Banerjee et al. 2013) and adoption of agricultural technologies (Beaman et al. 2018).
Not only do seed selection criteria matter, but also the number of seeds is relevant for a successful diffusion process since a larger number of seeds leads to greater reach (Bampo et al. 2008; Barbuto, Lopolito & Santeramo 2019). Expanding the number of randomly chosen seeds can even outperform centrality-based seed selections (Akbarpour, Malladi & Saberi 2018). Additionally, the interaction between the number of seeds and centrality measures chosen as criteria for seed selection affects the diffusion success (Mochalova & Nanopoulos 2013). Overall, the literature shows that seeding strategies play an important role for diffusion processes, but detailed studies comparing criteria and considering interdependencies in an empirical context remain limited.
Study Area and Data
The model is adjusted to a study area called Mantapala, which is located within the Congo Basin in northern Luapula Province of Zambia as illustrated in Figure 1. The study area was selected within the framework of the project Food Security in Rural Zambia (FoSeZa), funded by the German Federal Ministry of Food and Agriculture (BMEL). According to stakeholder discussions and results of a pilot survey, the region shows typical features of a rural village in a developing country such as a remote location, lack of infrastructure development (roads, electricity, market integration, etc.), pronounced malnutrition, and food insecurity (Hampwaye, Jeppesen, and Kragelund 2014; Central Statistical Office 2016; Gronau, Winter & Grote 2018). Given the distinct impoverishment combined with high food insecurity and dependence on farming, research regarding the spread of agricultural innovations can contribute to improving local livelihoods (Hampwaye, Jeppesen & Kragelund 2014; Central Statistical Office 2016, 2018; Gronau et al. 2018).
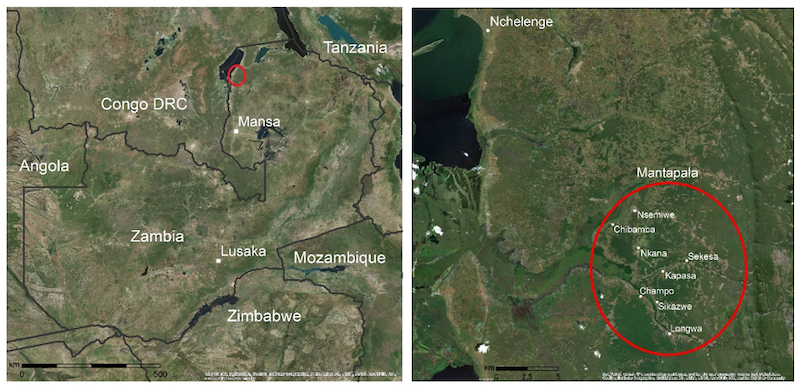
The household characteristics derived from the survey data displayed in Tables 1-2 illustrate the poverty, food insecurity, low levels of education, and agriculturally oriented livelihoods that prevail in the study area.
Characteristics | Number of households | Share of households (in %) |
Female headed households | 54 | 20 |
Farming as the main income source of household head | 234 | 90 |
Member of an agricultural, livestock, producer group | 42 | 16 |
Households with poor food security according to food consumption index | 55 | 21 |
Households with borderline food security according to food consumption index | 61 | 23 |
Households with acceptable food security according to food consumption index | 148 | 56 |
The study area consists of eight villages, which are overseen by seven village heads. Although the study area is rather isolated, exchange in terms of communication and economic activities amongst the eight villages takes place, offering interesting potential for social network analysis. Each village consists of about ten to 80 households summing up to 264 households. A structured household survey was conducted in 2018 as part of a census. Thus, the data set covers all households in the study area. In addition to sections on socio-economic characteristics, agricultural activities, and food security amongst other, the interviews contained a comprehensive segment on social capital, which provides information about the social network in the study area, which households exchange agricultural information, and how frequently they do so. Furthermore, GPS-data of the households were collected.
Characteristics | Mean |
Age of household head (in years) | 44 (14.65) |
Level of education of household head (in years) | 6.9 (2.98) |
Yearly household income (in Zambian Kwacha) | 3871.26 (4530.31) |
Land size owned (in hectare) | 6.80 (5.72) |
Household size | 6.03 (2.36) |
Methodology: The Information Diffusion Model
The present agent-based model simulates information diffusion through word-of-mouth communication between farming households in rural Zambia to identify seed sets with high potential for information spread. The following section provides an overview over the ABM, and the Appendix contains the full description in detail.
The households: The acting entities in the model are farming households, who exchange agricultural information. Variables indicating both location and hierarchical status describe the households. Households can be connected via links, which include data specifying whether and how frequently the respective households discuss agricultural matters. Data to initialize the households and the network origin from a household survey conducted in 2018 in Zambia.
Information transmission: The model employs an empirical approach to simulate transmission of agricultural information between households. Thereby, the diffusion is modelled as a simple contagion process, where information can be successfully transmitted through a single contact between farming households (Centola 2018). Each time step, information exchange between households takes place as follows: the households already informed randomly choose another household with which they share an information link. If the selected household has not yet obtained the information, the acting household transmits the information based on a probability that increases with higher frequency of agricultural discussions between these households, as indicated in the survey. The newly informed households then update their status accordingly.
Each model run starts with the initialization of the 264 households and the social network, which remains static over the course of the simulation. Initially, only those households selected as seeds set their state to “informed”, whereas all other households are “uninformed”. The model proceeds in weekly time steps. As information is assumed to lose relevance after a certain point in time, the time frame is set to one year, limiting the simulation to 52 steps.
Model output: The model output includes data regarding reach (total number of households informed) and speed (rate of diffusion, number of households informed monthly). Reach provides information on final spread; speed provides information on progress over time.
Scenarios: The analysis distinguishes between three scenarios to find the most eligible set of seeds for widespread and rapid information diffusion within a social network.
- The first scenario examines the impact of the following selection criteria for seeds on the diffusion process: i) random ii) hierarchy (village heads) iii) degree centrality iv) closeness centrality v) betweenness centrality and vi) eigenvector centrality. In this first scenario, the number of seeds is eight, which corresponds to approximately 3% of the population (Leeuwis 2004; Natcher et al. 2016). If the seeds are selected according to hierarchy, the total number of seeds is seven, which equals the number of village heads in the study region.
- The second scenario assesses the effect of the number of seeds on the diffusion process by varying it from two to 26 by increments of two, corresponding to 1 to 10% of the total population (Bampo et al. 2008; Erlandsson, Bródka & Borg 2017). Seeds selection is random.
- In order to test robustness of the results, scenario 3 examines the interaction effects between the selection criteria and number of seeds by simultaneously changing the seed set size and selection criteria.
Model calibration and verification: The applied model provides generic insights into how information spreads in a sparse social network. To ensure consistency with reality, the model parameters such as network structure and interaction behaviour are based on survey data from a case study in rural Zambia. A careful scan of the code was carried out whilst discussing it amongst researchers. Additionally, extreme values of the input variables were implemented to test corner cases. The simulation was run with at least 30 repetitions for each specific scenario.
Robustness tests: In order to test the dependence of the results on the specific network, the static network, as derived from the survey, was varied by changing the total number of links in the network during initialization (random addition and deletion of 5% and 10% of the existing links). Furthermore, 5% and 10% of existing links were rewired while keeping the total number of links constant to test for the results’ dependence on network properties and transferability.
Data analysis: The results of the simulations were analysed by applying t-tests and one- and two-way Analysis of Variance (ANOVA) statistics using Stata 14.
Results
The following chapter presents descriptive findings and simulation results. The presentation of the simulation results is structured according to the three scenarios.
Descriptive results
The subsequent section presents selected descriptive findings with respect to the social network in the study area. Further seed-specific network descriptives are included in the Appendix.
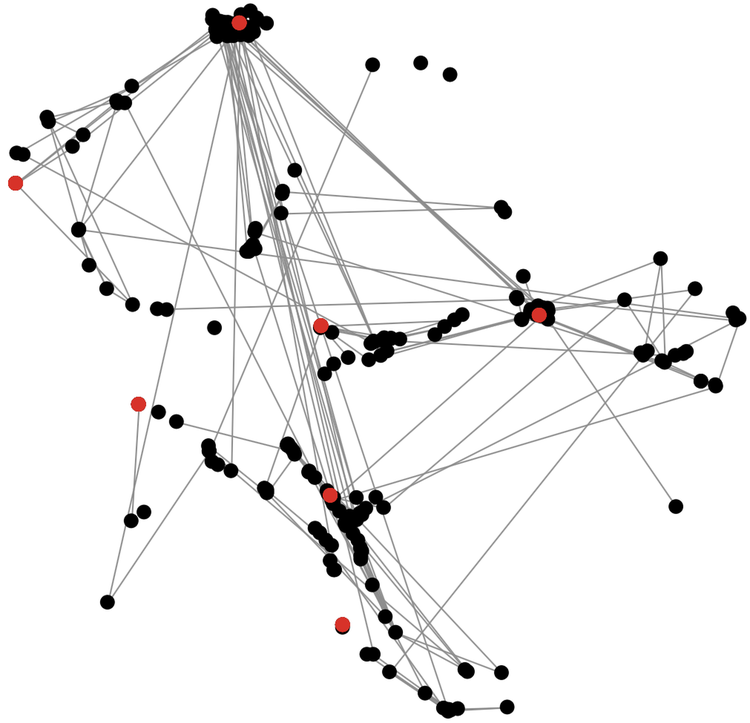
The household heads have on average 1.7 connections to other households in the study area as visualized in Figure 2. Connections are links between farmers that the respondent perceived as important for business or personal reasons. The low number of connections results in an overall network density of 0.01 implying low trust in the study area. This result is in line with Leavy (2015), who also does not find large networks in rural Zambia. On average, closeness centrality is 0.21, betweenness centrality 447.05, eigenvector centrality 0.34, and the cluster coefficient 0.05. The average shortest path in the largest component is 8.33, and transitivity is 0.08. In the network, 180 households (69%) are connected in a big component, but disconnected from the 84 (32%) remaining households, who are partially connected with each other in smaller components. Most connections represent close friendships or are based on family relations as displayed in Table 3.
