Introduction
How should subordinates be involved in task allocation? When all participants are considered better decisions can be made and subordinates could appreciate being part of any collective decisions. However, involving more participants in decisions can take longer and possibly introduce shortsightedness and global incoherence due to integrating individuals’ viewpoints with limited contextual overview.
Considering the influence of culture on organizations is relevant for making such decisions. For instance, the "power-distance" indicator (Hofstede et al. 2010a) is strictly related to this context. In high power-distance organizations, it is generally assumed that leaders manage task-allocation and subordinates to wait for orders. In low power-distance organizations, this process tends to be more democratic (e.g., subordinates actively request and reject tasks). Applying culturally inadequate allocation mechanisms often leads to profound organizational problems, as well exemplified by Hofstede et al. (2010a) (e.g., subordinates are required to pick tasks on their own, but they passively wait to be given tasks).
In spite of the availability of new theories, predicting the consequences of cultural influence on decisions and societies remains very difficult. This is due to numerous intricacies introduced by cultural influences at all levels, which are often hard to foresee. Available theories provide extensive correlations between culture, micro-level individual decisions, and macro-level collective outcomes. However, as illustrated in Figure 1, theories of culture do not explain the micro-to-macro link, i.e., how and why correlations between culture and cultural phenomena are related to correlations between culture and individual decisions.
This study aims to cover this gap, by exploring how culture influences interactions. Interactions offer an intermediate level between individual decisions and collective phenomena. The influence of culture on interactions can be more easily explained based on the influence on individual decisions, and the influence of culture on interactions can be used to more easily explain the influence of culture on collective outcomes. More specifically, this study considers the influence of culture on the emergence of 1) coherent, 2) recurrent, and 3) generic interaction patterns, and how these interaction patterns impact collective outcomes. Furthermore, we explore the relations between the environment and visibility of cultural influences, as a side-objective of our research. Towards longer-term considerations, this study aims to introduce further elements to answer the general question: how does the known influence of culture on collective outcomes come from the known influence of culture on individual decisions?
A theoretical understanding of this micro-to-macro link is relevant for supporting decisions, such as setting new policies, adapting rules, and reaching desired collective outcomes. When we consider possible simulations, under-standing this link facilitates the design of accurate situation-specific models, to be used for instance for building participatory simulations as proposed by D’Aquino et al. (2003), and studying “what if” scenarios for a wide range of topics (e.g. water management, refugee camp management).
Investigating such micro-to-macro phenomena is particularly challenging given classical social science methodologies. This investigation implies finding and studying comparable societies (i.e., societies with different cultures but facing similar situations), studying their individual-to-collective relations, and then comparing these relations across societies depending on their culture (e.g., as carried out by D’Iribarne 1989; Haaland & Balo 2017; Schramm-Nielsen 2001). The first challenge lies in finding such comparable societies, which is challenging as different societies tend to evolve into different social ecosystems (e.g., political context, regulations). The second challenge lies in collecting the adequate data. In spite of recent technical progress for acquiring extensive data (e.g., Internet of Things, mailing), acquiring relevant data still requires costly human intervention to determine which data to collect and, often, for performing the collection (e.g., interviews, setting up questionnaires). These challenges come on top of non-technical data collection issues, caused for instance by ethical or competition restrictions. Therefore, most of available results tend to come at a high cost while only providing ad hoc insight into the emerging phenomenon.
This study relies on Generative Social Sciences (GSSs), introduced by Epstein (1999) and re-discussed by Conte & Paolucci (2014), as a core methodological approach for efficiently exploring such micro-to-macro links. GSSs methodology consists in building realistic simulation models of individual decisions, such that, when applying these decisions in an environment that matches a real-world social context, the simulation replicates real-world collective-level properties. Such a computational model is particularly relevant for providing candidate explanations for elaborating and comparing social-science theories for explaining the causes of the emerging phenomenon. The GSSs methodology offers a very convenient playground for raising and testing hypotheses, which adds a strong complement to the classic array of social-science methods (e.g., polls, surveys). Simulations can be manipulated and are stable and explorable. They make it possible to generate situations with controlled differences (e.g., changing one aspect at a time), dampen out-of-context noise (e.g., public events, the social context), and probe the system more deeply (e.g., inspect individual mindsets and decisions, re-play interactions). These operations are much more difficult to perform with classical data-acquisition methodologies. GSSs methodology has been applied in several studies. Sugarscape (Epstein & Axtell 1996) studied the emergence of collective phenomena related to genetics, demography, trade, wealth, and culture based on micro-level influences in an abstract society. Dean et al. (2000) modelled a detailed demographic evolution of the Anasazi society to explain its collapse. More generally, while this methodology is insufficiently exploited, as argued by Conte & Paolucci (2014), GSSs are particularly relevant to study micro-to-macro phenomena (e.g., wealth distribution, demographic evolution), systems without equilibria, and phenomena that are difficult to approximate by equations, particularly in interdisciplinary contexts.
This study uses GSSs to explain the micro-macro influence of culture, i.e. explaining how the influence of culture on collective outcomes results from the influence of culture on individual decisions. The theory presented by this study aims at going beyond the (independent) correlations provided by Hofstede et al. (2010a) that relate culture and individual decisions on one hand, and culture and collective outcomes on the other. Our theory aims at explaining how the cultural influence at an individual level causes (or leads to the emergence of) the cultural influence on collective outcomes. Since the GSSs approach requires a realistic simulation model, we focused on the cultural influence on individual decisions and collective outcomes in the context of business organizations, from individual, psychological and cultural influences to collective performance. The psychological influence of culture is integrated via agent decision models containing culturally-sensitive value systems, based on Schwartz values (Miceli & Castelfranchi 1989). The influence of culture on value systems is defined by relying on Hofstede’s cultural dimensions (Hofstede et al. 2010a). These value systems influence agent decisions, actions, interactions, and the aggregated collective performance. This business organization context has been selected due to (1) the extensive amount of broadly-validated available literature that covers it, and (2) the semi-formal context it offers (e.g., organizational structure), detailed metrics (e.g. performance), which all provide a straight-forward modelling step. The simulation model replicates the main micro and macro-level features of culture as depicted by theories (e.g., known influence of culture on individual decisions and collective performance, multicultural misunderstandings). Based on this model, this paper proposes a theory to explain how the known influence of culture on individual decisions entails collective cultural phenomena.
The rest of this article is structured as follows. First, available theories and simulation models of the influence of culture on individual decisions and collective outcomes are introduced. Then, our simulation model is explained through a general overview and a more detailed presentation. Next, the model is empirically validated. Finally, a theory based on this model is proposed for explaining the micro-to-macro influence of culture before a conclusion.
Theoretical Framework
Culture is a complex and fuzzy topic that has received extensive attention (see the definitions proposed by Kroeber & Kluckhohn 1952). In spite of the weight of this research, the concept of culture remains difficult to grasp. The influence of culture is visible in many places: in what people say, what they think, how they behave, in music, in architecture, in societies etc. Specific manifestations of culture can be easily acknowledged when facing differences (e.g., different behaviours or building architectures). However, determining whether a given occurrence is cultural or not (e.g., personality, coincidence, environmental specificity) is difficult and error-prone. This section introduces available core concepts while acknowledging that the field has not yet reached a consensus on these concepts.
Due to the amount of available theories on culture (Kroeber & Kluckhohn 1952), which have introduced diverging scopes, assumptions, observations, and conclusions, focusing on a limited theoretical background is critical for coherence. Here, this article focuses on the theory of Hofstede et al. (2010a), which defines culture as “the collective programming of the mind that distinguishes the members of one group or category of people from others”. This definition highlights the intrinsic psychological origin of culture: e.g., the influence of culture is embedded within the minds of individuals. This definition also highlights the social nature of culture: the psychological influence is shared within groups and delimits them. Although focusing on a single theory may reduce the strength of the model, the theory of Hofstede et al. (2010a) introduces three major benefits that make it highly relevant as a standalone theory: first this theory is comprehensive, introducing several tightly-connected core concepts, covering the influence of culture on both individual decisions and collective outcomes, something which is very practical for building and validating simulation models; second, this theory is very robust, as it has received an extensive amount of attention and empirical validation; and, third it tightly relates to many other cultural studies and theories (e.g., a 20-pages long reference section, with references that are tightly connected with the main theory). The theory of Hofstede et al. (2010a) also has the highest citation count in the Social Science Index (Nakata 2009). Obviously, we acknowledge that while this mono-theory approach is sound for establishing a first model of the influence of culture on the emergence of individual to collective dynamics, the use of additional theories would further strengthen the validity of subsequent models.
Culturally-sensitive aspects
Culture is known to influence a very wide range of aspects: language, architecture, dressing, institutions etc. Given the goal of this paper, this section focuses on two culturally-sensitive aspects: individual decisions and collective outcomes.
Culture is acknowledged to be a psychological influence, which directly impacts individual decisions and behaviors. Hofstede et al. (2010a) particularly mention two culturally-sensitive cognitive aspects: practices and values. Practices constitute “standard behaviours” that are captured in three categories: symbols, heroes (e.g. important people to follow), and rituals (e.g. rules, ceremonies). Practices constitute the most visible side of the influence of culture on decisions. Practices encompass visible habits, such as use of language, eating, greeting, walking speed or clothing. Values (e.g., honesty, creativity, cleanliness, efficiency) represent core and abstract drives to indicate what an individual generally considers as being “good”. Cultures tend to be internally coherent: values and practices tend to match each other. For example, if respecting the social order is a cultural value, then chances are high that this culture also conveys practices for showing respect to higher-status people (e.g., for addressing superiors). This coherence tends to be shared within the community sharing this culture (e.g., individuals belonging to the same community comply with similar rules). Culture evolves over time, but major changes in a culture are relatively slow (over a scale of decades).
Through its influence on individual decisions, culture influences numerous collective phenomena, such as social welfare, law systems, emergence of gender inequalities, the timeliness of meetings, the amount of reporting or business performance. Culture is tightly related to the constitution of communities. Social structures (e.g., laws, institutions) tend to be coherent with the values of practices supported by the society’s culture (e.g., laws of equality vs. laws of equity).
Values
Values are considered to be individual decision drivers (Schwartz 2006a) (Figure 2). Values influence numerous aspects of decisions, as discussed by Miceli & Castelfranchi (1989). At the core, values indicate criteria that individuals assume to be “good”. Pursuing these criteria is generally beneficial for one’s welfare (e.g. being honest generally leads to desirable outcomes). The influence of values tends to be very generic: this influence is (relatively) context-free, it applies in many situations and influences numerous decision processes. By being very generic and influential, values introduce regularities and overall coherence within decisions.
Values are incorporated in value systems (Schwartz 2006a). Individuals tend to have multiple values altogether (i.e., both timeliness and obedience). This multiplicity strengthens decisions and covers a wider range of situations. However, values sometimes contradict each other (e.g., obeying an order may lead to delays). Value systems introduce a notion of relative importance between values for solving dilemmas. Culture influences which values are (assumed to be) more important (e.g. being on time is more important than strictly complying to orders in certain cultures, or the other way around in different cultures).
As a source of concrete values, this article relies on the collection of key values that individuals consider as being important as described by Schwartz (1999), and thus referred to as Schwartz values. Even if these values are acknowledged to be universal, culture influences the relative importance given to these values. This theory has received a lot of attention and has been strongly empirically validated (Schwartz 1999, 2006a, 2006b, 2006c).
Cultural dimensions
Certain theories aim at depicting general patterns of culture influence on decisions and societies (e.g., showing correlations between a high importance given to the value of “obedience” and to symbols for formalizing power status) (Hampden-Turner & Trompenaars 1993; Hofstede et al. 2010a; Schwartz 1999). Hofstede’s theory of culture organizes these patterns along “Cultural Dimensions” (CD) (Hofstede et al. 2010a). Each of Hofstede’s Cultural Dimensions (HCD) describes a generic abstract type of cultural influence (e.g., culture influences the importance given to power status). This influence is conceptually and empirically related to many concrete manifestations (e.g., cultural importance given to power status is related to numerous manifestations, such as stronger compliance to leaders’ orders, leader-based organizational structures and specific ways of addressing superiors).
HCDs are measured by using a score obtained through polls conducted at a national level (e.g., in France, power status is culturally given a relatively higher importance than in Norway). These scores relate to expectable cultural manifestations. For instance, in countries where polled individuals have a higher score on power distance (e.g., China, France), subordinates are more likely to comply with instructions without questioning the leaders than in countries where polled individuals gave less importance to power distance (e.g., the Netherlands, the United States).
Hofstede et al. (2010a) proposes six cultural dimensions: power distance (PDI-CD), individualism (IDV-CD), masculinity (MAS-CD), uncertainty avoidance (UAI-CD), long-term orientation (LTO-CD), and indulgence versus restraint (IVR-CD). For the sake of disambiguation with Multi-Agent Systems (MASs), the suffix “-CD” is added after the standard cultural dimension acronyms. Our simulation focuses on PDI-CD and MAS-CD cultural dimensions, which are now detailed further (other dimensions are left out, as justified in the Section 4). In this article, PDI-CD\(^+\) refers to high PDI-CD cultures, PDI-CD\(^-\) to low PDI-CD cultures, MAS-CD\(^+\) to high MAS-CD cultures and MAS-CD\(^-\) to low MAS-CD cultures.
Power Distance PDI-CD indicates the cultural importance given to power status. PDI-CD can be seen as the cultural response to the following social dilemma: should leaders be the ones making group decisions or should everyone participate in decision-making? Both answers make sense, but conflict with each other. Culture influences the relative preference for one or the other choice, and thus has indirect influence on both individuals and society.
In PDI-CD\(^+\) cultures, individuals tend to value humbleness, obedience, and discipline. Decision-makers are in charge of managing other individuals. Subordinates are more likely to accept revealing personal information to leaders and leaders are more likely to expect being able to access this information. Leaders should manage subordinates tightly and subordinates should comply with instructions from leaders. Both roles are strongly dependent on each other. In this culture, more power is given to decision-makers and strong social inequalities arise. PDI-CD\(^+\) cultures tend to support values from the “conservatism” quadrant from Figure 2.
In PDI-CD\(^-\) cultures, individuals tend to value equality, autonomy and self-direction. Power relationships between individuals tend to be weaker. Thus, individuals tend to prefer democratic decisions (decision makers should make decisions in line with opinions of others) and leadership tends to be more consultative (leaders propose tasks, subordinates accept them or not). Individuals are expected to (be free to) manage themselves, without needing to involve leaders. As a consequence, all members tend to be more involved in decisions and their consequences. Furthermore, individuals tend to be more independent of decision-makers (e.g. more prone to take initiatives). PDI-CD\(^-\) cultures tend to support values from the “openness to change” quadrant, in particular self-direction, from Figure 2. E.g.: Hofstede et al. (2010a): China and Russia score as PDI-CD\(^+\); Scandinavian countries score as PDI-CD\(^-\).
Masculinity versus Femininity (As a side note, Hofstede et al. 2010a use this dimension as a prime factor to explain the emergence of gender differences such as the ‘glass ceiling’. These terms were selected because the MAS-CD dimension shows consistent gender differences, with the exception of very low MAS-CD scores (Hofstede et al. 2010a, p.139).) MAS-CD is related to preferring one of the elements of the following elements: “assertiveness versus modesty”, “mastery versus harmony”, and “equity versus equality”. MAS-CD\(^+\) societies tend to support a preference for assertiveness, mastery, and equity. In MAS-CD\(^+\) societies, individuals tend to value individual performance. Individuals should aim at being the best possible (e.g., successful, excellent, performing, competent), and taking risks for greater rewards. Good performers should be rewarded, equity is important (e.g., rewards based on commission). Individuals are culturally driven to master their environment. This mastery is socially displayed through assertiveness, showing marks of success. Indirectly, this race towards performance tends to promote high competition. MAS-CD\(^+\) cultures tend to support values from the “self-enhancement” quadrant from Figure 2. MAS-CD\(^-\) societies are characterized by a preference towards avoiding conflicts. Individuals should avoid opposing each other, preferably by working together. They tend to be more willing to cooperate, make compromises, look for consensus, support and care about each other. In social interactions, individuals tend to be modest. They tend to avoid raising conflicts by not fulfilling their promises, thus avoiding taking risks. Similarly, such societies support equality as a basis for giving rewards. PDI-CD\(^-\) cultures tend to support values from the “self-transcendence” quadrant from Figure 2. E.g.: Hofstede et al. (2010a): Norway, Sweden score as MAS-CD\(^-\); Italy, Japan score as MAS-CD\(^+\). Previous research has explored cultural influence by empirical research, which at best establishes correlations between factors, but is unable to provide explanations and insights into the influence of culture on behavior and especially on the way it appears on the macro level derived from the micro level. One way to explore this relation is through the use of simulations, as explained above.
Cultural dimensions & Schwartz values
Correlations were found between the set of values carried by individuals and groups and their scores on cultural dimensions. Hofstede et al. (2010a), Schwartz (1994), Smith et al. (2002) empirically and conceptually link Schwartz values and Hofstede cultural dimensions to each other. These values are set by the culture of the agent. PDI-CD\(^+\) cultures promote the values of obedience and social order. PDI-CD\(^-\) cultures promote the values of self-direction, freedom, and autonomy. Higher autonomy from subordinates and more democratic decision-making is expected. MAS-CD\(^+\) cultures promote the achievement, success and ambition. MAS-CD\(^-\) cultures promote the values of benevolence, helpfulness and harmony.
Previous Research
Previous research on replicating the influence of culture can be split into four parts: replicating the dynamic of cultural propagation, models of culture for multi-agent systems (MAS) applications, models of the influence of culture on individual decisions, and models aiming to replicate collective cultural phenomena based on individual cultural influences.
Cultural propagation Models
This category of models simulate cultural dynamics, i.e. the evolution of culture over time (Axelrod 1997b; Epstein & Axtell 1996; Gabora 1997) (e.g., adoption of technology, cultural polarization, inter-cultural conflicts, memetics). While mimicking certain properties of cultural dynamics, these models fall out of the scope of this article because they tend to abstract away from the influence of culture on individual decisions and on collective outcomes. Such models have been left out of this paper, as we aim to model the day-to-day culturally-sensitive activity of a business, rather than long-term cultural dynamics.
Cultural models for operational applications
Models included in this category focus on modeling certain aspects of the influence of culture on individual decisions. These models aim at replicating operationally useful aspects of culture to enhance coordination or to smoothly interact with others, that is, without aiming for perfect correspondence with culture. Available coordination models introduce cultural practices (e.g., symbols: agent communication languages such as FIPA 2002; rituals: norms, Vázquez-Salceda et al. 2005) and values (Antunes & Coelho 1999; Cranefield et al. 2017; Vanhée 2015). While offering inspirational elements, the realism of these models is only partially validated. Furthermore, they tend to introduce aspects for handling operational concerns, but not for simulation.
Micro-level cultural models
Models of this category aim to tightly replicate micro-level cultural decisions. This category (Borit et al. 2013, 2014b; Degens et al. 2013; Endrass et al. 2013) tends to provide highly detailed individual decisions (e.g., animating virtual characters, influencing what to talk about, whether individuals introduce silences in speeches, whether to trust or not another individual). Nevertheless, the validity of these models regarding collective-level phenomena has not been considered.
Micro-to-macro cultural levels
Models included in this category aim at replicating micro-to-macro cultural phenomena (Dechesne et al. 2012; Hofstede et al. 2010b, 2014; Mc Breen et al. 2011; Vanhée et al. 2014). Hofstede et al. (2010b) show that HCDs can be used as a model to replicate the influence of culture, via a model of trading organizations. Vanhée et al. (2014) introduce a BDI (Belief Desire Intention) decision model that integrates the influence of culture, values, and needs on decisions, and that replicates collective properties of the influence of culture on organizational performance. Using the GSSs methodology, the model proposed and validated by Dechesne et al. (2012); Mc Breen et al. (2011) explains how culture influences the emergence of a collective acceptance of norms in a society, via a simulation of agents complying (or not) to a smoking ban norm in a pub, whereas Hofstede et al. (2014) explain how gender-inequalities emerge within a society, via a model of children interacting on a playground.
The technical content of these models can be re-used for our application. As a general principle, most of these simulation models rely on the KISS principle (Keep It Simple Stupid, Axelrod 1997a). This principle, in keeping with Occam’s Razor, provides that it is preferable to make use of agent decision models and environmental setups that are as simple as possible when aiming to replicate (and explain) an emerging phenomenon. Technically, many of these models rely on decision rules that change depending on culture, and on mathematical formulas that integrate cultural dimensions, represented as integers. While this principle should not be blindly pursued, as argued by Conte & Paolucci (2014), no better alternative stands out. Therefore, this study also relies on the KISS methodology. Regarding the content to be modeled, Conte & Paolucci (2014) argue to integrate generative elements, in our case psychological influences, that enable to consider unexpected situations. Value-based models proposed by, for example, Dechesne et al. (2012), Mc Breen et al. (2011), Vanhée et al. (2014) offer such a psychological and generative influence. The model presented in this article differs from other models in the sense that it focuses on individual-to-individual interactions. This focus enables us to apply the GSSs methodology to establish a theory of cultural interactions, which adds value to former research.
Simulation Overview
The GSSs approach requires the design of a realistic simulation, which is then used as a basis for proposing a theory. The present section introduces an overview of this simulation, which is detailed in the next section and validated in the following one.
Our model captures the activity of business organizations that process independent or splittable tasks. This model is generic and can be instantiated to represent a wide range of organizations (e.g., hair dressing salon, car repairing, administration, etc.). To ground the abstract concepts raised by this generic model into a concrete case, this abstract organization is specified as an IT department that provides helpdesk services for its system users. This organization is referred to as DIT, standing for “Department of Information Technology”. Note that the purpose of this organizational model is to replicate core emerging dynamics of task-based organizations, towards exploring the general influence of culture on these dynamics. Thus, the simulation model should be kept as simple as possible, to avoid the introduction of unnecessary complexity and noise that would offer little additional insight for our exploration. However, for a different purpose, such as predicting the evolution of service quality over the next six months, the model would benefit greatly from the introduction of application-specific concepts and detail, something falls outside of the scope of this paper.
The (individual) micro-level of this simulation is composed of the employees of the DIT, represented by agents. They perform actions such as allocating and working on tasks. Their combined actions lead to the completion of tasks. The macro-level is evaluated based on the collective performance of the DIT, i.e., the aggregated success in (timely) completing allocated tasks.
Environment
The environment consists of incoming requests made by clients. These requests are processed as tasks by the organization. These tasks are given an explicit deadline before which they have to be completed or else they fail. Each task requires a certain amount of worktime for being solved, represented by the expected processing duration (assumed to be the same for all agents, for the sake of simplicity). Agents can accurately estimate this value for normal tasks, but not complex tasks, see below.
Sometimes, clients can request complex tasks. These tasks require more time than expected in order to be solved. As an example of a complex task, a client calls with the problem “my email does not work”. After having checked the details of the mail server, the agent discovers that the problem comes from the network, which thus must be fixed in order to solve the initial problem. When such a complex task arises, the organization is allowed to expand the deadline without impacting organizational performance (e.g., they explain to the client that they depend on a third party and so need more time for completion). Note that a task is recognized as complex only after having attempted to solve the initial problem.
Three specific types of environments, for stressing the performance of the organization in specific directions that are known to be culturally-sensitive (e.g., efficiency, flexibility, robustness) are considered. These various environments and performance indicators are used for validating the simulation against social science expectations in Section 6.
The environment can be intensive, with a steady flow of incoming simple tasks to be quickly solved. This environment highlights the allocation efficiency of the organization. This scenario corresponds to the standard situation of the organization during peak business time. In a real-world scenario, this environment is the environment of, for example, a service in charge of setting up computers. Due to limited space for storing computers, the organization works on “just-in-time” delivery. This task is relatively simple even if variations can occur due to the different computational power of different devices or the amount of setup to perform.
The environment can be complex, with a relatively slow input of incoming tasks that have a chance of being complex. Tasks of this environment, in spite of possibly taking more time than expected, remain relatively easy to handle by the organization (long deadline, sufficient processing power). This situation can happen when the organization helps its clients to work with a complex program: fixing an issue often raises another. This environment stresses on the sensitivity to environmental complexity of the environment.
Finally, the environment can be irregular, when (simple) tasks arise at an irregular pace, with some periods of calm and others of overload. This environment captures, for example, the work of a support team in charge of helping its users working with software that is regularly updated. Each time an update is posted, the organization has to support many clients at once to fix their local issues (e.g., new features causing new bugs or calls for adapting configuration files). This environment stresses on the organizational robustness to congestion.
This environment model captures a set of key culturally-sensitive aspects of organizational life while keeping low model complexity. While further details can be added (e.g., measurements on real-world organizations for determining their time for completing tasks, the effect of exhaustion on performance) at the expense of higher model complexity and validation, the mechanisms introduced by our model globally match the key culturally-sensitive challenges that are faced by task-based organizations (e.g., certain tasks take longer to process, delegating takes time, various organizational performance aspects can be stressed by the environment).
Institutional context: Coordination structure
Theory describes certain properties of the influence of culture while taking the institutional context into consideration. Therefore, we introduced multiple institutional contexts for validating these properties and for better understanding the relations between culture, institutional context, and the micro-to-macro link.
The institutional context consists of a coordination structure (i.e., an organization and rules for coordination). For the sake of exploring the recurrence of the influence of culture in different institutional contexts and the influence of the institutional context on cultural expressiveness (presented in Section 7.1), we considered three types of organizational structures: simple structures, bureaucracies, and adhocracies, as described by Mintzberg & Heyden (1999).
The simple structure, referred to as DIT, relies on direct delegation. Clients issue tasks to the leader. Then, the leader allocates these tasks to subordinates. Subordinates determine whether they accept allocated tasks and, once accepted, work on accepted tasks until completion or failure. If a subordinate has accepted to perform multiple tasks, these tasks are performed one after the other, following a queue order. The bureaucracy, referred to as Rule-DIT, expands DIT by adding strict rules on how agents should behave: leaders must allocate tasks by using a round-robin (give a task to the subordinate that has received its last task the earliest) and sub-ordinates must accept them. The adhocracy, referred to as DIT-Together, is an open variant of DIT. Subordinates are free to cooperate with each other for efficiently tackling long tasks.