Type of connection | Absolute | Relative (in %) |
Number of connections that are blood relatives | 94 | 28 |
Number of connections that are related by marriage | 46 | 14 |
Number of connections that are best friends | 127 | 37 |
Number of connections that are friends | 40 | 12 |
Number of connections that are neighbours | 17 | 5 |
Despite the fact that the network is sparse and trust seems to be generally low in the study area, social networks nevertheless play a vital role for information exchange, and the existing connections are used to disseminate information as presented in Table 4.
Number of households / connections | Absolute | Relative (in %) |
Households relying mostly on family and friends for agricultural information | 115 | 44 |
Households relying mostly on experts and extension services for agricultural information | 77 | 29 |
Households relying mostly on village meetings for agricultural information | 23 | 29 |
Households relying mostly on media for agricultural information | 20 | 8 |
Connections exchanging agricultural advice | 296 | 87 |
Connections that discuss business several times per week | 142 | 42 |
Connections that discuss business several times per month | 92 | 27 |
Connections that discuss business several times per year or only when a decision is made | 46 | 13 |
Scenario 1: The impact of seed selection criteria
The following section presents the results of scenario 1, which tests the effects of different seed selection criteria on the diffusion process. As summarized in Table 5, seed selection based on degree centrality leads to highest final reach amongst all selection criteria with mean diffusion rates of 62.46% of the whole population. Hierarchy-based (62.20%), betweenness-based (62.08%), and random selection (60.61%) achieve very similar results. In contrast, seed selection based on closeness and eigenvector centrality leads to significantly lower reach with only a share of 17.55% and 18.93% of the population informed (p=0.00, degrees of freedom (DF)=5, F=821.92). Degree-, betweenness-, and hierarchy-based seeds lead to similar results in each simulation run, whereas random, closeness-, and eigenvector-based seeds fluctuate more between simulations.
Seed selection criterion | Number of informed households after one year | Share of households informed after one year (in %) |
Random | 160.2 (11.72) | 60.61 |
Village heads | 164.2 (5.40) | 62.20 |
Degree | 164.9 (3.38) | 62.46 |
Betweenness | 163.9 (4.15) | 62.08 |
Closeness | 46.3 (36.02) | 17.55 |
Eigenvector | 50.0 (54.27) | 18.93 |
As visualized in Figure 3, all seed selection criteria lead to distinct numbers of informed households during the first stages of the diffusion process (p=0.00, DF=5, F=1587.81 for timestep 8). However, results caused by selection based on hierarchy, betweenness, degree, and random choice converge at the end of the year. When about 60% of the population received the information, a saturation level seems to be reached. However, the distinct seed selection criteria attain this saturation level at different points in time. The graph shows that degree-based selection leads to the quickest spread.
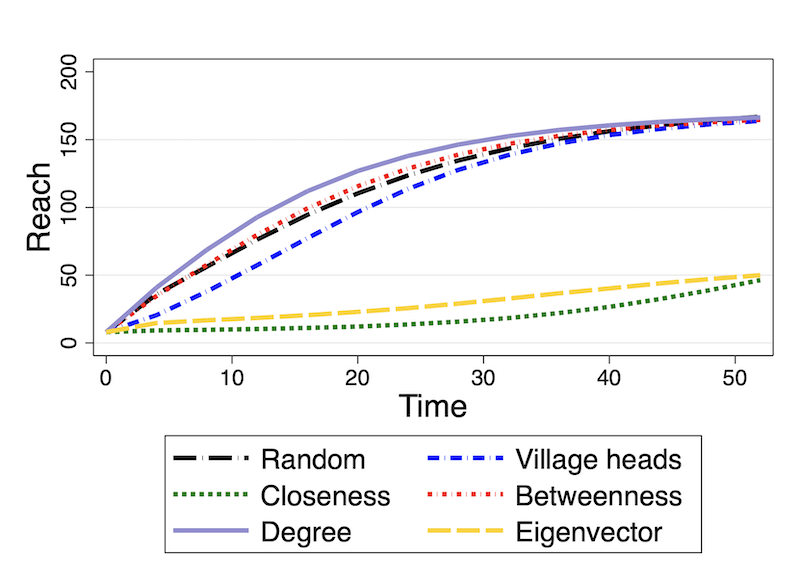
As most village heads are well connected in the network, hierarchy-based seed selection yields relatively good results. Despite the slightly lower number of seeds in the hierarchy-based scenario (seven compared to eight), reach does not significantly differ compared with betweenness-based (p=1.00), degree-based (p=1.00), and random seed selection (p=1.00) as the Bonferroni multiple comparison test shows (DF=5, F=821.97). In addition, random seed-selection performs quite well and yields similar results as degree-based, betweenness-based, or hierarchical-based seed selection.
Scenario 2: The impact of number of seeds
Scenario 2 investigates how the number of seeds influences spread over time. Hereby, the number of randomly chosen seeds is varied from 2 to 26 seeds, incrementally increased by two covering the span of circa 1% up to 10% of the population (Bampo et al. 2008; Erlandsson, Bródka & Borg 2017).
The ANOVA statistic examining the differences in seed set size shows that a higher number of seeds is positively associated with reach (p=0.00, DF=12, F=34.39). Furthermore, seed set size impacts the diffusion speed as the distinct slopes for different seed set sizes indicate (Figure 4). Relatively large seed set sizes increase speed in early stages of the diffusion process; relatively small seed set sizes enhance speed to a larger extent in later stages instead.
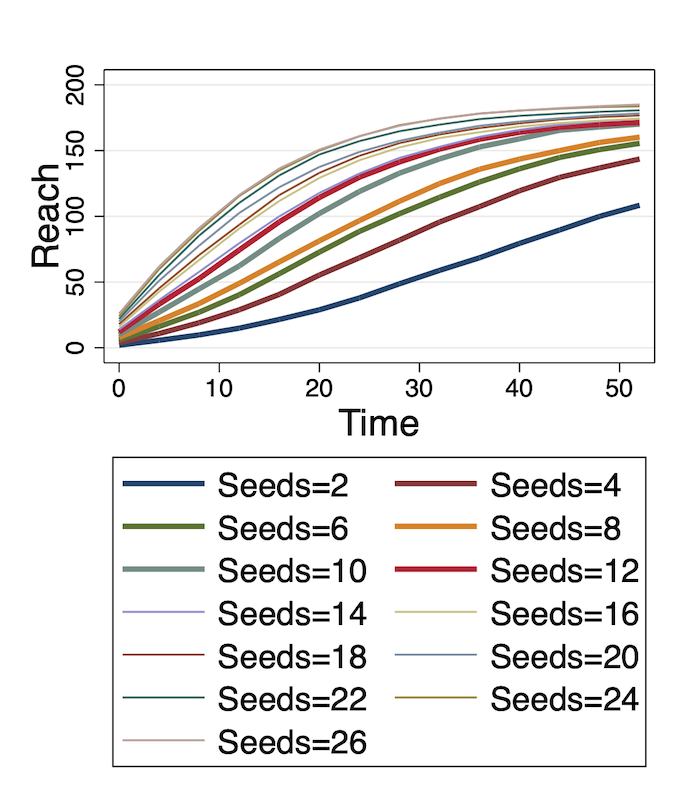
For smaller seed set sizes diffusion speed is lower at the beginning. Consequently, increasing small seed sets still significantly impacts reach by the end of the year (p=0.00 for increasing from 2 to 4 seeds in step 52), contrary to increasing rather large seed sets (p=1.00 for increasing from 20 to 22 seeds in step 52, DF=12, F=34.39). However, due to the less frequent information transmission in the beginning and resulting long take-off phase, small seed set sizes result in lower overall reach despite the higher speed at the end.
Scenario 3: Simulating interaction effects
Scenario 3 explores how the success of seed selection criteria depends on the number of seeds. By analysing the interaction effect between seed selection criteria and set size, robustness of the previous results is tested. The ANOVA analysis investigating seed set size, choosing mechanism as well as their interaction effects confirms that at all points in time the number of seeds, the selection criteria, and their interaction effect influence reach (p=0.00, DF=77, F= 626.64 in time step 52). The interaction effects, depicted in Figures 5-7, prove that random choice, betweenness-, and degree-based seed selection support the previous results from Scenario 2: for these selection criteria, an increase in seed set size leads to higher reach, but the effect of each additional seed on the diffusion success declines. In contrast, the performances of closeness- and eigenvector-based seed selection are not robust to the number of seeds since seed set size impacts the performances of seed selection based on these criteria. Thus, closeness- and eigenvector-based seed selection should be considered critically.