Agent decision process
This simulation models the following three main decisions, which both tightly impact coordination outcomes and are easily related to cultural influences:
- Leaders have to decide how to allocate tasks: shall they take time and monitor subordinates or allocate tasks quicker?
- Leaders have to determine whether they allocate a task and if they do, to which agents they allocate the tasks.
- Subordinates have to decide whether they accept tasks allocated to them.
Agent decisions are made based on two influences: rationality and values. Agent rationality forces or forbids rationally obvious choices (e.g., subordinates always refuse tasks when infeasible). Values are represented by Schwartz values, introduced in Figure 2 (e.g. self-direction, obedience, achievement), influence remaining choices (e.g., subordinates valuing obedience always accept tasks, if feasible). Further decision aspects could be considered (e.g., trust, exhaustion), but they are less relevant with regards to the scope of this paper.
The values of the agent are set by its cultural background. Two cultural dimensions are considered: PDI-CD and MAS-CD, generating a total of four culture types: (PDI-CD\(^+\), MAS-CD\(^+\)); (PDI-CD\(^+\), MAS-CD\(^-\)); (PDI-CD\(^-\), MAS-CD\(^+\)) and (PDI-CD\(^-\), MAS-CD\(^-\)). Each cultural dimension promotes certain values, as depicted in Figure 2 and detailed in Section 2.16.
Justifying design choices
As a core design principle, this simulation aims to enable applying the GSSs methodology. A relevant simulation to apply the GSSs methodology should: (1) introduce a setting in which culture influences individual decisions and collective outcomes; (2) replicate this influence in details; and (3) enable a collective phenomenon to be observed. This way, the realism of this simulation can be assessed via a validation and then be used as an exploratory tool for proposing a theory.
The organizational setting presented in this paper is highly culturally-sensitive. Numerous related situations are detailed, both quantitatively and qualitatively, by D’Iribarne (1989), Hofstede et al. (2010a), Vanhée et al. (2013). We focus on the PDI-CD and MAS-CD dimensions, as they have been well-studied: straightforward connections can be made between these dimensions and individual-level decisions as well as macro-level cultural phenomena. PDI-CD is directly related to power structures and interactions that are brought about by organizations. MAS-CD is easily related to risk-taking and individual performance, which are both significant in organizations. The integration of additional HCDs (e.g., individualism, uncertainty avoidance, long-term orientation, and indulgence vs. restraint) is left for future work, as integrating more HCDs in the current model would make it very complex and the current HCDs are sufficient for highlighting interesting conclusions. Finally, this model can also be relatively accurately validated, by being relatable to numerous collective properties of the cultural influence (testing the model against numerous relations between culture and organization as depicted by (Hofstede et al. 2010a), such as flexibility, robustness, capability for handling simple vs. complex tasks).
Regarding the exploratory part of the GSSs methodology, this simulation offers a rich playground in which theories can be tested. Agents are given sufficiently detailed actions to explore cultural influences. Furthermore, this simulation enables to study in great detail how agent individual actions connect to collective outcomes, by “unwinding" the simulation. The other way around, the simulation facilitates the explanation of collective outcomes via a chain getting down to cultural influences on individual decisions. These explanations can easily be connected to more generic theories for explaining the micro-to-macro influence of culture.
Simulation Model
This section formalizes the simulation model described in this article and informally introduced in Section 4. We first introduce the various entities (e.g. agents, tasks) that compose it. Next, we describe the simulation loop of the environment. Then, we describe agent decisions. This model is implemented using NetLogo (version 6.0.1) and illustrated in Figure 3.
Simulation entities
This section introduces the core non-agent entities composing our simulation model. These entities are time, tasks, environment types and coordination structures. Their properties and their relations are depicted in Figure 4.
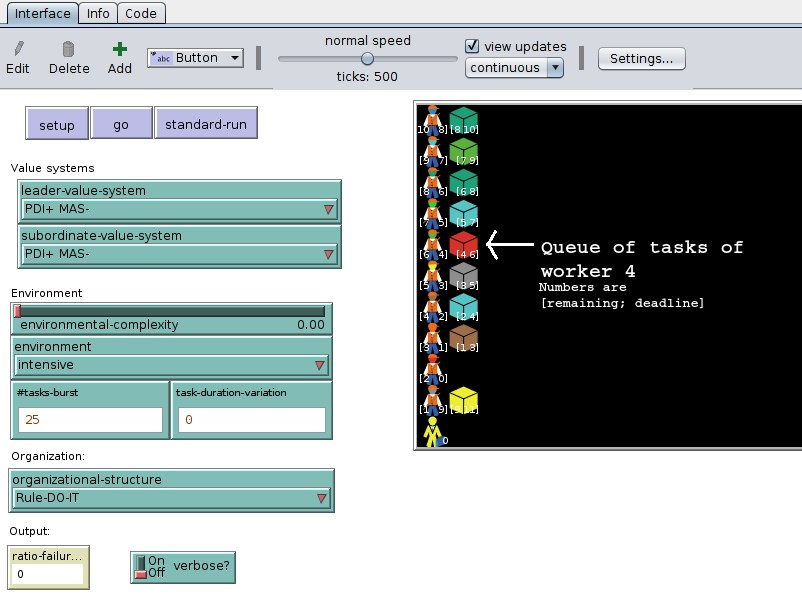
Time
The variable \(\mathit{time}\in \mathbb{N}\) represents the current amount of worktime units that have been spent so far. Each execution of the simulation loop models the passing of one worktime unit, therefore incrementing \(\mathit{time}\) by one.
Tasks
The set of tasks being currently processed by the organization is represented by \(T\). Incoming tasks are added to \(T\). Completed or failed tasks are removed from \(T\).
Each task \(\mathit{task}\in T\) is related to a deadline, a remaining amount of work, and a complexity. The deadline of a task is represented by \(\mathit{deadline}:T\rightarrow \mathbb{N}\), the time at which \(t\) will be failed. The amount of worktime units that remains to be performed for completing a task is represented by \(\mathit{remaining}:T\rightarrow \mathbb{N}\). \(\mathit{remaining}(\mathit{task})\) decreases by one each time an agent performs a work action on task \(t\). The complexity of a task is modeled by \(\mathit{complex?}:T\rightarrow \{\top ,\bot\}\) where \(\mathit{complex?}(\mathit{task})=\top\) means that \(t\) is complex (i.e., the task needs more time in order to be completed) and complex \(\mathit{complex?}(\mathit{task})=\bot\) means that \(t\) is simple. Agents can see that a task is complex only when they have performed the operation to solve it and discovered the presence of a follow-up problem.
Two properties are derived from these base variables: feasibility and completion. When the remaining time is too short, a task can become infeasible. Formally, a task \(t\) is infeasible if \(\mathit{time} + \mathit{remaining}(\mathit{task}) > \mathit{deadline}(\mathit{task})\). Agents rationally fail and discard tasks as soon as they become infeasible, agents only work on feasible tasks. A task is completed if \(\mathit{remaining}(\mathit{task}) = 0\) and \(\mathit{complex?}(\mathit{task})=\bot\). If \(\mathit{remaining}(\mathit{task}) = 0\) but \(\mathit{complex?}(\mathit{task})=\top\), then the task and its deadline are to be expanded (see Section 5.18). Organizational performance is measured by the ratio of successfully completed tasks.
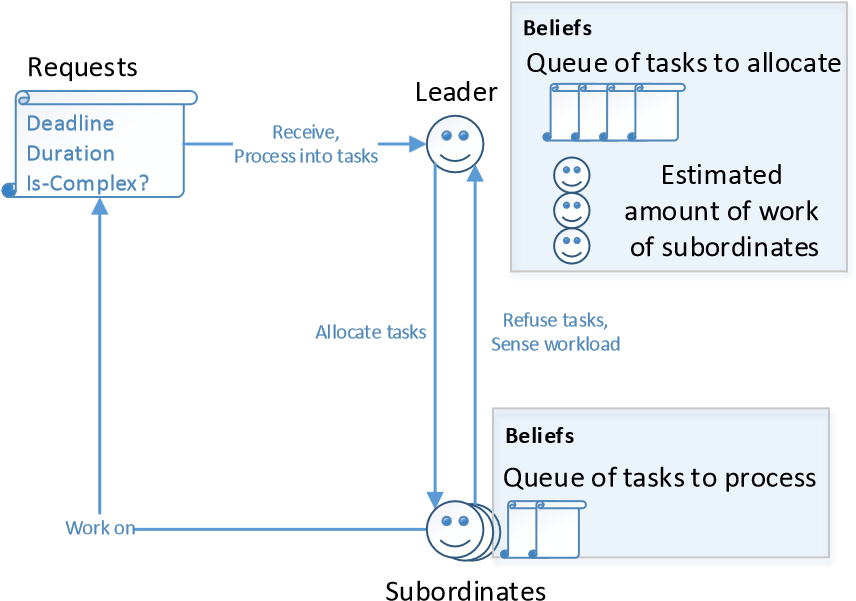
Intensive | Complex | Irregular | Coop-Intensive | Coop-Complex | Coop-Irregular | |
\(\mathit{\#tasks}\) | \(1\) | \(1/2\) | \(k/20\) | \(1/10\) | \(1/15\) | \(k/30\) |
\(\mathit{base\_duration}\) | \(10\) | \(5\) | \(5\) | \(75\) | \(50\) | \(25\) |
\(\mathit{deadline}\) | \(12\) | \(15\) | \(15\) | \(35\) | \(50\) | \(35\) |
\(\mathit{environmental\_complexity}\) | \(0\) | \(k\) | \(0\) | \(0\) | \(k\) | \(0\) |
\(\mathit{variation}\) | \(k\) | \(0\) | \(0\) | \(5\times k\) | \(0\) | \(0\) |
\(\mathit{\#subordinates}\) | 10 | 10 | 10 | 10 | 10 | 10 |
Finally, special tasks have been designed to simulate collaborative work (see DIT-Together organizations in Section 5.11). These tasks are normally infeasible when performed by single agents. However, agents can split tasks into subtasks that can be shared with other agents. Splitting up a task takes five time units and splits the task into five equal subtasks (splitting should take a bit of time, but not too much). A task is completed when all of its subtasks are completed. If a subtask is not completed in due time, the whole task fails.
Task-generation
The different environmental types (intensive, complex, and irregular, introduced in Section "Simulation Overview") are modeled by changing the parameters that set the generation of incoming tasks. These parameters are chosen to introduce situations in which performance is acknowledged to be culturally-sensitive. In our case, we consider three types of environments: simple and inventive environments that stress on efficiency, complex environments that stress on flexibility, and irregular environments that stress on robustness.
The generation of tasks depends on the intensity or pace of incoming tasks, the variability of incoming tasks and the complexity of tasks. Intensity defines the number of incoming tasks at any given time, represented by \(\mathit{\#tasks}:\mathbb{N} \rightarrow \mathbb{N}\), where \(\mathit{\#tasks}(\mathit{time})\) is the number of tasks that will arise during time-slot time. Variability is simulated by a uniform variation with regard to a base duration of incoming tasks. The base duration of a task is \(\mathit{base\_duration}\in \mathbb{N}\). The variability is defined by \(\mathit{variation}\in\mathbb{N}\). The initial duration of a task is drawn uniformly in \([\mathit{base\_duration}-\mathit{variation};\mathit{base\_duration}+\mathit{variation}]\). Environmental complexity is represented by the variable \(\mathit{environmental\_complexity}\in [0,1]\). The probability of a new task to be complex is \(\mathit{environmental\_complexity}\).
In the intensive environment, tasks come at a high pace, compared to the number of agents in the organization (10 agents). Tasks are to be processed quickly. Failures mostly arise from failed allocations of tasks and idling subordinates. In the complex environment, tasks can be complex and arise at a relatively low pace. The challenge raised by this environment lies in deciding on when to commit to tasks at the right time. Failures occur when an agent queues multiple tasks hoping to complete them all in due time, and discovers too late that some are more complex than expected. The irregular environment emphasizes on organizational congestion robustness Dodds et al. (2003) (i.e., capability for handling many tasks at once). The organization has sufficient work-power to cope with all incoming tasks, but allocation output has to be fast to prevent the leader overloading and thus failing to allocate tasks in due time. These environments are expanded for fitting the processing power of DIT-Together organizations (introduced in Section "Coordination Structures"). The detail of all the parameters per environment is presented in Table 1.
Coordination structures
The coordination structure consists of an organization with two roles: one leader and several subordinates. Leaders are in charge of allocating tasks to subordinates and subordinates are supposed to perform allocated tasks. Figure 5 illustrates the interaction loop.
The set of agents is represented by \(A\). The leader agent is represented by \(a_l\in A\). Subordinate agents are represented by the set \(S=A\setminus \{a_l\}\). In our simulations, \(|S|=10\). This number was selected for the sake of realism (10:1 is a reasonable subordinate:leader ratio, which actually matches the size of the IT-support organization at our university) and computational performance. Tests highlight that similar results are achieved for different organizational sizes, provided the organizational workload is comparable.
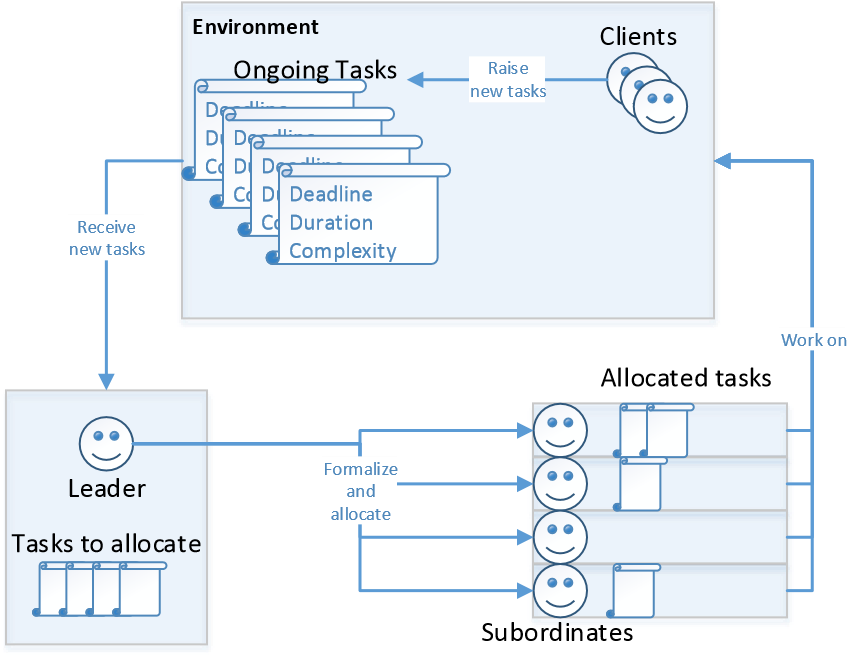
The coordination structure allocates a queue of tasks to each agent. This queue defines the tasks to be processed and the order in which they have to be processed. Formally, this queue is represented by \(\mathit{queue_a}\) of tasks in \(T\) for each agent \(a\in A\). The next task to be processed is the first task of \(\mathit{queue_a}\). This task is represented by \(\mathit{next\_task_a}\).
To explore the combined influence of culture and organizations, three specific coordination structures are defined: a simple structure (DIT), a bureaucracy (Rule-DIT) and an adhocracy (DIT-Together), from the categorization by Mintzberg (1980), as explained in Section 4.12.
DIT relies on a simple structure, which relies on direct delegation as its prime coordination mechanism. Leaders are linked to subordinates in a top-down manner. In this organization, leaders are free to propose tasks to their chosen subordinate. Subordinates are (arguably) free to decide whether they accept or refuse allocated tasks.
Rule-DIT relies on a machine bureaucracy. This organization relies on standardization (rules) as a core coordination mechanism. In this organization, leaders must allocate tasks in a round-robin way to subordinates, such that the next task will be allocated to the subordinate that has received its previous task the longest time ago. Subordinates must accept all incoming tasks.
DIT-Together relies on an adhocracy. This form of organization relies on mutual adjustment (free interaction) as a core coordination mechanism. With this form of organization, agents can split tasks, freely exchange them, and collaboratively work on them. Leaders still receive and delegate incoming tasks, but anyone, including subordinates, can split and exchange tasks with each other.
Simulation loop
The simulation loop formalizes the evolution of agents, organizations, and environments. Each round represents one "worktime unit", with relative duration scales with the tasks performed by the organization. As each scenario focuses on a single type of tasks, all worktime units are scaled with each other and the run is internally consistent. The intensive environment, which features classic IT support helpdesk missions, has a worktime unit scaled to two minutes (thus 10-worktime unit long tasks are solved within 20 minutes); the complex environment, which features tasks of network and program debugging, has a worktime unit scaled to 30 minutes (thus, 5-worktime unit long tasks are solved within 2.5 hours, but require \(30\times 0.5 = 15\) hours of extra work when complex); the irregular environment, which features solving many small and repetitive issues after an up-date, has a worktime unit scaled to two minutes (thus 5-worktime unit long tasks are solved within 10 minutes). These values are derived from Table 1. In our experiments, simulations are run for \(500\) rounds, which provides robust indication of the average success rate.
The steps of this simulation loop are introduced one by one in the following paragraphs. At each round, time is incremented by \(1\); new tasks are generated and added to the leader’s queue according to the definition of the environment; failed and infeasible tasks are cleared; the leader decides on how to allocate tasks (monitor & allocate or fast allocation) and then all subordinates work on their first task. If the leader decides to monitor, then the leader selects the next subordinate to be monitored using a round-robin mechanism and acquires the information on the subordinate workload. When leaders attempts to allocate tasks, they first decide on whether they should allocate the first task on their queue.If so, the leader selects, based on its beliefs, the least loaded subordinate. Then, the subordinate agent decides on whether it accepts the task or not. If it does, the task is added to the subordinate’s queue. The whole process is illustrated on Figure 6 and by Algorithm 1 and Algorithm 2.
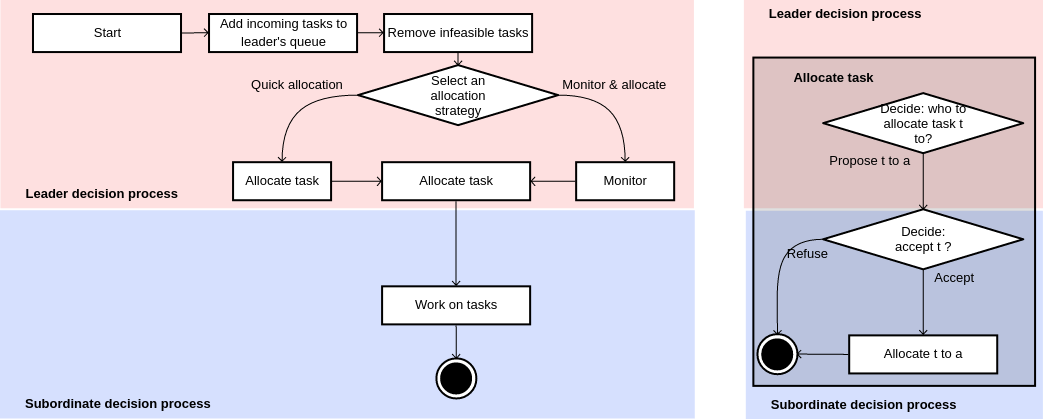
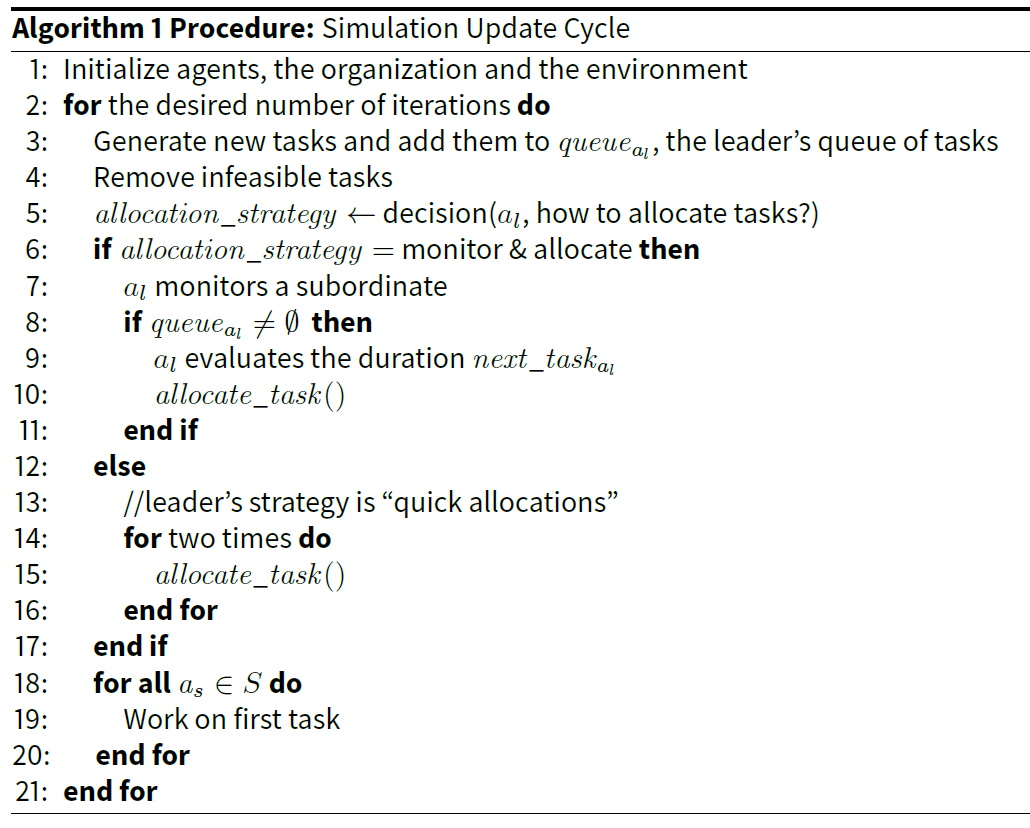
Initialization: The environment sets \(T=\emptyset\). Agent queues are emptied.
Generating New Tasks: at each round, \(\mathit{\#tasks}(\mathit{time})\) tasks are generated and added at the tail of the leader’s queue.
Remove Infeasible Tasks: tasks that cannot be completed before their deadline are removed from the agents’ queues. Note that subordinates ignore whether their tasks are complex. In this situation, they remove infeasible tasks only when they discern that their current task is complex. When discovering such a task, agents can then deduce that next tasks in the queue cannot be completed in due time. In the cooperative scenario, agents take into consideration that they can split tasks for determining whether they are infeasible.
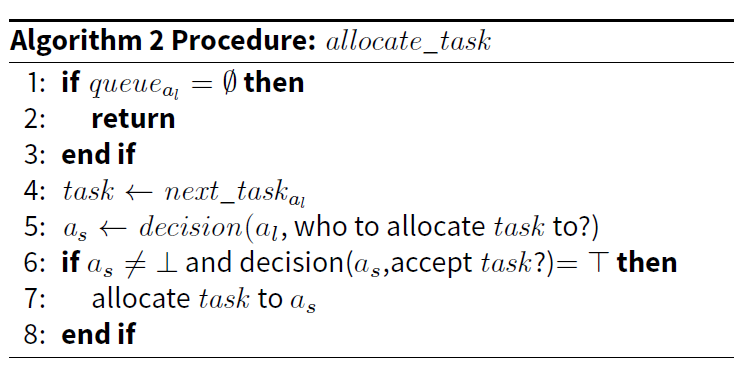
Decision: How to Allocate Tasks? Detailed in Section 5.31.
Monitoring a Subordinate: leaders select a subordinate and monitors it. Leaders perceive the expected amount of work for this subordinate, ignoring whether this task is complex or not. Formally, the amount of work is represented by:
$$\sum_{\mathit{task}\in \mathit{queue}_{a_s}} \mathit{remaining(\mathit{task})}$$ |
Evaluating the Duration of the Next Task to Allocate: the leader perceives the expected duration of the task that is currently trying to allocate. Note that the leader ignores whether the task is complex.
Decision: Whether and Who to Allocate the Next Task? Detailed inSection 5.31.
Decision: Accept Proposed Tasks? Detailed in Section 5.31.
Allocating tasks: The task moves from the head of leader’s queue to the tail of the allocated subordinate’s queue.
Working on Tasks: At each round, subordinates work on the tasks they are allocated. Formally, each subordinate \(a_s\in S\) works on \(\mathit{next\_task_{a_s}}\), if \(\mathit{queue_{a_s}}\neq \emptyset\). In this case, \(\mathit{remaining(next\_task_{a_s})}\) is decreased by \(1\).
If \(\mathit{remaining(task)}=0\) and \(\mathit{complex?}(\mathit{task})=\bot\), then the task is completed and removed from the queue. Else, when \(\mathit{remaining(task)}=0\) and \(\mathit{complex?}(\mathit{task})=\top\), then the task is found to be more complex than expected and thus requires additional work for being completed. In that situation, \(\mathit{remaining(task)}\) is set to \(30\) (more work), \(\mathit{deadline}(\mathit{task})\) is increased by \(40\) (extended deadline) and \(\mathit{complex?(task)}\) is set to \(\bot\) (the task is no longer complex).
Agent decision process
This section details how agents make decisions. To keep the model as simple as possible, we rely on pre-set decision rules. While simple, these rules still offer relatively high realism and expressive power, as argued later in this section.
Memory structure
This section introduces the elements of memory that agents locally store and update throughout their decision cycle. Beliefs: Each agent \(a\) has accurate information about \(\mathit{time}\), and their queue of tasks \(\mathit{queue}_a\). For each task \(t\in \mathit{queue}_a\), agent know \(\mathit{deadline}(\mathit{task})\), \(\mathit{remaining}(\mathit{task})\). Agents ignore whether \(\mathit{complex?}(\mathit{task})\). Agents can infer whether their tasks are feasible, the first tasks to fail in an infeasible queue, and the feasibility of adding a new task. For each subordinate \(\in A\), leaders record \(\mathit{estimated\_remaining}_a\in \mathbb{N}\), the estimated amount of remaining work to be performed by \(a\). This value decreases at each round, increases when the subordinate accepts a new task, and is updated to the expected value when the subordinate is monitored.