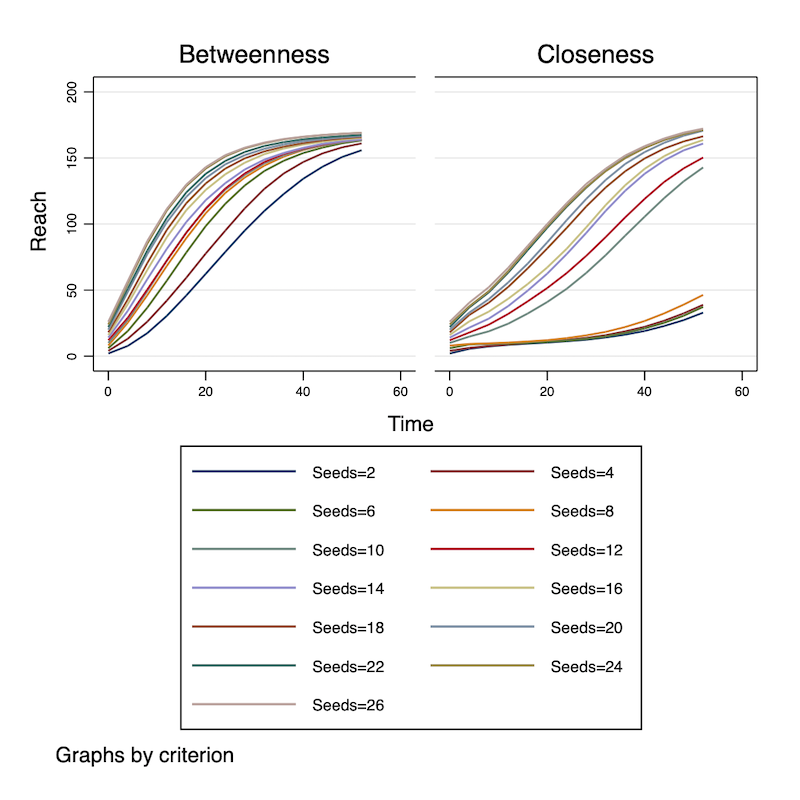
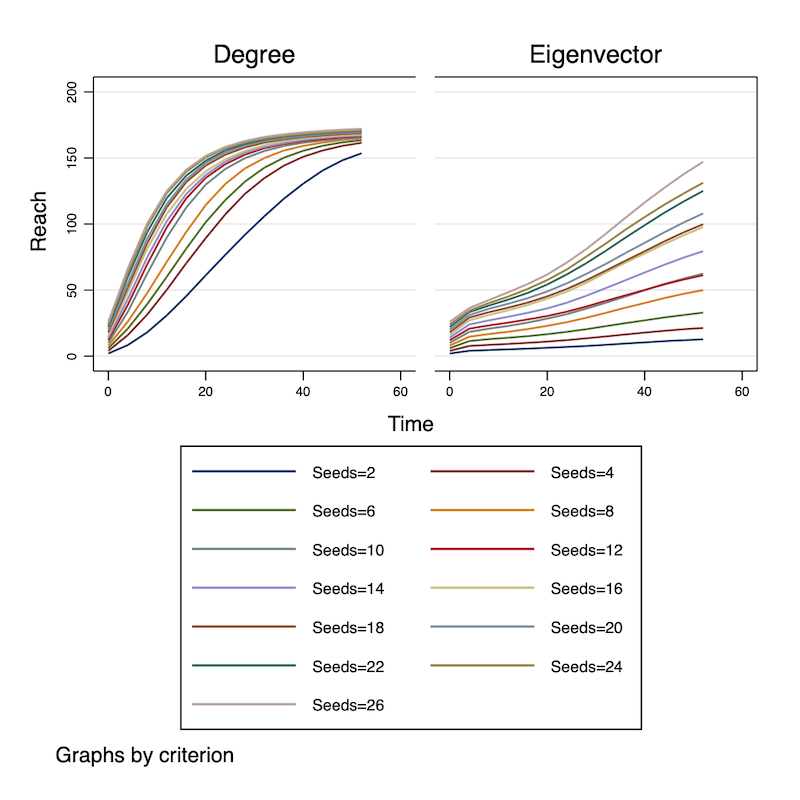
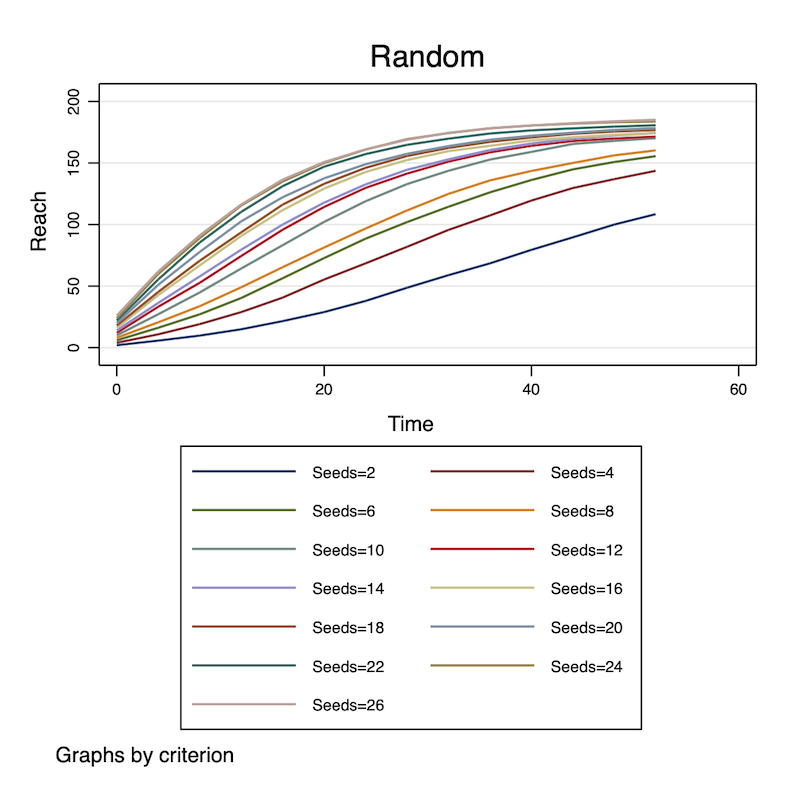
The simulations show that choosing village heads as seeds leads to quite high reach although the number of seeds is always restricted to seven (this restriction is the reason for excluding hierarchy from Figures 5-7). If, for example, the maximum seed set size of 26 households is chosen for the other selection criteria, this results in a difference of only 20 additional households informed at the end of the year compared to hierarchy-based seed selection. Thus, informing village heads seems quite efficient.
Robustness of the results
To test the robustness of the previous results, the network was systematically varied through rewiring 5% and 10% of the links, while the total number of links remained constant, and adding as well as deleting links (5% and 10%). The results show that while the number of links is positively associated with reach and speed, the general findings remain valid. Thus, the results prove to be robust towards small changes in the network and therefore demonstrate transferability of the derived policy recommendations for seeding strategies to other applications with similar network characteristics.
Discussion and Conclusions
Our analysis explored how the selection of farmers who receive information first (‘seeds’) influences knowledge diffusion in a sparse social network. An empirical ABM was implemented and adjusted to a case study area in rural Zambia. Our results provide policy makers and development practitioners with insights into how seeding strategies can support the promotion of innovations, while making use of social diffusion processes in rural communities in developing countries. Our study emphasizes the role of adequate seed selection strategies, which take into account both the seed selection criterion and the set size for enhancing information dissemination.
Scenario 1 showed that selection of farmers with most connections (highest degree centrality) improves the speed and reach of diffusion the most. Selecting farmers with high betweenness centrality, who link network components, or village heads, who also are well connected, significantly contributes to the spread of information as well. However, due to very low levels of trust and resulting sparseness in the network, seeds selected based on eigenvector and closeness centrality obstruct diffusion. Several studies (Hui et al. 2010; Hinz et al. 2011; Hussain et al. 2013; Cadger et al. 2016) have confirmed that degree centrality performs well as a seed selection criterion for rapid and widespread information dissemination. Households with great degree centrality have the largest number of direct connections and therefore a relatively high level of immediate influence on others (Delre et al. 2010; Barbuto, Lopolito & Santeramo 2019). In addition, degree centrality poses a simple and cost-effective method for seed selection, which does not require census data (Erlandsson, Bródka & Borg 2017; Borgatti, Everett & Johnson 2018). Thus, choosing the most popular farmers, farmers with the most connections, offers high potential for improving the dissemination of information.
The finding that betweenness-based seeds achieve quick and wide reach is in line with Goldenberg et al. (2009), Hinz et al. (2011), and Cadger et al. (2016). In sparse networks, information can get trapped because it needs to be passed on through more actors to reach other communities in the network (Delre et al. 2010). Thus, targeting farmers who function as bridges and connect villages or friendship groups is important for the diffusion success, particularly in sparse networks.
The fact that seeds selected on the basis of eigenvector centrality lead to poor diffusion is rather surprising as it is in contrast to studies such as Banerjee et al. (2013). However, eigenvector centrality relates to the centrality of the household’s links rather than to the household itself (Chen, van der Lans & Phan 2017; Borgatti, Everett & Johnson 2018). According to Barbuto, Lopolito & Santeramo (2019), directly exercised influence has a stronger impact on diffusion than indirect influence. Besides, Borgatti, Everett & Johnson (2018) argued that eigenvector centrality is not well defined in disconnected networks. Overall, while it may be advantageous for some applications to address farmers with high eigenvector centrality, in the context of our study area this leads to poor diffusion success.
In line with Mochalova & Nanopoulos (2013), closeness-based selection causes low average reach. As closeness centrality represents the distance from an actor to all other actors in the network but does not focalize the direct influence, effects of closeness-based seeds are more likely to be visible in the long run (Muller & Peres 2019). Closeness-based seed selection indeed induces a relatively long take-off phase of the diffusion process, and in some simulation runs the diffusion is not even fully executed at the end of the year. Consequently, closeness-based seed selection calls for critical consideration and is not advisable in this case study for dissemination of information related to agricultural innovations.
Hierarchy-based seed selection causes reach that does not significantly differ compared with betweenness-based, degree-based, or random seed selection despite the slightly lower number of seeds in this scenario. Thus, informing village heads is cost-effective due to the smaller number of required seeds and gains particular appeal if budget restrictions exist. Furthermore, village heads are easy to identify, and informing them is not only convenient to implement, but also meets social norms. Receiving information from village heads directly might be perceived and accepted differently than knowledge transmitted by other community members (Muller & Peres 2019). However, higher ranks can increase social distance, which reduces the probability of information exchange (Beaman & Dillon 2018; Shikuku 2019). Additionally, informing the village heads first might reinforce existing hierarchical structures and favour elites that may capture early rents from the innovation. Which effect predominates is subject to further research. Overall, the choice of village heads as seeds is an attractive option due to the resulting high reach, but might cause unforeseen complications in the practical implementation.
All these results are compared with the scenario where seeds are randomly selected. Random selection of seeds achieves comparatively good results because information can be seeded in the main as well as smaller components and consequently be disseminated simultaneously to several parts of the network. Since random distribution in the community reduces the risk of knowledge being trapped, it can cause a large network reach. In contrast, centrality-based seeding strategies might lead to seed redundancy due to the choice of farmers who are part of the connected core in the network’s big component (Akbarpour Malladi, & Saberi 2018; Beaman & Dillon 2018). Furthermore, Isaac et al. (2007) found intensified information exchange between farmers in the core of the network compared with peripheral farmers. However, the high reach of random seeds relates to the mean value of the simulations, but can vary considerably in individual simulation runs as the high standard deviation indicates. Therefore, random seed selection achieves comparatively good results on average, but should be treated carefully in practice due to high uncertainty.