Value system (and Cultural Influence): agents are allocated a set of values, part of the set of values introduced in Figure 2: obedience, social order, self-direction, freedom, autonomy, achievement, success, ambition, benevolence, helpfulness, harmony. As detailed in Section 2.16, the set of values promoted by an individual is related to his/her culture and scores on the Hofstede Cultural Dimensions as follows: autonomy, self-direction, and freedom values are promoted by PDI-CD\(^-\); obedience and social-order are promoted by PDI-CD\(^+\); achievement, success, and ambition are promoted by MAS-CD\(^+\) values; and helpfulness and harmony are promoted by MAS-CD\(^-\) values.
Decisions
This section details the agent decision rules. As introduced in Section 4.14, the agent decision process consists of two parts: a rational decision process, which removes rationally suboptimal choices and value-driven preferences, which help in turn to resolve ties.
How to Allocate Tasks? Leaders have to decide how to allocate tasks, be selecting one of the following two task-allocation strategies: 1) quick allocation: leaders repeat twice “allocate”; and 2) monitor & allocate: the leader can monitor one subordinate \(s\) and allocate one task. The monitoring step informs the leader about the expected amount of remaining work in \(\mathit{queue}_s\), excluding unexpected work due to task complexity. For organizations involving cooperation (DIT-Together), leaders are given a third option: 3) split a task into subtasks.
Rational Process: All alternatives are arguably rationally acceptable: quick allocation is more efficient while monitor & allocate is more accurate. For cooperative work, splitting tasks is more accurate, while allocating them is more efficient.
Value-Driven Preferences: “Quick allocation” is favored by PDI-CD\(^-\) values, notably by the self-direction and freedom Schwartz values. With quick allocation, subordinates are le in charge of managing their own workload, while improving allocation efficiency. “Monitor & allocate” is favored by the PDI-CD\(^+\) values, notably by the obedience and humility Schwartz values. When making this choice, leaders gain information about the sub-ordinates’ workload, empowering leaders to decide alone on who to allocate tasks to. For similar arguments, “splitting task” is promoted by PDI-CD\(^+\) values: this strategy gives more control to leaders on the distribution and allocation of tasks among the subordinates. Thus, PDI-CD\(^+\) leaders in DIT-Together first split tasks and then allocate them, while PDI-CD\(^-\) leaders quickly allocate collaborative tasks and let subordinates split and share them.
Who to Allocate to? The leader decides whether to allocate \(\mathit{next\_task_{a_l}}\). If so, the leader decides to which subordinate to allocate the task to. The set of allowed actions for this decision is \(\{\mathit{allocate(a)}|a\in S\}\cup \bot\), where \(\bot\) means “no allocation”.
Rational Process: The target of the allocation is a subordinate with the least believed amount of work. If the leader is uninformed about subordinates’ workload, tasks are allocated via a round-robin mechanism, which offers an a priori balanced task distribution.
A dilemma occurs when leaders expect a subordinate to be working on a task. When leaders immediately propose a new task, they can cause a premature commitment, which can lead to failures if the current task is more complex than expected. By waiting for the subordinate to be free, the failure can be avoided. However, delaying allocations can entail inefficiencies (and thus possibly failures), when multiple subordinates become available at the same time. In this case, the subordinates have to (passively) wait for the leader to offer them a task.
Value-Driven Preferences: MAS-CD\(^+\) values promote ambition and achievement, which favour early allocation to optimize the productivity of leaders and subordinates (minimal idling). On the contrary, MAS-CD\(^-\) values favour late commitment, to avoid unnecessary failures that may break harmony.
Accepting Tasks? Subordinates that are proposed tasks have to decide whether they accept them or not. Agents select one of the two actions: “accept” or “reject”.
Rational Decision: The answer is always “accept” if the agent task queue is empty. The answer is always reject if adding this task implies a failure.
A dilemma occurs when agents have already at least one task to perform. On the one hand, accepting another task may lead to failures if one of the already accepted task takes longer than expected. On the other hand, refusing the task incurs additional management costs to the leader, which can also lead to failures.
Value-Driven Preferences: subordinates favouring obedience and social order, promoted by PDI-CD\(^+\) cultures accept orders, as they are given by their hierarchy. If agents do not convey such values, other values are expressed. As for the decision "who to allocate tasks to", subordinates helpfulness and harmony values, promoted by MAS-CD\(^-\) cultures, prefer to refuse tasks if they are already processing one. Subordinates with values of achievement, success, and ambition, promoted by MAS-CD\(^+\) cultures, prefer to accept new tasks if possible to avoid being forced to idle while waiting for propositions.
Simulation Experiments
As a next step in applying the GSS methodology, the simulation needs to be validated. This validation assesses the reliability of this model, which can then be used confidently for proposing theories.
This section aims to investigate whether our simulation model replicates the emerging influence of culture on collective outcomes. Therefore, we tried to show that the influence of culture on individual decisions and on collective outcomes is replicated within our model. The replication of cultural influence on individual decisions is achieved by construction. The justification of the match between our simulation and social science theories is detailed in Section 5. This section aims to validate the remainder: showing that our model replicates culture influence on collective outcomes.
In order to perform this validation, we explored the core acknowledged properties of culture influence on emerging outcomes in organizations under possible cultural influences. We found five main categories of emerging properties. Each category is further detailed in the following section. Before going into the details of the simulation output, we should briefly note the range of simulations setups that can be generated by our model. Our simulation model provides three sources of input. First, we can set the environment type and its variables: intensive environment and the average deviation; complex environment with the complexity risk; irregular environment with the amount of task per burst. Second, we can set the organizational type: DIT, Rule-DIT, and DIT-Together. Third, we can set the cultural background of leaders and subordinates, by setting their PDI-CD and MAS-CD scores. Each experiment is repeated 100 times and output is averaged. A sensitivity analysis is detailed in the Appendix.
Property 1: Acknowledged influences per cultural dimension on collective performance
Hofstede et al. (2010a) introduce specific properties of the influence of PDI-CD and MAS-CD on collective performance. This section studies the match between these properties and the behaviour of our model in the standard DIT organization.
Organizations with PDI-CD\(^+\) cultures tend to perform better in simple environments: Hofstede et al. (2010a) argue that, in simple environments, organizations with PDI-CD\(^+\) cultures tend to perform better because leaders can unilaterally perform accurate allocations. Direct allocation, supported by PDI CD\(^+\)cultures, is more time-efficient than democratic negotiation supported by PDI-CD\(^-\) cultures.
In order to investigate this property, we consider the performance of the organization in various cultures in the “intensive” environment, presented in Section 5.8. This environment is simple (no complex tasks) and easily leads to failures when tasks are inefficiently allocated.
Results presented in Figure 7(a) show that our simulation replicates this property. Results indicate that PDI-CD\(^+\) organizations perform better in this simple environment, for equal setup on MAS-CD and for all degrees of duration variability.
Complex environments are best handled by organizations with PDI-CD\(^-\) and MAS-CD\(^-\) cultures: Hofstede et al. (2010a) argued that individuals in such a culture tend to handle better complex problems due to lower pressure from hierarchy (PDI-CD) and from setting high performance goals (MAS-CD).
In order to investigate this property, we consider the performance of the organization in various cultures in the “complex” environment. The influence of complexity can be emphasized by altering the environmental complexity variable and thus increasing the probability that tasks are complex.
The results presented in Figure 7(b) match expectations inferred from the theory of Hofstede et al. (2010a). These results indicate that, when environmental complexity increases, MAS-CD\(^-\) organizations tend to outperform MAS-CD\(^+\) organizations. Likewise, organizations with MAS-CD\(^-\), PDI-CD\(^-\) cultures tend to achieve better results than organizations with PDI-CD\(^+\) cultures.
The only exception occurs in comparing organizations with PDI-CD\(^-\) vs. PDI-CD\(^+\) that both have MAS-CD\(^+\) cultures. This exception is raised by a modelling simplification: leaders with MAS-CD\(^+\) cultures are, here, too risk-taking, which combines poorly with the fast allocation made by leaders with PDI-CD\(^-\) cultures. Subordinates with PDI-CD\(^-\) MAS-CD\(^+\) cultures are allocated tasks fast, commit early and therefore make many mistakes when facing complex tasks. Conversely, PDI-CD\(^+\) MAS-CD\(^+\) are allocated tasks at a lower pace with a leader having a better oversight on the workload of the agents. This modelling problem would be fixed in providing subordinates with the capability of evaluating earlier whether their task is complex.
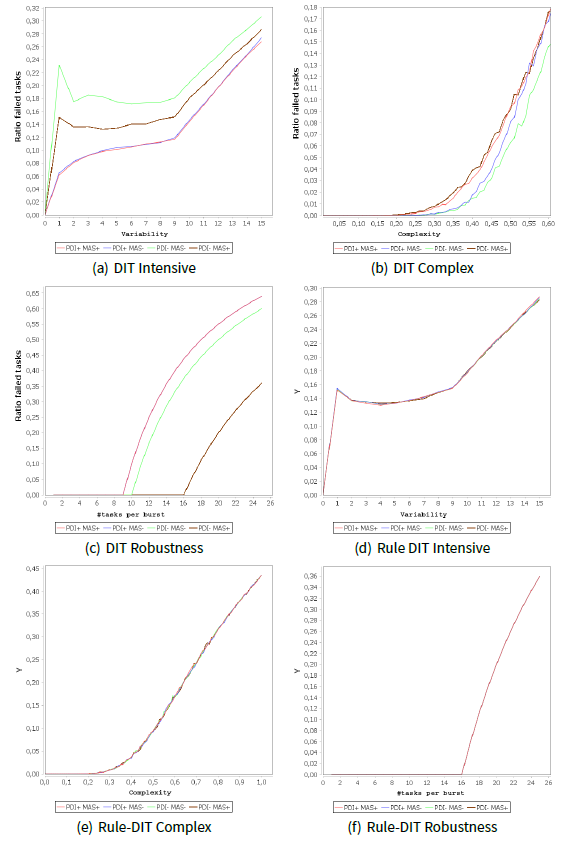
PDI-CD\(^-\) organizations tend to be more robust than PDI-CD\(^+\) organizations: PDI-CD is correlated with a tendency towards centralization. Centralization often creates critical failure points and bottlenecks for sudden overloads, which leads to lower robustness.
The influence of culture on (congestion) robustness is emphasized by altering the cultures in the “irregular” environment. In particular, the amount of tasks per bursts further stresses the organizational robustness.
Results presented in Figure 7(c) match this expectation. These results indicate that PDI-CD\(^-\) organizations perform better when many tasks arise at once, under any MAS-CD orientation. In other words, our simulated PDI-CD\(^-\) organizations are more robust than simulated PDI-CD\(^+\) organizations.
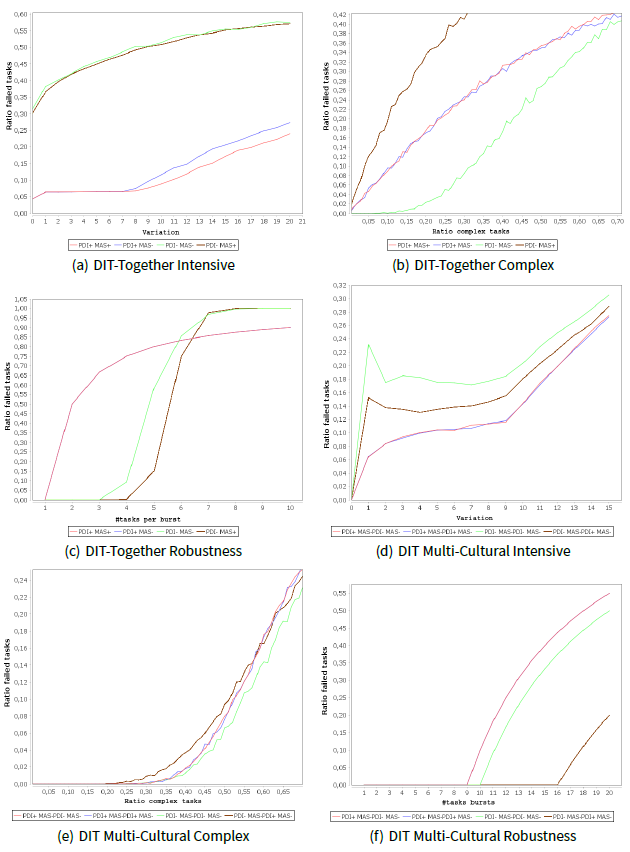
Organizations with MAS-CD\(^+\) cultures tend to handle better heavy workloads. Hofstede et al. (2010a) explained that, because of a tendency for the “great risks for great rewards” attitude, MAS-CD\(^+\) organizations tend to perform better when handling heavy workloads.
This assertion is emphasized in intensive and irregular environments, which raise such heavy workloads.
Results presented in Figure 7(a) and Figure 7(c) match this expectation. In both environments, for any set of cultural preference towards PDI-CD, MAS-CD\(^+\) organizations perform better than MAS-CD\(^-\) organizations.
Property 2: The influence of culture is similar across organizations
Hofstede et al (2010a, 312-315) indicated that organizations with similar (national-level) cultures tend to display similar individual and collective behaviours, in spite of different organizational features[1]. This property can be verified in our simulation by checking the tendencies per cultural background in various organizational settings. The set of relative tendencies per culture introduced in Property 1 should also be observable in Rule-DIT and DIT-Together. In concrete terms, we re-ran the same experiments as run for Property 1, albeit with altered organizational backgrounds.
Results regarding Rule-DIT, introduced in Figure 7(d), Figure 7(e), Figure 7(f), are inconclusive since the influence of culture is not visible in this organization[2]. These results do not either validate or invalidate the hypothesis.
Results regarding DIT-Together, introduced in Figure 8(a), Figure 8(b) and Figure 8(c), are in line with expectations. With a similar analysis as for Property 1, this figure shows the same patterns as provided by Hofstede et al. (2010a) and presented for DIT. Namely, organizations with PDI-CD\(^+\) cultures tend to perform better in simple environments; PDI-CD\(^-\) and MAS-CD\(^-\) tend to perform better in complex environments; PDI-CD\(^-\) organizations tend to be more robust than PDI-CD\(^+\) organizations; MAS-CD\(^+\) organizations tend to handle heavy workloads better.
As a side note, similar results can be found in the study of Hofstede et al. (2010b), which simulates business-to-business trade. Our simulation confirms their Hypothesis 6: “In [MAS-CD\(^+\)] societies, agents deal rapidly but many negotiations fail, due to the combination of impatience and high quality ambitions”. The same can be said about their Hypothesis 7: “In MAS-CD\(^-\) societies, negotiations proceed slowly, but with a low failure rate”.
Property 3: Culture influences the resolution of coordination situations
As illustrated in the introduction section, argued by Hofstede et al. (2010a), and studied in detail on real organizations by D’Iribarne (1989), culture deeply influences how coordination is set and applied. For instance, D’Iribarne (1989) explains that, when facing troublesome situations, members of the French organization (PDI-CD\(^+\), average MAS-CD) tend to try to fix the issue by themselves at best, without reporting the issue. Members of the Dutch organization (PDI-CD\(^-\), MAS-CD\(^-\)) tend to raise the issue in informal meetings and find consensual agreement on how to tackle it. Members of the United States organization (PDI-CD\(^-\), MAS-CD\(^+\)) tend to prefer raising the issue as a challenge and offer “contractual” rewards for motivating members to tackle it. Such a property is best observed via a qualitative study of the coordination patterns that arise within our model. As such, the GSSs approach is very helpful by enabling to run step-by-step decisions and interactions.
The influence of the PDI-CD on coordination can be characterized as follows. In PDI-CD\(^+\) organizations, the allocation process is centred around leaders. Leaders take the responsibility of managing subordinates’ workload and allocating tasks accordingly. In return, assuming that allocations are performed carefully, subordinates comply with leaders’ orders without questioning. This direction is further highlighted when comparing DIT and DIT-Together when leaders take on the charge of splitting and allocating collaborative tasks.
In PDI-CD\(^-\) organizations, the allocation process is equally shared between leaders and subordinates. Leaders are simple coordination interfaces without any visibility of the workload of subordinates (no \(\mathit{estimated\_remaining}_a\) beliefs). Subordinates are in charge of managing their own workload and decide on their own whether to accept tasks or not. This direction is further highlighted in DIT-Together, when subordinates split and exchange tasks on their own.
Likewise, as suggested by Hofstede et al. (2010a), the influence of MAS-CD on coordination in our model is observed in terms of eagerness for commitments and tolerance for higher workload. Leaders and subordinates with MAS-CD\(^+\) commit early to giving and accepting allocations. Leaders are more ready to allocate tasks early to subordinates, even if these are already processing a task. Likewise, subordinates can accept more tasks at once. Conversely, MAS-CD\(^-\) organizations show late commitment for allocations. Leaders and subordinates make sure that the latter are loaded with only one task at a time.
As a side note, these observed patterns match another strong empirical finding by Hofstede et al. (2010a): influences of PDI-CD and MAS-CD are independent. We observe four coordination patterns: “last minute allocation with centralized workload management”, “last minute allocation with decentralized workload management”, “early allocation with centralized workload management”, “early allocation with decentralized workload management”. Both cultural dimensions impact coordination relatively independently. This independence matches the empirical independence relating PDI-CD and MAS-CD as presented by Hofstede et al. (2010a).
Property 4: Multiculturalism often raises cultural conflicts
Hofstede et al. (2010a) introduce the concept of cultural conflicts (or clashes) that can arise during encounters of individuals of different cultures. Cultural clashes are unintended disagreement (e.g. distrust) or conflicts between multiple parties for which the origin can be explained in terms of cultural differences (e.g. mismatching expectations, misunderstandings).
The replication of this phenomenon by our model can be emphasized by running simulations in which individuals have different cultural backgrounds. Due to the informal nature of such conflicts, a qualitative study can provide strong insight into whether our model replicates these conflicts or not.
Hofstede et al. (2010a) detail a concrete example of a French leader (PDI-CD\(^+\)) working at a Swedish organization (PDI-CD\(^-\)). The leader gives an order to a subordinate with a PDI-CD\(^-\) culture. The leader expects the subordinate to immediately comply with the order. Instead, the subordinate questions the reasoning of this or-der. This scenario is observed in our simulation by setting a leader with a PDI-CD\(^+\) culture with subordinates with PDI-CD\(^-\) cultures. If this subordinate is already busy, the subordinate will refuse the task allocated by the leader. This situation goes against the expectations of both agents[3]. The leader’s decision is based on the expectation that the subordinate should accept the task (justifying why leaders make careful allocations). The subordinate’s decision is based on the expectation that leaders “propose” and not “order”. Arguably, this situation is prone to conflicts.
Likewise, our simulation replicates expectable conflicts when setting organizations with leaders with PDI-CD\(^-\) cultures and subordinates with PDI-CD\(^+\) cultures. In this setting, both the leader and the subordinate expect the other to manage the subordinates’ workload. Leaders carelessly delegate to subordinates, who blindly accept feasible incoming tasks, leading to poor workload balance and thus failures.
Our simulation also replicates conflicts arising when individuals have cultures that differ along the MAS-CD axis. In PDI-CD\(^-\) organizations, these conflicts are visible along subordinate-to-subordinate indirect interactions. Subordinates with MAS-CD\(^+\) cultures commit early to tasks, whereas those with MAS-CD\(^-\) cultures commit late. When carried out, a skewed distribution of tasks can be observed: in the organisation, subordinates with the MAS-CD\(^+\) culture are (even more) overloaded while those with a MAS-CD\(^-\) culture. Often, subordinates with the MAS-CD\(^+\) culture are (even more) overloaded while those with the MAS-CD\(^-\) culture are (even more) idling. Furthermore, subordinates with the MAS-CD\(^+\) culture, by accepting more tasks, increase the likelihood or failures in case a task is more complex than expected. In PDI-CD\(^+\) organizations, these conflicts are visible when considering leader-subordinate interactions. Leaders with MAS-CD\(^+\) cultures can order subordinates with MAS-CD\(^-\) cultures to accept multiple tasks in a row and therefore be considered as abusive (expanding the model with the notion of frustration would easily capture this feature). The other way around, subordinates with MAS-CD\(^+\) cultures can consider that leaders with MAS-CD\(^-\) cultures are careless, when they do not allocate tasks early.
Property 5: Cultural conflicts tend to negatively impact performance
Without being very specific about this point, Hofstede et al. (2010a) relate coordination conflicts with lower organizational performance (e.g. for settling down the conflict). Cultural clashes often result in coordination failures, which negatively impact performance. A quantitative investigation should display lower collective performance for organizations with agents having different cultures.
The presence of this property within our model can be verified by studying how cultural divergences impact organizational performance. Specifically, we compared organizational performance when agents share the same culture vs. have different cultures, all other aspects (environment, organizations) being equal. The number of possible combinations being too large to remain readable[4]. in this article, we have only presented simulation results that best highlight cultural conflicts. In our setting, the strongest variation is observed when both leaders and subordinates share a MAS-CD\(^-\) background and vary along the PDI-CD.
Results, presented in Figure 8(d), Figure 8(e) and Figure 8(f), mostly match expectations. In these plots, organizations with members with mismatching cultures all achieve lower performance than organizations in which members share the same culture. The only exception happens for the irregular environment with subordinates with PDI-CD\(^+\) cultures and leaders with PDI-CD\(^-\) cultures. In this setting, leaders perform quick and uninformed allocations that are blindly accepted by subordinates (if feasible). This match happens to achieve luckily excel-lent results in this very specific scenario, for which speed is far more important than accuracy or safety. However, this form of allocation is very poor in general as can be found in intensive and complex environments.
A Theory for Explaining the Influence of Culture on Societies, Based on the Influence of Culture on Individuals
Following the principles of GSSs, the current section presents the insight into the micro-to-macro influence of culture (on individual decisions and collective outcomes) that we extracted during the process of building and studying the model. While we do not claim our theory is strongly validated (though our model matches the key individual and collective cultural influences as presented by Hofstede et al. 2010a), our simulations provide an orthogonal perspective to explain the micro-to-macro phenomena. In particular, we relied on the following benefits of the GSSs methodology: (1) deeply probing organizations, which helps gathering quantitative data and observing qualitative patterns; (2) generating and comparing settings with minimal and controlled differences from one to the next; (3) studying “what if” scenarios.
Cultural expressiveness
Hofstede et al. (2010a) related the influence of culture on individuals and societies to environmental and institutional conditions. However, while highlighting specific correlations, Hofstede et al. (2010a) offer very limited insight into the general principles of this relation.
In order to further explain this relationship, we introduced the concept of cultural expressiveness. Cultural expressiveness evaluates the intersection between the amount of features that are usually visible in a culture and the amount of features that are actually visible in a given context. Cultural expressiveness aims to capture the fact that certain cultural features may or not may be expressed, depending on the context. For instance, the influence of PDI-CD\(^+\) on subordination relationships may or may not be expressed depending on the presence of a hierarchical structure in the organization. Obviously, the concept of cultural expressiveness does not cover all cases (e.g. a context might lead to visible cultural features that broadly differ from usual features of the target culture). However, cultural expressiveness provides a reasonable starting point for relating culture, the visible expression of this culture, and the environmental and institutional context. Cultural expressiveness has numerous patterns in common with gene expressiveness as theorized by Dawkins (1976): a set of background influences are carried by individuals, these influences may be visible (i.e. expressed) or silenced, due to environmental influences.
Our simulation model provides a concrete ground for explaining the links relating environment, institutions, and cultural expressiveness. In order to explore this relation, we observed both qualitatively and quantitatively the visible expressions of the influence of culture when changing the environmental, institutional and cultural context.
As a first observation, cultural expressiveness appears to be related to the degree of environmental and institutional freedom that is given to individuals. In particular, regimentation, i.e. rigid rules and systems, appears to tightly restrict cultural expressiveness. This assertion is emphasized when comparing DIT, Rule-DIT, and DIT-Together. In the stricter environment, i.e., Rule-DIT, all outcomes are very similar independently of the cultural background of the agents, as presented in Figure 7(d), Figure 7(e) and Figure 7(f). These results can be compared to the outcome in a more relaxed environment, i.e. DIT. DIT relaxes these strong rules by giving leaders the freedom to decide how they allocate tasks and by giving subordinates the freedom to accept these allocations or not. Results, presented in Figure 7(a), Figure 7(b), and Figure 7(c), indicate that organizations display a greater variation when switching from one culture to the next. Finally, these results can be compared with the outcomes of an even more relaxed environment, i.e. DIT-Together. In this organization, agents are also free to split tasks and initiate collaborative work. Again, this additional freedom appears to further increase the expression of culture by displaying a greater variability in terms of performance when switching culture, as presented in Figure 8(a), Figure 8(b) and Figure 8(c). As a general pattern, the more freedom is given to the agents, the more agents make choices in which culture can have an influence, and finally the more culture can be expressed. Note that this freedom is necessary, but not sufficient for expressing the influence of culture. It seems very likely that culture may have no visible expression in certain settings that offer wide freedom (e.g., “obvious” decisions, “human-nature” decisions, Hofstede et al. 2010a).
As a second observation, the environment and the institutional context can favour cultural expression. In addition to the requirement of being given sufficient freedom, culture tends to be best expressed in contexts that provide culturally-sensitive “affordances” (Gibson & Walker 1984) (perception anchors, which enable context-specific reasoning and action). For instance, the expression of the influence of PDI-CD is less expected to be seen without leader and subordinate organizational roles. Likewise, it would have been more difficult to observe the influence of MAS-CD without decisions involving risk-taking. This theory expands Hofstede’s theory by introducing the concept of “affordances” for cultural expressiveness. These affordances are easy to derive from the description of the cultural dimensions presented by Hofstede et al. (2010a), which provide a strong and coherent conceptual background.
Culture and interaction coherence
Without providing extensive details, Hofstede et al. (2010a) suggest that culture is beneficial for interactions (by at least, helping to understand each other). It is arguable that culture is a form of long-term social learning (Hofstede et al. 2010a, 10-11). It conveys social information such as how to behave in society, what is expected from individuals in various situations, what is important in life, etc. Conversely, misunderstandings and conflicts tend to occur more often when culture is not shared. From an individual perspective, it seems that sharing a culture helps “playing the same game”. Culture enriches actions with an additional implicit meaning. Thus, individuals sharing the same culture tend to understand better other individuals and to be better understood by others. Therefore, sharing a culture avoids unintended disagreement, even though individuals sharing a culture can be in situations in which conflicts are being raised.