Scenario 2 reveals that an increase in seed set sizes generally improves information spread, but the marginal effects of additional seeds decline. Whereas larger seed set sizes accelerate the diffusion process and therefore reach saturation levels earlier, smaller seed set sizes lead to an initially slower information exchange. These results are in line with expectations and the literature (Bampo et al. 2008; Akbarpour, Malladi & Saberi 2018). Bampo et al. (2008) explained that the probability of information transmission between households and thus speed in early time steps increase with larger seed set sizes. If the number of seeds is high, the maximum spread is reached more promptly. Because a saturation level of the diffusion is attained eventually, increasing large quantities of seeds after a certain point does not further enhance reach, and the marginal value of additional seeds decreases (Bampo et al. 2008; Aral, Muchnik & Sundarajan 2013; Akbarpour, Malladi & Saberi 2018). Overall, policy-makers can improve the reach by increasing the seed set size. Especially if information is time-critical, expanding the seed set is efficient to enhance reach in the short run. Enlarging seed sets also increases reach in the long run if the seed set size is small. However, for larger seed set sizes other interventions than increasing the number of seeds can be more efficient to improve diffusion as a saturation level will be eventually reached.
Scenario 3 shows that interaction effects between seed set size and selection criteria exist. In the case of random, degree-, and betweenness-based seed selection, larger seed set sizes result in wider reach with decreasing marginal effects of additional seeds, whereas the performance of closeness-and eigenvector-based seed selection depends on the size of the seed set. Targeting a large number of farmers with high degree centrality achieves best results in terms of speed and reach. Overall, the simulation of the interaction effects highlights that both seed selection criterion and seed size should be considered simultaneously in the design of innovation interventions.
Our simulations as well as the growing use of ABMs in policy in general demonstrate that ABMs can be a beneficial tool to support policy and the design of interventions. By conducting experiments in a virtual setting, several policy options and possible future development paths can be explored ex-ante at relatively low costs (Ahrweiler 2017; Gilbert et al. 2018). Accordingly, the ABM implemented in this study can allow the user to investigate the performance of a range of different seed sets before deciding which strategy to employ for the actual project implementation. ABMs offer some advantages over other modelling techniques as they do not impose assumptions on stationary, linearity, and homogeneity. Furthermore, they account for individual decision making and interaction between heterogenous agents and can cover a range of potential system states, including outcomes that might be of low probability, path-dependent, or emergent (Dechesne, Ghorbani and Yorke-Smith 2015; Polhill et al. 2019). In this way, ABMs enable the user to relate the system’s dynamics and structure to the properties and behaviours of individual agents (Ahrweiler 2017). Consequently, ABMs contribute to the understanding of system dynamics and development paths that can be expected under different policy scenarios (Ahrweiler 2017; Gilbert et al. 2018). Our ABM shows how the transfer of information to a set of individuals and the resulting information exchange on the farmer level shape the process of knowledge dissemination at the community level.
However, ABMs do not provide any prediction into the future. Rather, they help to identify possible and probable paths and undesirable or unintended consequences (Ahrweiler 2017; Polhill et al. 2019). For instance, our analysis showed that degree-based seeds perform well in all scenarios, but choosing seeds based on eigenvector centrality leads to undesirable diffusion results and should therefore be avoided. While our ABM could contribute to understanding the role of different actors for knowledge diffusion processes, our insights can inform development practitioners in designing innovation interventions.
This said, our study also has several limitations. Whereas our study focuses on information as a prerequisite for adoption, other factors also play a role in adoption decisions of farmers. According to the literature, these include financial constraints (Krejci & Beamon 2015; Nguyen et al. 2019; Perello-Moragues, Noriega & Poch 2019), attractiveness of alternative choices (Xu et al. 2020), compatibility of the innovation with existing livelihood strategies (Gassner et al. 2019) and characteristics of the innovation itself (Okello et al. 2019; Caffaro et al. 2020). In addition, adoption decisions are influenced by socio-economic characteristics of farmers such as education and land size (Nguyen et al. 2017; Beyene et al. 2018), biophysical factors such as irrigation (Olum et al. 2020), as well as psychological and behavioural factors such as risk attitude (Kahneman & Tversky 1979; Chavas & Nauges 2020) and time preference (Lawrance 1991; Liebenehm & Waibel 2014; Llewellyn & Brown 2020). Our study only looked at information that is transferred by word-of-mouth communication and thus does not consider dissemination via other information channels, such as observation or media (Perello-Moragues, Noriega & Poch 2019; Xu et al. 2020). Furthermore, networks may evolve over time as a result of knowledge transfer and interaction between farmers (Isaac et al. 2007; Wood et al. 2014). However, in our study, the network was assumed to be static. Our analysis focussed on information of low complexity that is transferable between farmers via single encounters as simple contagion. This implies that the validity of our results is restricted to this type of information.
At the same time, these limitations can stimulate further research. The role of alternative information sources may be considered for further investigation. Furthermore, a systematic testing of additional criteria such as diffusion centrality and combination of criteria, strategies that consider individual characteristics like persuasiveness, and, in this context, the role of village heads and gender are worth of note (Hinz et al. 2011; Banerjee et al. 2013). Future research should also assess inefficiencies that may occur in the transfer process, such as declining values of information, lack of incentives to pass information on or individual resistance to knowledge transfer due to competitiveness between farmers. Therefore, it would be interesting to evaluate ways to enhance not only seed selection, but also the transfer process itself by testing different interventions and comparing their cost-effectiveness. The simultaneous consideration of several factors as prerequisites for adoption could increase our understanding of adoption decisions. In general, more empirical research is needed on comprehensive and system-level approaches to improve information dissemination (Aral, Muchnik & Sundarajan 2013; Barbuto, Lopolito & Santeramo 2019).
Model Documentation
The model has been implemented in NetLogo (Wilensky 1999). The code is available at: https://www.comses.net/codebase-release/42f7c896-7f0a-4ccd-afe8-a9dda594eff8/.Acknowledgements
We thank the community of Mantapala for their participation and continuous cooperation. We also thank the Zambian local Ministry of Agriculture for their support in the organization of the research activities. This work was supported by the Federal Office for Agriculture and Food (BLE) and funded by the Federal Ministry of Food and Agriculture (BMEL) under grant 2813FSNU11.Appendix
Appendix A: Supplementary Tables and Figures
Closeness-centrality | 0.2435 |
Degree | 3.2857 |
Betweenness-centrality | 1472.4024 |
Eigenvector-centrality | 0.3054 |
Cluster | 0.000 |
Closeness-centrality | 0.2176 |
Degree | 1.800 |
Betweenness-centrality | 470.7631 |
Eigenvector-centrality | 0.3500 |
Cluster | 0.0592 |
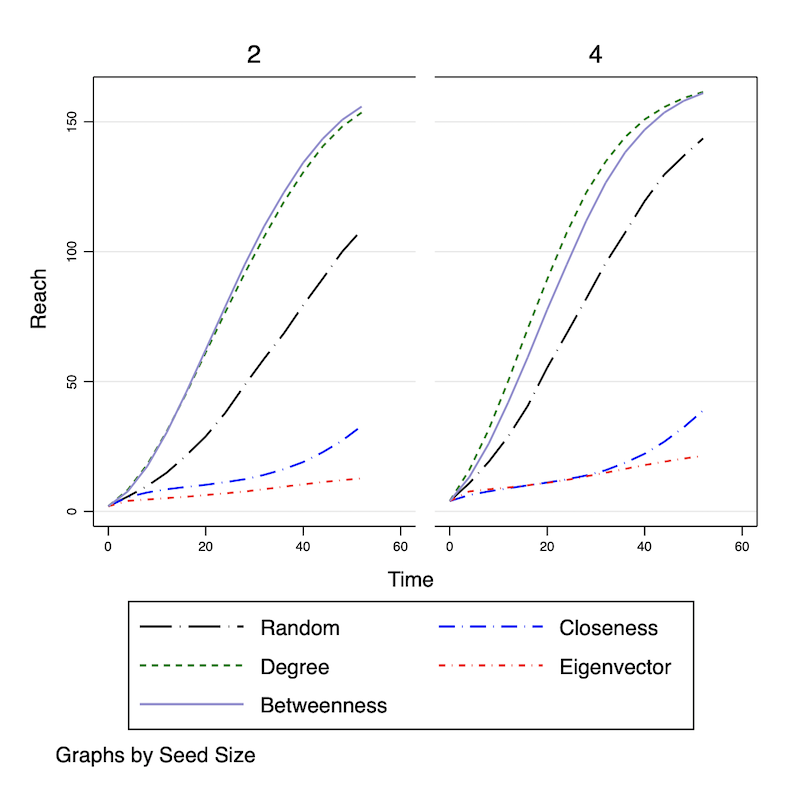
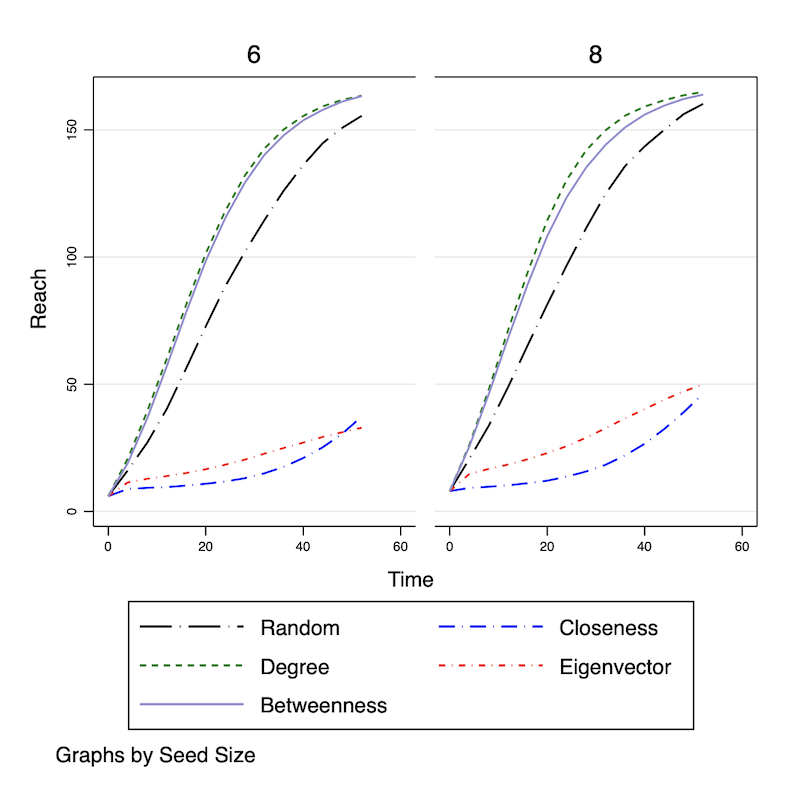
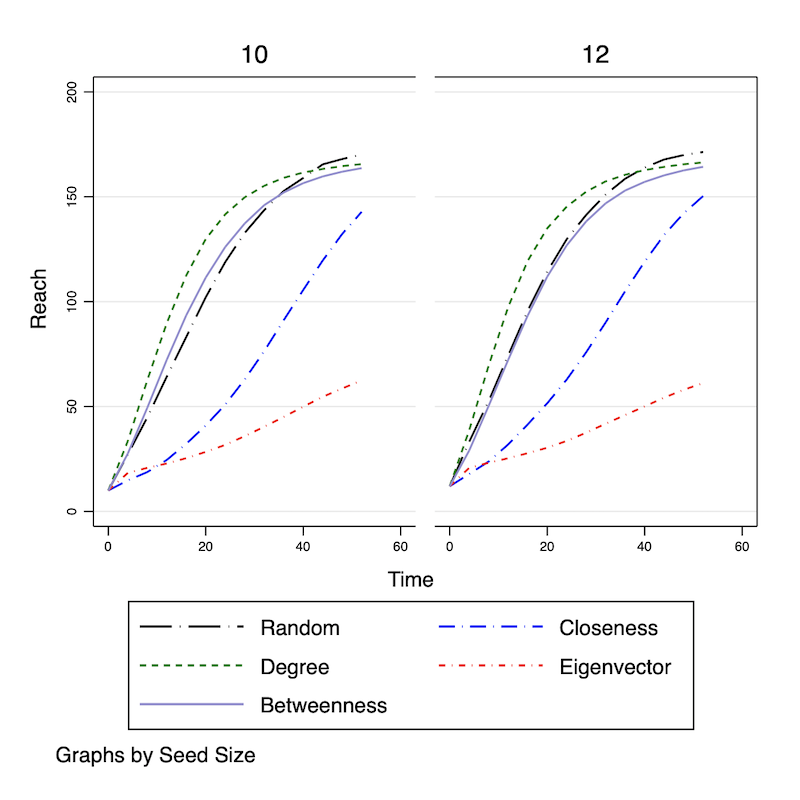
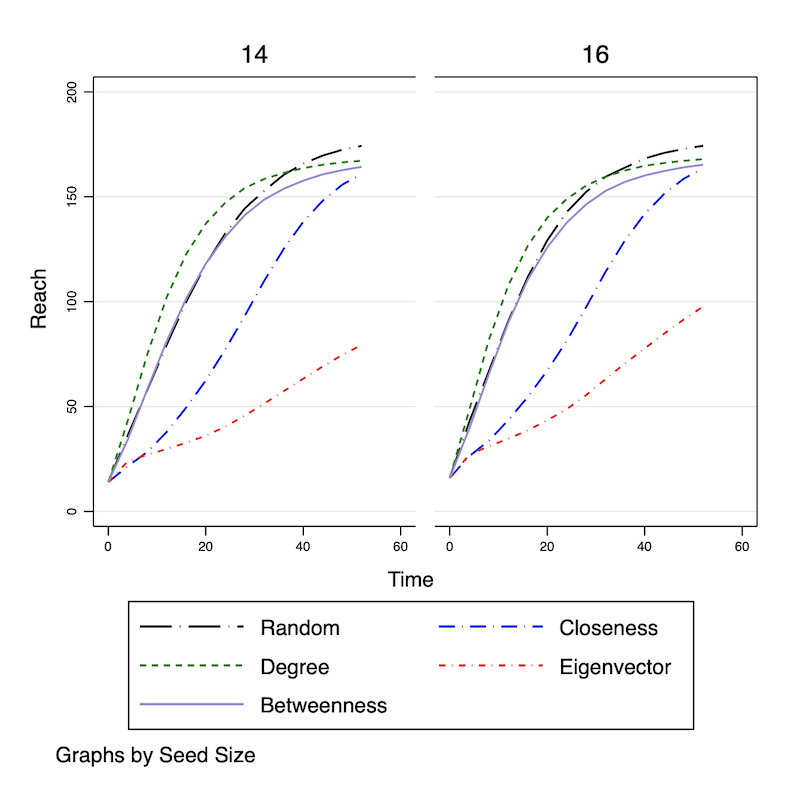
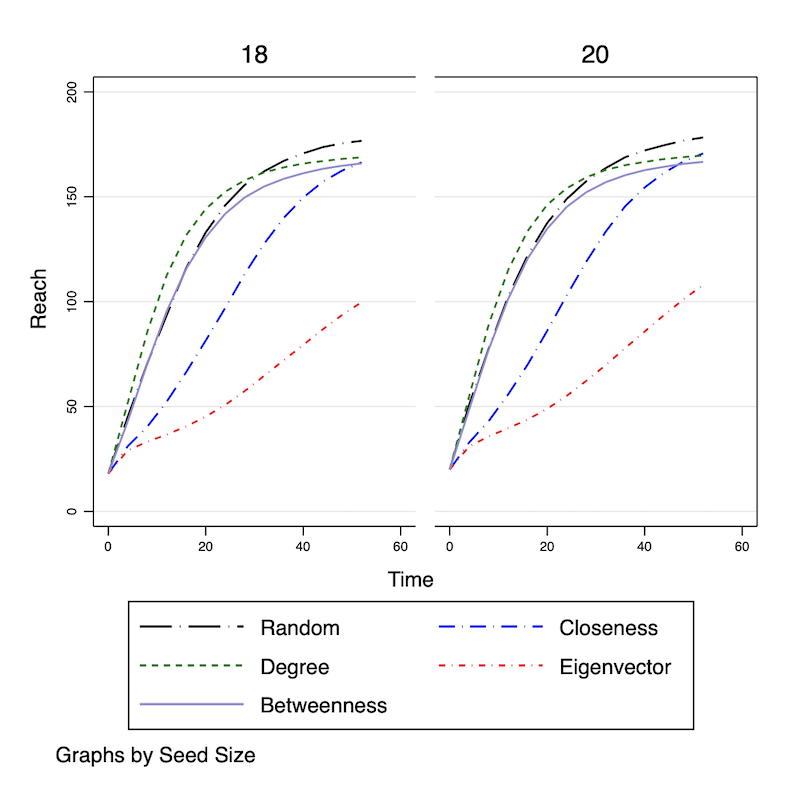
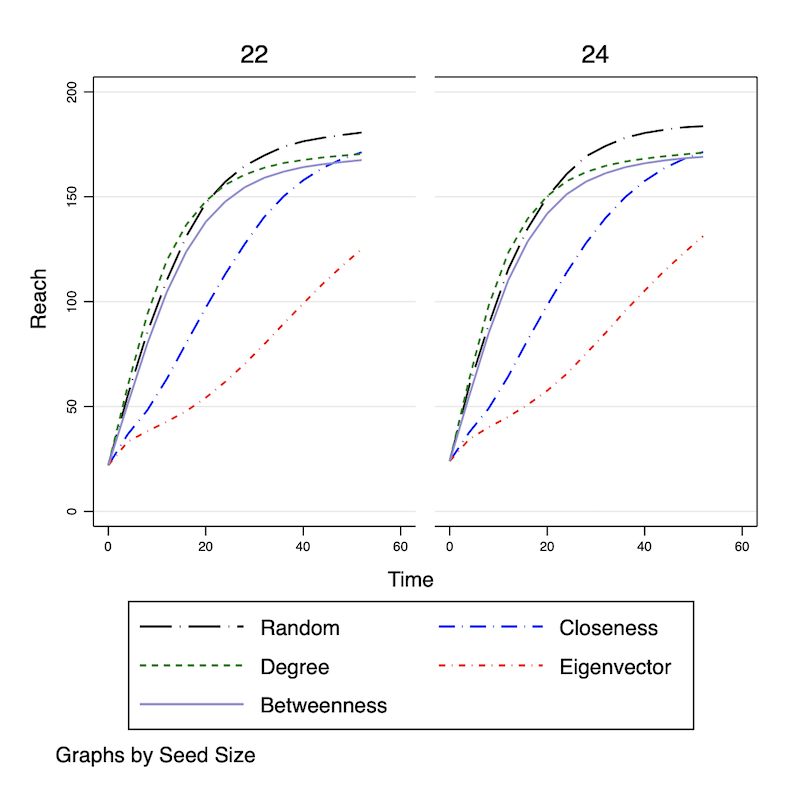
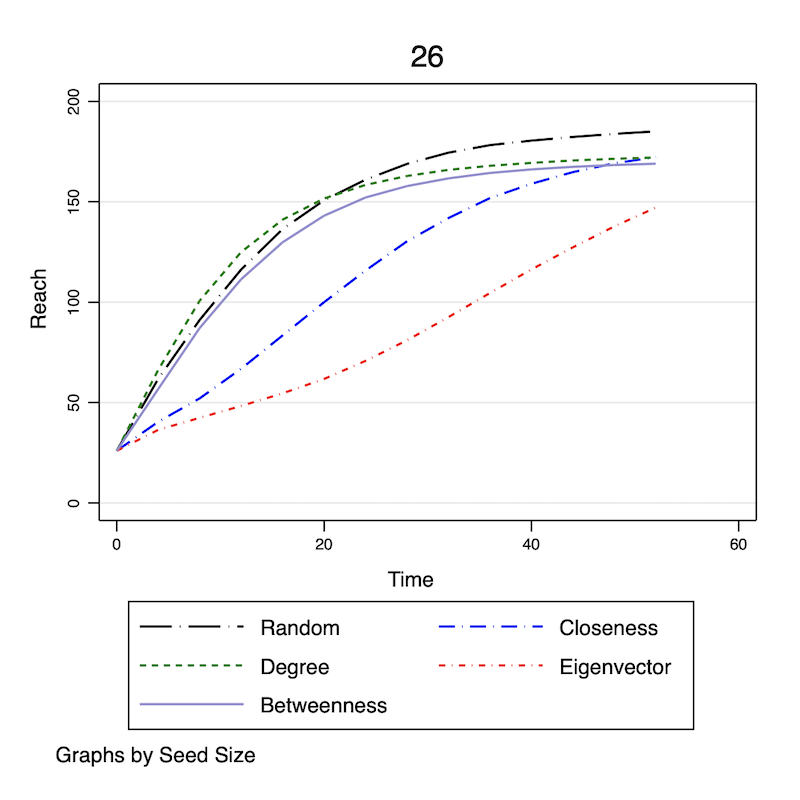
Appendix B: ODD-Protocol
The description of the ABM follows the ODD (Overview, Design concepts, Details) protocol as presented in the subsequent section (Grimm et al. 2006, 2010). The model was implemented using NetLogo 6.0.2.