In spite of these various elements, how culture influences interactions remains mysterious. Rather than considering the individual-level perspective, which is already well tackled by psychology and other social sciences, we investigated here the influence of culture on the interaction level, which, in our opinion, is best handled using the GSSs methodology.
One of the prime observations from designing and executing the model presented here lies in the strong link relating culture and interaction coherence: individuals sharing a cultural background tend to achieve more coherent interactions. Coherence is difficult to define formally, but it can be captured along multiple lines.
Very illustratively[5], coherence can be expressed in terms of Nash-Equilibria-like situations. In games where multiple equilibria are present (e.g. coordination games, as in our simulation), culture points to a given equilibrium. In some ways, culture introduces expectations about the strategies of others, therefore inciting the initial agent to match the equilibrium, as a best-response. Culture brings in coherence by providing the means for all individuals to select their best-response.
This property can be observed in our simulation by refactoring the current agent decision model with a game-theoretical framework. For instance, leaders with PDI-CD\(^+\) cultures are culturally-driven to expect that the strategy of subordinates with PDI-CD\(^+\) cultures consists of blindly accepting orders. Therefore, the best response consists of monitoring and performing informed allocations. From the perspective of subordinates with PDI-CD\(^+\) cultures, they expect leaders to perform informed allocations. Therefore, they should immediately accept any allocation and not spend time for checking its correctness. Similar reasoning can be applied for justifying PDI-CD\(^-\) MAS-CD-related decisions.
Interaction coherence can also be expressed analytically. One can argue that decisions of individuals are relatively coherent with each other, even though individuals may not reason about others when acting (e.g. following habits, matching cultural expectations). In our study, the interaction coherence raised by a culture is best observed when compared with situations in which culture is not shared.
The interaction coherence can be emphasized via a study of the prime coordination dynamics that emerge depending on the cultural background. As detailed in Property 4, in PDI-CD\(^+\) organizations leaders manage the workload of subordinates, whereas subordinates take this responsibility in PDI-CD\(^-\) organisations. In both cases, all agents act to make this interaction happen, may it be intentional or not. When PDI-CD is not shared, then the workload can be managed twice (PDI-CD\(^+\) leaders and PDI-CD\(^-\) subordinates) or none at all (PDI-CD leaders\(^-\) and PDI-CD\(^+\) subordinates). These last two interaction patterns are arguably incoherent. Similar (in)coherence can be raised regarding the MAS-CD.
A last expression of interaction coherence lies in the creation and the realization of mutually shared mutual expectations. Culture influences the expectations that individuals tend to create about themselves, others, society, and the environment. Furthermore, culturally-driven expectations, because of the shared nature of culture, tend to be assumed to be also expected by others. Furthermore, through their behaviour and not necessarily intentionally, individuals tend to make these expectations happen. In our model, PDI-CD\(^+\) agents can reasonably expect that leaders manage decisions related to allocation, whereas PDI-CD\(^-\) agents can expect that field-workers should be best placed to decide on the feasibility of an allocation. When culture is shared, then mutual expectations are met.
Culture and generic recurrent interaction patterns
As indicated in Property 1 (Section 6.4), Hofstede et al. (2010a) show that the influence of a culture within a society tends to be recurrent at the individual and collective levels. Given a culture, very similar conceptually-related phenomena tend to be observable in many places of the society (e.g. PDI-CD\(^-\) students tend to take initiatives at school and workers tend to take initiatives at work). Nevertheless, Hofstede et al. (2010a) provide only a limited insight into whether and how this recurrence is visible in interaction patterns.
As an observation from the process of constructing and exploring our model, culture seems to drive the emergence of generic and recurrent interaction patterns. This genericity can be expressed in terms of abstract inter-action patterns that tend to be instantiated when facing a concrete situation of interaction.
As an explanation, the influence of culture is both deeply social and applicable in a wide range of situations. As pointed to by cultural dimensions, and particularly by the influence of culture on values, the influence of culture tends to be conceptually consistent from one situation to the next. In other words, culture can be said to drive individual decisions towards a general common direction, pointed to by values. In this regards, because culture is shared and influential on a wide range of decisions, the indirect influence of culture on the visible interaction patterns is unsurprising. Considering available literature and our experimentation, it seems that many interactions can be captured by a few culturally-driven abstract interaction patterns.
This theory is strongly highlighted by the PDI-CD in our simulation. The PDI-CD\(^+\) interaction pattern is strongly related to centralization. Leaders are in charge of managing the interaction. They are best informed and decide for subordinates, who comply to leaders’ decisions. The PDI-CD\(^-\) interaction pattern is strongly related to distribution. Leaders are information-dispatchers and coordinators for enabling subordinates to operate on their own. Subordinates manage their own workload and task allocation. Similar patterns are observed in the DIT-Together type of organizations. In PDI-CD\(^+\) organisations, leaders manage the splitting of tasks for passive subordinates, whereas in PDI-CD\(^-\) organisations, subordinates manage to split and allocate subtasks on their own.
Explaining the micro-macro relations
This recurrence of interaction patterns can be used as an intermediate conceptual level for explaining the micro-macro link: explaining how the influence of culture on individual decisions leads to the influence of culture on collective outcomes. The global idea is that culture influences individual decisions, which influence the type of emerging interaction patterns, which then influence the global organizational performance.
This link can be observed in our simulation when trying to explain individual-to-collective links. At the individual level, PDI-CD\(^+\) cultures support a preference by both subordinates and leaders to rely on leaders to make collective decisions. These individual decisions, at the interaction level, tend to raise centralized interaction patterns. These centralized interaction patterns, at the level of collective performance, are acknowledged to achieve higher performance in simpler environments (tight management of subordinates by leaders who can grasp the whole environment) and lower robustness (see Property 1). Likewise, similar explanations can be provided to explain emerging PDI-CD\(^-\) phenomena: individuals prefer to distribute power within the organisation, leading to the “distribution” interaction pattern, which is acknowledged to perform well for handling complex environment and achieving high robustness.
Conclusion
This study relies on the Generative Social Sciences (GSSs) methodology for explaining how the known influence of culture at the collective level emerges from the known influence of culture on individual decisions. The theory proposed by this paper and based upon the GSSs methodology goes beyond the statistical correlations provided by Hofstede et al. (2010a) (i.e., relating culture and individual decisions; relating culture and collective outcomes) by proposing an integrative explanation for relating the influence of culture on individuals and the emergence of collective cultural phenomena. We presented a realistic simulation model of the influence of culture on individual decisions and collective outcomes. This model introduces the influence of culture on individual decisions that are made within an organization. Then, we validated this model by showing that its output matches the expectations provided by social sciences, both at AN individual level and through emerging collective properties. Next, through an empirical and analytical study of this model, we have proposed elaboration on available theories of the influence of culture for better capturing this influence in general and specifically its emerging properties.
Our elaboration on available theories suggests that culture supports the emergence of culturally-specific, abstract, coherent, and recurrent interaction patterns (e.g., leaders decide for subordinates), by influencing individual decisions towards a common direction, pointed to by values (e.g., obedience, social order). Coherence is promoted by a match between individual decisions (e.g., Nash-equilibria type of situations, ease for understanding each other, matching actions). These patterns are recurrent, they are repeated in numerous interaction situations. In turn, these recurrent interaction patterns support the emergence of recurrent macro-level collective phenomena, as observed in the social sciences (e.g., lower robustness). In addition to this micro-macro link, the model also made it possible to study the relatively unexplored relation linking the environment and the expression of culture.
This study shows that GSSs are particularly relevant as a complementary approach to study the influence of culture from individual decisions to collective outcomes. GSSs offer a new perspective for overcoming numerous issues faced by classic social sciences methodologies regarding the study of the micro-to-macro influence of culture. One of the core issues that simulation-based exploration helps overcoming lies in comparability: it is very difficult to find comparable situations (e.g., organisations facing very similar environments, but with different cultures). Furthermore, exploring the performance of these organizations from the individual to the collective level is very time-consuming, if even possible. Comparatively, this exercise is more feasible in a simulation setting, by changing various parameters, adding a new probe or observing step-by-step execution of the simulation. Furthermore, the GSSs approach offers a strong self-explanatory support: in multiple occasions, we had results that did not match our expectations and also discovered that our expectations were incorrect via an exploration of the simulation. More generally, GSSs provide a new approach for crafting theories, which can be validated without paying the expensive cost of collecting data in situ.
The research performed in this article opens new longer-term perspectives. The theory of the micro-to-macro influence of culture presented by this article is ready to be expanded, sharpened, and further validated. While our simulation matches general social science expectations, this theory can be strengthened by being tested against concrete real-world situations. The availability of the simulation model presented in this paper enables us to perform this step efficiently by specifying this simulation for a given application (e.g., modelling the organization of river management) and then comparing the simulation output against real-world data. This application is also beneficial for providing tools for decision-makers, for instance by helping to envision the consequences of their decisions along many aspects (e.g. how to handle a large incoming flux of migrants? Should they be sent back to their homeland? Should they be kept in a camp? Should they be incorporated within the society and how?).
Acknowledgements
The authors wish to thank Melania Borit for her continuous support in proofreading the text and her mentorship on the writing style. Likewise, the authors wish to thank Gert-Jan van der Krogt and Bruno Zanuttini for proofchecking the text.Notes
- Note that this property does not necessarily hold for any level of culture: organizational-level cultures, grown at the level of teams and organizations, are acknowledged to be correlated to organizational features, such as size and objectives (Hofstede et al. 2010a, 360-364).
- To study the relations between the organizational background and the expression of cultural influences in Section 7.2, this surprising outcome is actually purposefully sought for.
- While not explicitly integrated in the model, agents are related to cultural expectations about responsibilities of leaders and subordinates, as explained in Section 5.
- Sixteen different cultural setups can be raised just by considering all possible combinations of cultures for leaders and subordinates.
- This explanation only aims to provide Game-Theoretical insight on what coherence captures here, but is by no means a claim on how decisions are actually made. The Game-Theory formalism assumes unrealistic rational reasoning made by individuals of the form “I do \(X\) because others are expected to do \(Y\)”. Obviously, in situ, individuals are more likely to comply to the equilibrium by following habits than by seeking to optimize a utility function while reasoning about others.
Appendix: Sensitivity Analysis
As described by Augusiak et al. (2014); Ten Broeke et al. (2016); Thiele et al. (2014), sensitivity analysis is a technique for assessing whether the model replicates the global tendencies of real-world phenomena (versus matching the adequate output “by chance"). Sensitivity analysis consists in evaluating the sensitivity of the model output depending on its input, by determining how far the match between the output and real-world phenomena is preserved when facing (moderately) altered model input.
Our sensitivity analysis is based on the “One Factor at A Time" (OFAT) approach (Augusiak et al. 2014; Ten Broeke et al. 2016; Thiele et al. 2014), which matches the characteristics of the model presented in this paper (numerous input parameters and numerous experiments). The OFAT approach consists of selecting a set of relevant input parameters (i.e. factors) and of evaluating, factor per factor, how moderate alterations of this factor impact model behaviour. Moderate variations of the input parameters should lead to moderate variations of the output values of the model (or else be explainable).
We considered the following set of factors: number of subordinates (\(\mathit{\#subordinates}\)) in the group, base duration for tasks (\(\mathit{base\_duration}\)), and time before the deadline (\(\mathit{deadline}\)). Other parameters are either not part of the experiment or are a free variable for the experiment. Each factor was altered by plus and minus 10%, rounded respectively up and down, for creating wider gaps.