Overview
Purpose: The purpose of the model is to simulate the information diffusion by word-of-mouth communication between households in rural Zambia. Thereby, the model aims at identifying the set of seeds that enhances information spread the most. It addresses policy-makers, extension officers, and project managers to support them spreading information within a social network effectively when resources limit the direct contact with all farmers.
Entities, state variables, and scales: The acting entities in the model are households represented by their household head. They correspond to the 264 interviewed households in the study area. Households are defined by a variable describing their state of information. For the village heads, a variable indicating their hierarchical status is introduced. GPS data of the households provide input for the model environment, which reproduces the original village structure. Households can be connected via links. The links include variables indicating whether the respective households discuss agricultural matters and how often they exchange agricultural information. One simulation run includes 52 steps and represents a year.
Process overview and scheduling: Each model run starts with the initialization procedure (see initialization). The model proceeds in weekly time steps. During each time step, the households can exchange information as illustrated in Figure 15: The already informed households randomly pick another household to whom they are connected through an information link. If the other household has not received the information yet, the acting household may inform the other household: The more frequently the two households discuss agriculture among each other according to the survey, the higher the likelihood of information transmission. In case of successful knowledge transmission, the newly informed households update their status. At the end of each time step, plots and global variables are updated.
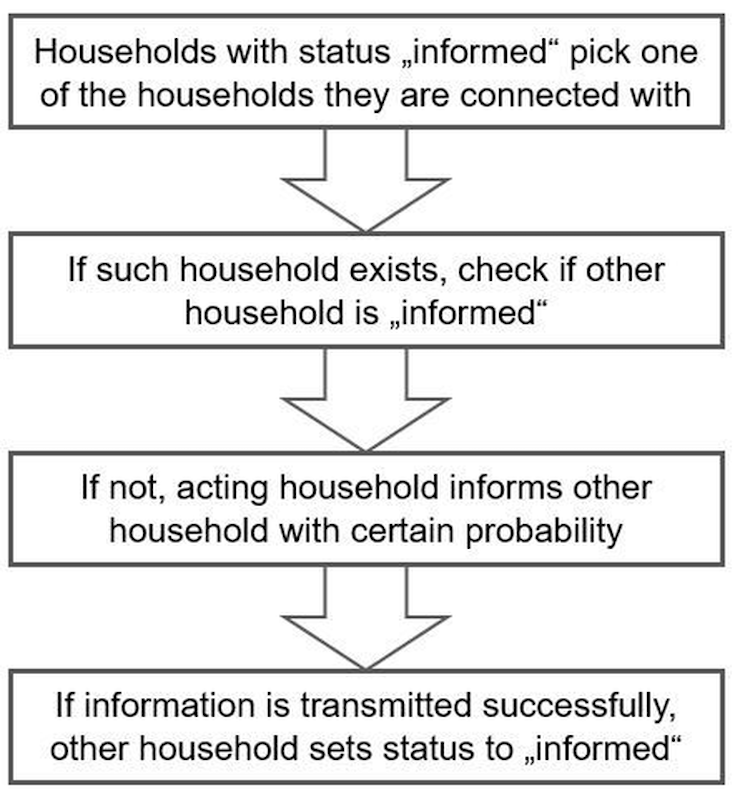
Design concepts
Basic principles: The model employs an empirical approach to simulate information exchange between households. The application of empirically grounded behavioural information based on the household census requires no further assumptions regarding a specific behavioural theory, and the model resembles reality. The information is assumed to be non-rival in consumption and to have low complexity so that one-time contacts between farming households suffice for successful transmission (simple contagion) (Centola 2018).
Emergence: System dynamics emerge from actions on the household level. The individual behaviour of households represented by the household heads in the model replicates the patterns observed in the survey coupled with some randomness. The network itself is static.
Adaptation: Decision rules are implicitly modelled based on the empirical data on information exchange from the census.
Objectives: The households do not follow an explicit objective; they act according to the behavioural data collected.
Sensing: Households know whether they have received the information, to whom they are linked, and with whom they discuss business. They are also aware whether their contacts have obtained the information.
Interaction: Interaction occurs every time step through direct word-of-mouth communication during which households can exchange information.
Stochasticity: Households choosing other households to interact within each time step takes place in a random order. If a household is connected to several households, the choice of a household to exchange information with is random with equal likelihoods. Additionally, the probability of information exchange between two households depends on the frequency of discussion as indicated in the survey, but is also subject to stochasticity. Randomness can also occur during seed selection.
Observation: The model output includes data regarding the reach (total number of households informed) and speed (rate of diffusion, number of households informed monthly). Thereby, reach provides information about final spread; speed provides information about progress over time. The interface presents several graphic outputs that visualize the dynamics of the model.
Details
Initialization: To initialize the model, 264 households (agents), their characteristics (location, hierarchical status) as well as the links and their features describing the exchange of agricultural information are set up matching the survey data. Initially, all households set their status to “uninformed”; only the households selected as seeds set their state to “informed”. Global variables are set to zero.
Input data: A household survey conducted in 2018 in Zambia provides the data used as input. The data includes household and network characteristics. GPS-data of the households spatially locate the households.
Interventions: Three scenarios are conducted to find the most eligible set of seeds for improved information diffusion within a social network.
- The first scenario examines the impact of selection criteria for seeds on the diffusion process. Selecting seeds is based on the following selection criteria i) random ii) hierarchy (village heads) iii) degree centrality iv) closeness centrality v) betweenness centrality and vi) eigenvector centrality. If seeds are chosen according to a centrality measure, isolated households are rejected as seeds. For closeness-centrality, the intra-component closeness of a household is calculated, and thus considers only the distances to the households that are part of the same component, since distance to households in other components is undefined. The closeness centrality of an isolated household is defined to be zero. While comparing these seed selection criteria, the number of seeds is kept constant at eight in the first scenario. This corresponds to approximately 3% of the whole population (Leeuwis 2004; Natcher et al. 2016). Because seven village heads administer the study area, in the case of hierarchy-based seed selection the number of seeds is held constant at seven.
- To assess the effect of the number of seeds on the diffusion process, the number of seeds in the setup varies in the second scenario from two to 26 by increments of two, which corresponds to 1 up to 10% of the whole population (Bampo et al. 2008; Erlandsson, Bródka, & Borg 2017). Thereby, the seed selection is random.
- Scenario 3 investigates the interaction effects between the selection criteria of the seeds and number of seeds by simultaneously changing the seed set size and selection criteria as summarized in Table 8.
Parameter | Function | Options |
Number-entry-points | Indicates the seed set size | From 2 to 26; increment by 2 |
Choosing-mechanism | Indicates seed selection criterion | Random Degree centrality Eigenvector centrality Closeness centrality Betweenness centrality Hierarchy (village heads) |
References
AHRWEILER, P. (2017). Agent-based simulation for science, technology, and innovation policy. Scientometrics, fhtt110(1), 391-415. [doi:10.1007/s11192-016-2105-0]
AKBARPOUR, M., Malladi, S., & Saberi, A. (2018). Just a Few Seeds More: Value of Network Information for Diffusion. Available at SSRN: https://ssrn.com/abstract=3062830. [doi:10.2139/ssrn.3062830]
ANDERSON, J.R., & Feder, G. (2004). Agricultural Extension: Good Intentions and Hard Realities. The World Bank Research Observer, 19(1), 41-60. [doi:10.1093/wbro/lkh013]
ARAL, S., Muchnik, L., & Sundrararajan, A. (2013). Engineering Social Contagions: Optimal Network Seeding in the Presence of Homophily. Network Science, 1(2), 125-153. [doi:10.1017/nws.2013.6]
BAMPO, M., T. Ewing, M. T., Mather, D. R., Stewart, D., & Wallace, M. (2008). The Effects of the Social Structure of Digital Networks on Viral Marketing Performance. Information Systems Research, 19(3), 273-290. [doi:10.1287/isre.1070.0152]
BANDIERA, O., & Rasul, I. (2006). Social Networks and Technology Adoption in Northern Mozambique. The Economic Journal, 116(514), 869-902. [doi:10.1111/j.1468-0297.2006.01115.x]
BANERJEE, A., Chandrasekhar, A. G., Duflo, E., & Jackson, M. O. (2014). Gossip: Identifying Central Individuals in a Social Network. NBER Working Paper no. 20422 Cambridge. [doi:10.3386/w20422]
BANERJEE, A., Chandrasekhar, A. G., Duflo, E., & Jackson, M. O. (2013). The diffusion of microfinance. Science, 341(6144). [doi:10.1126/science.1236498]
BARBUTO, A., Lopolito, A., & Santeramo, F. G. (2019). Improving Diffusion in Agriculture: An Agent-based Model to Find the Predictors for Efficient Early Adopters. Agricultural and Food Economics, 7(1), 1. [doi:10.1186/s40100-019-0121-0]
BEAMAN, L., & Dillon, A. (2018). Diffusion of Agricultural Information within Social Networks: Evidence on Gender Inequalities from Mali. Journal of Development Economics, 133, 147-161. [doi:10.1016/j.jdeveco.2018.01.009]
BEAMAN, L., BenYishay, A., Magruder, J., & Mobarak. A. M. (2018). Can Network Theory based Targeting Increase Technology Adoption. Cowles Foundation Discussion Paper no. 2139; Yale University Economic Growth Center Discussion Paper no. 1062. [doi:10.3386/w24912]
BEYENE, A., Mekonnen, A., Randall, B., & Deribe, D. (2019). Household Level Determinants of Agroforestry Practices Adoption in Rural Ethiopia. Forests, Trees and Livelihoods, 28(3), 194-213. [doi:10.1080/14728028.2019.1620137]
BOGNER, K., Müller, M., & Schlaile, M. P. (2018). Knowledge Diffusion in Formal Networks: The Roles of Degree Distribution and Cognitive Distance. International Journal of Computational Economics and Econometrics, 8(3-4), 388-407. [doi:10.1504/ijcee.2018.10017752]
BORGATTI, S. P., Everett, M. G., & Johnson, J. C. (2018). Analyzing Social Networks. 2nd ed. Los Angeles: Sage.
CADGER, K., Quaicoo, A. K., Dawoe, E., & Isaac, M. E. (2016). Development interventions and agriculture adaptation: a social network analysis of farmer knowledge transfer in Ghana. Agriculture, 6(3), 32. [doi:10.3390/agriculture6030032]
CAFFARO, F., Cremasco, M., Roccato, M., & Cavallo, E. (2020). Drivers of farmers’ intention to adopt technological innovations in Italy: The role of information sources, perceived usefulness, and perceived ease of use. Journal of Rural Studies, 76, 264-271. [doi:10.1016/j.jrurstud.2020.04.028]
CENTOLA, D. (2018). How Behavior Spreads. The Science of Complex Contagions. Princeton, NJ: Princeton University Press.
CENTRAL Statistical Office Zambia. (2016). 2015 Living Conditions Monitoring Survey Report. Lusaka: Zambia. Retrieved from https://www.zamstats.gov.zm/phocadownload/Living_Conditions/2015%20Living%20Conditions%20Monitoring%20Survey%20Report.pdf.
CENTRAL Statistical Office Zambia. (2018). Zambia in Figures 2018. Lusaka: Zambia. Retrieved from: https://www.zamstats.gov.zm/phocadownload/Dissemination/Zambia%20in%20Figure%202018.pdf.
CHAVAS, J. & Nauges, C. (2020). Uncertainty, Learning, and Technology Adoption in Agriculture. Applied Economic Perspectives and Policy, 42(1), 42-53. [doi:10.1002/aepp.13003]
CHEN, X, van der Lans, R., & Phan, T. Q. (2017). Uncovering the Importance of Relationship Characteristics in Social Networks: Implications for Seeding Strategies. Journal of Marketing Research, 54(2), 187-201. [doi:10.1509/jmr.12.0511]
CONLEY, T. & Udry, C. (2001). Social Learning through Networks: The Adoption of New Agricultural Technologies in Ghana. American Journal of Agricultural Economics, 83(3), 668-673. [doi:10.1111/0002-9092.00188]
COWAN, R. & Jonard, N. (2004). Network Structure and the Diffusion of Knowledge. Journal of Economic Dynamics and Control, 28(8), 1557-1575. [doi:10.1016/j.jedc.2003.04.002]
D'ANGELO, G., Severini, L., & Velaj, Y. (2017). Selecting Actors and Buying Links to Maximize the Information Diffusion in a Network. Presented at 42nd International Symposium on Mathematical Foundations of Computer Science, Aalborg, August 21-25. Retrieved from: http://arxiv.org/pdf/1706.06466v1.
DECHESNE, F., Ghorbani, A., & Yorke-Smith, N. (2015). Introduction to the special issue on agent-based modelling for policy engineering. AI & SOCIETY, 30(3), 311-313. [doi:10.1007/s00146-014-0535-x]
DELRE, S. A., Jager, W., & Janssen, M. A. (2007). Diffusion Dynamics in Small-world Networks with Heterogeneous Consumers. Computational and Mathematical Organization Theory, 13(2), 185-202. [doi:10.1007/s10588-006-9007-2]
DELRE, S. A., Jager, W., Bijmolt, T. H., & Janssen, M. A. (2010). Will it spread or not? The effects of social influences and network topology on innovation diffusion. Journal of Product Innovation Management, 27(2), 267-282. [doi:10.1111/j.1540-5885.2010.00714.x]
DIJKXHOORN, Y., Plaisier, C., Verwaart, T., Wagenberg, C. V., & Ruben, R. (2019). Trusted sorghum: simulating interactions in the sorghum value chain in Kenya using games and agent-based modelling. Journal of Development Effectiveness, 11(2), 146-164. [doi:10.1080/19439342.2019.1624596]
ERLANDSSON, F., Bródka, P., & Borg, A. (2017, November). Seed selection for information cascade in multilayer networks. In International Conference on Complex Networks and their Applications (pp. 426-436). Springer, Cham. [doi:10.1007/978-3-319-72150-7_35]
FEDER, G., Just, R. E., & Zilberman, D. (1985). Adoption of Agricultural Innovations in Developing Countries: A Survey. Economic Development and Cultural Change, 33(2), 255-298. [doi:10.1086/451461]
FOSTER, A. D., & Rosenzweig, M. R. (1995). Learning by Doing and Learning from Others: Human Capital and Technical Change in Agriculture. Journal of Political Economy, 103(6), 1176-1209. [doi:10.1086/601447]
GASSNER, A., Harris, D., Mausch, K., Terheggen, A., Lopes, C., Finlayson, R., & Dobie, P. (2019). Poverty eradication and food security through agriculture in Africa: Rethinking objectives and entry points. Outlook on Agriculture, 48(4), 309–315. [doi:10.1177/0030727019888513]
GENIUS, M., Koundouri, P., Nauges, C., & Tzouvelekas, V. (2014). Information Transmission in Irrigation Technology Adoption and Diffusion: Social Learning, Extension Services, and Spatial Effects. American Journal of Agricultural Economics, 96(1), 328-344. [doi:10.1093/ajae/aat054]
GILBERT, N., Ahrweiler, P., Barbrook-Johnson, P., Narasimhan, K., & Wilkinson, H. (2018). Computational Modelling of Public Policy: Reflections on Practice. Journal of Artificial Societies and Social Simulation, 21(1), 14: https://www.jasss.org/21/1/14.html. [doi:10.18564/jasss.3669]
GOLDENBERG, J., Sangman, H., Lehmann, D. R., & Hong, J. W. (2009). The Role of Hubs in the Adoption Process. Journal of Marketing, 73(2), 1-13.
GRANOVETTER, M. (1973). The Strength of Weak Ties. American Journal of Sociology, 78, 1360–1380. [doi:10.1086/225469]
GRONAU, S., Hadersdorfer, J., Nöldeke, B., Petrusjanz, N., Stützel, H., & Winter, E. (2019). Ernährungssicherung im ländlichen Sambia. Ernährung im Fokus – 02 2019 – Zeitschrift für Fach-, Lehr- und Beratungskräfte, 5982. Bundeszentrum für Ernährung. Bundesanstalt für Landwirtschaft und Ernährung.
GRONAU, S., Winter, E., & Grote, U. (2018). Papyrus, forest resources and rural livelihoods: A village computable general equilibrium analysis from northern Zambia. Natural Resources 9 (2018), Nr. 6. [doi:10.4236/nr.2018.96017]
HAMPWAYE, G., Jeppesen, S., & Kragelund, P. (2014). Zambia country background report. Successful African Firms and Institutional Change (SAFIC) Project.
HINZ, O., Skiera, B., Barrot, C., & Becker, J. U. (2011). Seeding Strategies for Viral Marketing: An Empirical Comparison. Journal of Marketing, 75(6), 55-71. [doi:10.1509/jm.10.0088]
HUI, C., Goldberg, M., Magdon-Ismail, M., &. Wallace, W. A. (2010). Simulating the Diffusion of Information: An Agent-Based Modeling Approach. International Journal of Agent Technologies and Systems (IJATS), 2(3), 31-46. [doi:10.4018/jats.2010070103]
HUSSAIN, O., Anwar, A., Saleem, S., & Zaidi, F. (2013). Empirical Analysis of Seed Selection Criterion in Influence Mining for Different Classes of Networks. Paper presented at the 2013 International Conference on Cloud and Green Computing, Karlsruhe, September 30 - October 2. [doi:10.1109/cgc.2013.61]
ISAAC, M., Erickson, B., Quashie-Sam, S., & Timmer, V. (2007). Transfer of Knowledge on Agroforestry Management Practices: the Structure of Farmer Advice Networks. Ecology and Society, 12(2), 32. [doi:10.5751/es-02196-120232]
JANG, J., Ju, X., Ryu, U., & Om, H. (2019). Coevlutionary Characteristics of Knowledge Diffusion and Knowledge Network Structures: A GA-ABM Model. Journal of Artificial Societies and Social Simulation, 22(3), 3: https://www.jasss.org/22/3/3.html. [doi:10.18564/jasss.4037]
KABUNGA, N. S., Dubois, T., & Qaim. M. (2012). Heterogeneous Information Exposure and Technology Adoption: The Case of Tissue Culture Bananas in Kenya. Agricultural Economics, 43(5), 473-486. [doi:10.1111/j.1574-0862.2012.00597.x]
KAHNEMAN, D. & Tversky, A. (1979). Prospect Theory: An Analysis of Decision under Risk. Econometrica, 47, 263-292. [doi:10.2307/1914185]
KEMPE, D., Kleinberg, J., & Tardos, E. (2003, August). Maximizing the spread of influence through a social network. In Proceedings of the ninth ACM SIGKDD international conference on Knowledge discovery and data mining (pp. 137-146). [doi:10.1145/956750.956769]
KHONJE, M., Manda, J., Alene, A. D., & Kassie, M. (2015). Analysis of Adoption and Impacts of Improved Maize Varieties in Eastern Zambia. World Development, 66, 695–706. [doi:10.1016/j.worlddev.2014.09.008]
KOWALSKA-STYCZEN, A., Malarz, K., & Paradowski, K. (2018). Model of Knowledge Transfer Within an Organisation. Journal of Artificial Societies and Social Simulation, 21(2), 3: https://www.jasss.org/21/2/3.html.
KREJCI, C. & Beamon, B. (2015). Impacts of Farmer Coordination Decisions on Food Supply Chain Structure. Journal of Artificial Societies and Social Simulation, 18(2), 19: c. [doi:10.18564/jasss.2727]
LAWRANCE, E. (1991). Poverty and the Rate of Time Preference: Evidence from Panel Data. Journal of Political Economy, 99(1), 54-77. [doi:10.1086/261740]
LEAVY, J. (2015). Measuring Social Networks in Three Zambian Villages. Journal of Development Effectiveness, 7(3), 357-374. [doi:10.1080/19439342.2015.1068831]
LEEUWIS, C. (2004). Communication for Rural Innovation. Rethinking Agricultural Extension. 3rd ed. Oxford, UK: Blackwell Science Ltd.