\(\mathit{\#subordinates}\) | \(\mathit{base\_duration}\) | \(\mathit{deadline}\) | ||||
DIT Intensive | 10 \(\rightarrow\) 9 | 0.03 | 10 \(\rightarrow\) 9 | 0.05 | 12 \(\rightarrow\) 10 | 0.14 |
10 \(\rightarrow\) 11 | 0.03 | 10 \(\rightarrow\) 11 | 0.06 | 12 \(\rightarrow\) 14 | 0.10 | |
DIT Complex | 10 \(\rightarrow\) 9 | 0.05 | 5 \(\rightarrow\) 4 | 0.02 | 15 \(\rightarrow\) 13 | 0.01 |
10 \(\rightarrow\) 11 | 0.03 | 5 \(\rightarrow\) 6 | 0.03 | 15 \(\rightarrow\) 17 | 0.01 | |
DIT Irregular | 10 \(\rightarrow\) 9 | 0.03 | 5 \(\rightarrow\) 4 | 0.02 | 15 \(\rightarrow\) 13 | 0.07 |
10 \(\rightarrow\) 11 | 0.00 | 5 \(\rightarrow\) 6 | 0.09 | 15 \(\rightarrow\) 17 | 0.04 | |
Rule-DIT Intensive | 10 \(\rightarrow\) 9 | 0.03 | 10 \(\rightarrow\) 9 | 0.06 | 12 \(\rightarrow\) 10 | 0.14 |
10 \(\rightarrow\) 11 | 0.03 | 10 \(\rightarrow\) 11 | 0.06 | 12 \(\rightarrow\) 14 | 0.10 | |
Rule-DIT Complex | 10 \(\rightarrow\) 9 | 0.04 | 5 \(\rightarrow\) 4 | 0.02 | 15 \(\rightarrow\) 13 | 0.02 |
10 \(\rightarrow\) 11 | 0.04 | 5 \(\rightarrow\) 6 | 0.02 | 15 \(\rightarrow\) 17 | 0.02 | |
Rule-DIT Irregular | 10 \(\rightarrow\) 9 | 0.02 | 5 \(\rightarrow\) 4 | 0.01 | 15 \(\rightarrow\) 13 | 0.05 |
10 \(\rightarrow\) 11 | 0.00 | 5\(\rightarrow\) 6 | 0.05 | 15 \(\rightarrow\) 17 | 0.02 | |
DIT-Together Intensive | 10 \(\rightarrow\) 9 | 0.07 | 75 \(\rightarrow\) 67 | 0.05 | 35 \(\rightarrow\) 31 | 0.07 |
10 \(\rightarrow\) 11 | 0.05 | 75 \(\rightarrow\) 83 | 0.05 | 35 \(\rightarrow\) 39 | 0.02 | |
DIT-Together Complex | 10 \(\rightarrow\) 9 | 0.06 | 50 \(\rightarrow\) 45 | 0.04 | 50 \(\rightarrow\) 45 | 0.03 |
10 \(\rightarrow\) 11 | 0.06 | 50 \(\rightarrow\) 55 | 0.04 | 50 \(\rightarrow\) 55 | 0.04 | |
DIT-Together Irregular | 10 \(\rightarrow\) 9 | 0.01 | 25 \(\rightarrow\) 22 | 0.02 | 35 \(\rightarrow\) 31 | 0.01 |
10 \(\rightarrow\) 11 | 0.01 | 25 \(\rightarrow\) 28 | 0.01 | 35 \(\rightarrow\) 39 | 0.05 | |
Multi-cultural DIT Intensive | 10 \(\rightarrow\) 9 | 0.03 | 10 \(\rightarrow\) 9 | 0.05 | 12 \(\rightarrow\) 10 | 0.14 |
10 \(\rightarrow\) 11 | 0.03 | 10 \(\rightarrow\) 11 | 0.06 | 12 \(\rightarrow\) 14 | 0.10 | |
Multi-cultural DIT Complex | 10 \(\rightarrow\) 9 | 0.04 | 5 \(\rightarrow\) 4 | 0.02 | 15 \(\rightarrow\) 13 | 0.02 |
10 \(\rightarrow\) 11 | 0.03 | 5 \(\rightarrow\) 6 | 0.02 | 15 \(\rightarrow\) 17 | 0.02 | |
Multi-cultural DIT Irregular | 10 \(\rightarrow\) 9 | 0.02 | 5 \(\rightarrow\) 4 | 0.02 | 15 \(\rightarrow\) 13 | 0.07 |
10 \(\rightarrow\) 11 | 0.00 | 5 \(\rightarrow\) 6 | 0.07 | 15 \(\rightarrow\) 17 | 0.04 |
Results, presented in Table 2 , show a high stability of the simulation patterns presented in this paper. Each cell of this table indicates the average distance between the ratio of failed tasks for the base and tilted values. A majority of these tiles show a very low distance between the input model and the tilted output (below 0.06). The largest gaps are either related to larger parameter changes (e.g. changing the base task duration of the irregular environment from \(5\) to \(6\) causes an increase of 20% of the base value) or can be easily explained (e.g. intensive environments see larger gaps when lowering the deadline, because this deadline is intentionally set low for stressing on the smooth allocation of tasks that is needed in intensive environments).
References
ANTUNES, L. & Coelho, H. (1999). Decisions Based upon Multiple Values: The BVG Agent Architecture. In P. Barahona & J. J. O. Alferes (Eds.), Progress in Artificial Intelligence: Proceedings 9th Portuguese Conference on Artificial Intelligence, Lecture Notes in Computer Science, (pp. 297–311). Springer.
AUGUSIAK, J., den Brink, P. J. & Grimm, V. (2014). Merging validation and evaluation of ecological models to ’evaluation’: a review of terminology and a practical approach. Ecological Modelling, 280, 117–128.
AXELROD, R. (1997a). The Complexity of Cooperation. Princeton, NJ: Princeton University Press.
AXELROD, R. (1997b). The Dissemination of Culture: A Model with Local Convergence and Global Polarization. Journal of Conflict Resolution, 41(2), 203–226.
BORIT, M., Vanhée, L. & Olsen, P. (2013). Theoretical Considerations for Enhancing Social Believability through Integrating Culturally Specific Trust-Building Cognitive Features in Non-Playing Characters. In Social Believability in Games Workshop, the Conference for Advances in Computer Entertainment. Twente
BORIT, M., Vanhée, L. & Olsen, P. (2014a). Towards enhancing trustworthiness of socially interactive and culture aware robots. In Culture Aware Robotics (CAR2014).
BORIT, M., Vanhée, L. & Olsen, P. (2014b). Understanding the Impact of Culture on Cognitive Trust-Building Processes: How to Increase the Social Influence of Virtual Autonomous Agents. In International Workshop on Trust in Agent Societies TRUST2014.
CONTE, R. & Paolucci, M. (2014). On agent-based modeling and computational social science. Frontiers in Psychology, 5: 668. [doi:10.3389/fpsyg.2014.00668]
CRANEFIELD, S., Winikoff, M., Dignum, V. & Dignum, F. (2017). No Pizza for You: Value-based Plan Selection in BDI Agents. IJCAI, (pp. 178–184). [doi:10.24963/ijcai.2017/26]
D’AQUINO, P., Le Page, C., Bousquet, F. & Bah, A. (2003). Using self-designed role-playing games and a multi-agent system to empower a local decision-making process for land use management: The SelfCormas experiment in Senegal. Journal of Artificial Societies and Social Simulation, 6(3), 5: https://www.jasss.org/6/3/5.html.
DAWKINS, R. (1976). The Selfish Gene. Oxford: Oxford University Press.
DEAN, J. S., Gumerman, G. J., Epstein, J. M., Axtell, R. L., Swedlund, A. C., Parker, M. T. & McCarroll, S. (2000). ‘Understanding Anasazi culture change through agent-based modeling.’ In Dynamics in human and primate societies: Agent-based modeling of social and spatial processes. Oxford: Oxford University Press, pp. 179–205.
DECHESNE, F., Tosto, G. D., Dignum, V. & Dignum, F. (2012). No smoking here: values, norms and culture in multi-agent systems. In Artificial Intelligence and Law, (pp. 79–107).
DEGENS, N., Hofstede, G.-J., Mascarenhas, Samuel Silva, A., Paiva, A., Kistler, F., André, E., Swiderska, A., Krumhuber, E., Kappas, A., Hume, C., Hall, L. & Aylett, R. (2013). Traveller - Intercultural training with intelligent agents for young adults. In Proc. 1st International Workshop on Intelligent Digital Games for Empowerment and Inclusion (IDGEI 2013) held in conjunction with the 8th Foundations of Digital Games (FDG 2013). Chania, Crete, Greece: ACM, SASDG Digital Library.
D’IRIBARNE, P. (1989). La logique de l’honneur. Gestion des entreprises et traditions nationales. Paris: Seuil.
DODDS, P. S., Watts, D. J. & Sabel, C. F. (2003). Information exchange and the robustness of organizational networks. Proceedings of the National Academy of Sciences of the United States of America, 100(21), 12516–12521. [doi:10.1073/pnas.1534702100]
ENDRASS, B., André, E., Rehm, M. & Nakano, Y. (2013). Investigating culture-related aspects of behavior for virtual characters. Autonomous Agents and MultiAgent Systems, 27(2), 277–304.
EPSTEIN, J. M. (1999). Agent-based computational models and generative social science. Complexity, 4(5), 41–60. [doi:10.1002/(SICI)1099-0526(199905/06)4:5<41::AID-CPLX9>3.0.CO;2-F]
EPSTEIN, J. M. & Axtell, R. (1996). Growing Artificial Societies. Social Science from the Bottom Up. Boston, MA: The MIT Press.
FIPA, A. C. L. (2002). FIPA ACL message structure specification. Foundation for Intelligent Physical Agents: http://www.fipa.org/specs/fipa00061/SC00061G.html.
GABORA, L. (1997). The Origin and Evolution of Culture and Creativity. Journal of Memetics, 1(1), 1–27.
GIBSON, E. J. & Walker, A. S. (1984). Development of knowledge of visual-tactual affordances of substance. Child Development, 55(2), 453–460. [doi:10.2307/1129956]
HAALAND, C. B. & Balo, I. (2017). Exploring Perceptual Distances in Norwegian-Brazilian B2B Relationships. Master’s thesis, University of Stavanger, Norway.
HAMPDEN-TURNER, C. & Trompenaars, A. (1993). The Seven Cultures of Capitalism: Value Systems for Creating Wealth in the United States, Japan, Germany, France, Britain, Sweden, and the Netherlands. New York, NY: Currency Doubleday.
HOFSTEDE, G., Dignum, F., Prada, R., Student, J. & Vanhée, L. (2014). Gender difference: the role of nature, nurture, social identity and self-organization. In The 15th International Workshop on Multi-Agent-Based Simulation MABS2014. Paris, France.
HOFSTEDE, G., Hofstede, G. J. & Minkov, M. (2010a). Cultures and Organizations: Software of the Mind, Third Edition. New York, NY: McGraw-Hill Professional.
HOFSTEDE, G. J., Jonker, C. M. & Verwaart, T. (2010b). Cultural Differentiation of Negotiating Agents. Group Decision and Negotiation, 21(1), 79–98.
KROEBER, A. L. & Kluckhohn, C. (1952). Culture: A critical review of concepts and definitions. Papers. Peabody Museum of Archaeology & Ethnology, Harvard University, 47(1).VIII, 223.
MC BREEN, J., Di Tosto, G., Dignum, F. & Hofstede, G. J. (2011). Linking Norms and Culture. 2011 Second International Conference on Culture and Computing, (pp. 9–14).
MICELI, M. & Castelfranchi, C. (1989). A Cognitive Approach to Values. Journal for the Theory of Social Behaviour, 19(2), 169–193. [doi:10.1111/j.1468-5914.1989.tb00143.x]
MINTZBERG, H. (1980). Structure in 5’s: A Synthesis of the Research on Organization Design. Management Science, 26(3), 322–341.
MINTZBERG, H. & Heyden, L. V. D. (1999). Organigraphs: Drawing How Companies Really Work. Harvard Business Review, 77, 87-94.
NAKATA, C. (2009). Beyond Hofstede. Culture Frameworks for Global Marketing and Management. London, UK: Palgrave Macmillan.
SCHRAMM-NIELSEN, J. (2001). Cultural dimensions of decision making: Denmark and France compared. Journal of Managerial Psychology, 16(6), 404–423. [doi:10.1108/02683940110402389]
SCHWARTZ, S. (1999). A Theory of Cultural Values and Some Implications for Work. Applied Psychology, 48(1), 23.
SCHWARTZ, S. H. (1994). Beyond Individualism/Collectivism: New Cultural Dimensions of Values. London: Sage Publications.
SCHWARTZ, S. (2006a). An Overview Basic Human Values: Theory, Methods, and Applications Introduction to the Values Theory. Jerusalem Hebrew University.
SCHWARTZ, S. H. (2006b). A Theory of Cultural Value Orientations: Explication and Applications. Comparative Sociology, 5(2), 137–182.
SCHWARTZ, S. H. (2006c). Basic Human Values: An Overview Basic Human Values : Theory, Methods and Applications Introduction to the Values Theory. Jerusalem Hebrew University, 48, 49–71.
SMITH, P. B., Peterson, M. F. & Schwartz, S. H. (2002). Cultural values, sources of guidance, and their relevance to managerial behavior: A 47-nation study. Journal of Cross-Cultural Psychology, 33(2), 188–208. [doi:10.1177/0022022102033002005]
TEN BROEKE, G., Van Voorn, G. & Ligtenberg, A. (2016). Which sensitivity analysis method should I use for my agent-based model? Journal of Artificial Societies and Social Simulation, 19(1), 5: https://www.jasss.org/19/1/5.html. [doi:10.18564/jasss.2857]
THIELE, J. C., Kurth, W. & Grimm, V. (2014). Facilitating parameter estimation and sensitivity analysis of agent-based models: A cookbook using NetLogo and R. Journal of Artificial Societies and Social Simulation, 17(3), 11: https://www.jasss.org/17/3/11.html. [doi:10.18564/jasss.2503]
VANHÉE, L. (2015). Using Culture and Values to Support Flexible Coordination. Ph.D. thesis, Utrecht Universiteit.
VANHÉE, L., Dignum, F. & Ferber, J. (2013). Towards Simulating the Impact of National Culture on Organizations. In MABS2013: 14th International Workshop on Multi-Agent-Based Simulation, (p. 12). Saint Paul.
VANHÉE, L., Dignum, F. & Ferber, J. (2014). Modeling Culturally-Influenced Decisions. In MABS2014:15th International Workshop on Multi-Agent-Based Simulation. Paris, France.
VÁZQUEZ-SALCEDA, J., Aldewereld, H. & Dignum, F. (2005). Norms in Multiagent Systems: From Theory to Practice. International Journal of Computer Systems Science & Engineering, 20(4), 225–236.