LIEBENEHM, S. & Waibel, H. (2014). Simultaneous Estimation of Risk and Time Preferences among Small-scale Cattle Farmers in West Africa. American Journal of Agricultural Economics, 96(5), 1420–1438. [doi:10.1093/ajae/aau056]
LLEWELLYN, R. & Brown, B. (2020). Predicting Adoption of Innovations by Farmers: What is Different in Smallholder Agriculture? Applied Economic Perspectives and Policy, 42(1), 100-112. [doi:10.1002/aepp.13012]
LÜ, L., Chen, D., Ren, X., Zhang, Q., Zhang, Y., & Zhou, T. (2016). Vital Actors Identification in Complex Networks. Physics Reports, 650, 1–63. [doi:10.1016/j.physrep.2016.06.007]
MAGNAN, N., J. Spielman, D. J., J. Lybbert, T. J., & Gulati, K. (2015). Leveling with Friends: Social Networks and Indian Farmers' Demand for a Technology with Heterogeneous Benefits. Journal of Development Economics, 116, 223–251. [doi:10.1016/j.jdeveco.2015.05.003]
MANSON, S. M., Jordan, N. R., Nelson, K. C., & Brummel, R. F. (2016). Modeling the effect of social networks on adoption of multifunctional agriculture. Environmental modelling & software, 75, 388-401. [doi:10.1016/j.envsoft.2014.09.015]
MATUSCHKE, I. (2008). Evaluating the Impact of Social Networks in Rural Innovation Systems. IFPRI Discussion Paper 00816, Food and Agriculture Organization of the United Nations.
MATUSCHKE, I., & Qaim, M. (2009). The impact of social networks on hybrid seed adoption in India. Agricultural Economics, 40(5), 493-505. [doi:10.1111/j.1574-0862.2009.00393.x]
MEKONNEN, D. A., Gerber, N., & Matz, J. A. (2018). Gendered Social Networks, Agricultural Innovations, and Farm Productivity in Ethiopia. World Development, 105, 321–335. [doi:10.1016/j.worlddev.2017.04.020]
MOCHALOVA, A., & Nanopoulos, A. (2013). On The Role Of Centrality In Information Diffusion In Social Networks. Paper presented at Proceedings of the 21st European Conference on Information Systems, Utrecht, June 5-8.
MORONE, P., & Taylor, R. (2004a). Knowledge Diffusion Dynamics and Network Properties of Face-to-face Interactions. Journal of Evolutionary Economics, 14(3), 327-351. [doi:10.1007/s00191-004-0211-2]
MORONE, P., & Taylor, R. (2004b). Small World Dynamics and The Process of Knowledge Diffusion: The Case of The Metropolitan Area of Greater Santiago De Chile. Journal of Artificial Societies and Social Simulation, 7(2), 5: https://www.jasss.org/7/2/5.html.
MUANGE, E. N., Schwarze, S., & Qaim, M. (2014). Social Networks and Farmer Exposure to Improved Crop Varieties in Tanzania. Presented at Agri-Food and Rural Innovations for Healthier Societies, Ljubeljana, August 26-29.
MUELLER, M., Bogner, K., Buchmann, T., & Kudic, M. (2017). The Effect of Structural Disparities on Knowledge Diffusion in Networks: An Agent-based Simulation Model. Journal of Economic Interaction and Coordination, 12(3), 613-634. [doi:10.1007/s11403-016-0178-8]
MULLER, E., & Peres, R. (2019). The Effect of Social Networks Structure on Innovation Performance: A Review and Directions for Research. International Journal of Research in Marketing, 36(1), 3-19. [doi:10.1016/j.ijresmar.2018.05.003]
NATCHER, (2016). Knowledge diffusion and the adoption of fertilizer microdosing in Northwest Benin. Sustainable Agriculture Research, 5(526-2016-37807). [doi:10.5539/sar.v5n3p1]
NGUYEN, H., Chiong, R., Chica, M., Middleton, R., & Pham, D. (2019). Contract Farming in the Mekong Delta’s Rice Supply Chain: Insights from an Agent-Based Modeling Study. Journal of Artificial Societies and Social Simulation, 22(3), 1: c. [doi:10.18564/jasss.4008]
NGUYEN, T., Nguyen, L., Lippe, R., & Grote, U. (2017). Determinants of Farmers’ Land Use Decision-Making: Comparative Evidence From Thailand and Vietnam. World Development, 89, 199-213. [doi:10.1016/j.worlddev.2016.08.010]
OKELLO, J., Zhou, Y., Barker, I., & Schulte-Geldermann, E. (2019). Motivations and Mental Models Associated with Smallholder Farmers’ Adoption of Improved Agricultural Technology: Evidence from Use of Quality Seed Potato in Kenya. The European Journal of Development Research, 31(2), 271-292. [doi:10.1057/s41287-018-0152-5]
OLUM, S., Gellynck, X., Juvinal, J. Ongeng, D., & De Steur, H. (2019). Farmers’ adoption of agricultural innovations: A systematic review on willingness to pay studies. Outlook on Agriculture, 0030727019879453. [doi:10.1177/0030727019879453]
PERELLO-MORAGUES, A., Noriega, P., & Poch, M. (2019). Modelling Contingent Technology Adoption in Farming Irrigation Communities. Journal of Artificial Societies and Social Simulation, 22(4), 1: c. [doi:10.18564/jasss.4100]
POLHILL, J., Ge, J., Hare, M., Matthews, K., Gimona, A., Salt, D., & Yeluripati, J. (2019). Crossing the chasm: a ‘tube-map’ for agent-based social simulation of policy scenarios in spatially-distributed systems. GeoInformatica, 23(2), 169-199. [doi:10.1007/s10707-018-00340-z]
PRATIWI, A., & Suzuki, A. (2017). Effects of Farmers’ Social Networks on Knowledge Acquisition: Lessons from Agricultural Training in Rural Indonesia. Journal of Economic Structures, 6(1), 8. [doi:10.1186/s40008-017-0069-8]
QIAO, T., Shan, W., Zhang, M., & Liu, C. (2019). How to Facilitate Knowledge Diffusion in Complex Networks: The Roles of Network Structure, Knowledge Role Distribution and Selection Rule. International Journal of Information Management, 47, 152–167. [doi:10.1016/j.ijinfomgt.2019.01.016]
RINK, A., & Wong-Grünwald, R. (2017). How Effective Are HIV Behaviour Change Interventions? Experimental Evidence from Zimbabwe. Journal of Development Effectiveness, 9(3), 361-388. [doi:10.1080/19439342.2017.1327880]
ROGERS, E. M. (1983). Diffusion of Innovations. 3rd ed. New York: Free Press; Collier Macmillan.
SAINT Ville, A. S., Hickey, G. H., Locher, U., & Philip, L. E. (2016). Exploring the Role of Social Capital in Influencing Knowledge Flows and Innovation in Smallholder Farming Communities in the Caribbean. Food Security, 8(3), 535-549. [doi:10.1007/s12571-016-0581-y]
SCHELLER, F., Johanning, S., & Bruckner, T. (2018). A Review of Designing Empirically Grounded Agent-based Models of Innovation Diffusion: Development Process Conceptual Foundation and Research Agenda. Contributions of the Institute for Infrastructure and Resources Management 01/2019, University of Leipzig, Institute for Infrastructure and Resources Management.
SHIKUKU, K. M. (2019). Information Exchange Links, Knowledge Exposure, and Adoption of Agricultural Technologies in Northern Uganda. World Development, 115, 94–106. [doi:10.1016/j.worlddev.2018.11.012]
SONGSERMSAWAS, T., Baylis, K., Chhatre, A., & Michelson, H. (2016). Can Peers Improve Agricultural Revenue? World Development, 83, 163–178. [doi:10.1016/j.worlddev.2016.01.023]
VALENTE, T. W., & Davis, R. L. (1999). Accelerating the Diffusion of Innovations Using Opinion Leaders. Annals of the American Academy of Political and Social Science, 566(1), 55-67. [doi:10.1177/000271629956600105]
VAN den Broeck, K. & Dercon, S. (2011). Information Flows and Social Externalities in a Tanzanian Banana Growing village. The Journal of Development Studies, 47(2), 231-252. [doi:10.1080/00220381003599360]
VASILAKY, K. N., & Leonard, K. L. (2018). As Good as the Networks They Keep? Improving Outcomes through Weak Ties in Rural Uganda. Economic Development and Cultural Change, 66(4), 755-792. [doi:10.1086/697430]
WANG, Y., Zhang, B., Vasilakos, A. V., & Ma, J. (2014). A Heuristic Scheme of Initial Seeds Selection for Diffusion Maximization in Social Networks. Paper presented at International Conference on Intelligent Computing, Taiyuan, August 3-6. [doi:10.1007/978-3-319-09333-8_17]
WATTS, D. J., & Strogatz, S. H. (1998). Collective Dynamics of 'Small-world' Networks. Nature, 393(6684), 440-442. [doi:10.1038/30918]
WOOD, B., Blair, H., Gray, D., Kemp, P., Kenyon, P., Morris, S., & Sewell, A. (2014) Agricultural Science in the Wild: A Social Network Analysis of Farmer Knowledge Exchange. PLoS ONE, 9(8), e105203. [doi:10.1371/journal.pone.0105203]
XIONG, H., Payne, D., & Kinsella, S. (2018). Identifying Mechanisms Underlying Peer Effects on Multiple Networks. Journal of Artificial Societies and Social Simulation, 21(4), 6: https://www.jasss.org/21/4/6.html. [doi:10.18564/jasss.3797]
XU, Q., Huet, S., Perret, E., & Deffuant, G. (2020). Do Farm Characteristics or Social Dynamics Explain the Conversion of Dairy Farmers to Organic Farming? An Agent-Based Model of Dairy Farming in 27 French Cantons. Journal of Artificial Societies and Social Simulation 23(2), 4: https://www.jasss.org/23/2/4.html. [doi:10.18564/jasss.4204]
ZHAO, J., Wu, J. & Xu, K. (2010). Weak ties: Subtle role of information diffusion in online social networks. Physical Review E, 82, 016105. [doi:10.1103/PhysRevE.82.016105]