Introduction
Agrobiodiversity plays an important role in African agricultural systems (Khumalo et al. 2012; McCord et al. 2015; Wale 2008). In the Sahel, farmers use crop varietal diversity to cope with the variability of environmental constraints, such as climate variability and change, degradation of soil fertility (McCord et al. 2015; Soumare et al. 2008), to cope with risks of crop failure (Cavatassi et al. 2010; Siart et al. 2008), market access and multiple uses (food, market, cultural and traditional uses) (Bazile et al. 2008; Coulibaly et al. 2008).
In Malian agricultural systems, sorghum (Sorghum bicolor) is one of the most important food crops, representing 35% of dry cereal production, and varietal diversity of sorghum plays an important role (Coulibaly 2011; Vaksmann et al. 2008). The high stability of consumption from the 1980s to date confers on sorghum, together with pearl millet (Pennisetum glaucum) the lead place with more than 55% of the cereal diet in 2011, ahead of rice (26%) and maize (15%). Despite its importance, the crop varietal diversity of sorghum is declining in Mali due to the development of maize and rainfed rice production, which are progressively replacing sorghum in southern Mali, where farmers have access to agricultural inputs associated with cotton production (Coulibaly 2011).
Recently, the Malian government has implemented various measures favoring the dissemination of improved (modern) sorghum. However, the adoption rate of the modern varieties is still low at 5% (Matlon 1990). Policy makers use informal and formal seed networks, as well as organizational and media communication strategies aimed at informing and influencing individual and collective decisions. The main challenges for policy makers when trying to increase adoption of improved varieties are 1) how to determine the best strategies of variety diffusion through complex social networks, and 2) how to improve the adoption of improved varieties while maintaining local varietal biodiversity.
Developing policies for improved varieties introduction is a difficult task. When assessing or supporting agrobiodiversity conservation and use in developing countries, one must consider seed systems, part of the agricultural system which encompasses complex interactions among social, economic and biophysical dynamics. In addition, implementation policies and the adoption of new strategies take more time.
All this calls for a relevant tool to address the complexity of crop varietal management and ex-ante analysis of the impacts of policies. Modeling is one such relevant tool.
Modeling has previously been applied in the assessment of crop varietal diversity management by farmers (Dusen et al. 2007; He and Cai 2016). However, the existing works are site-specific or focused on specific crops (Abrami et al. 2008; Bazile and Abrami 2008) and do not represent the interactions between the different scales of an agricultural system: plot, farm and system levels.
Furthermore, they do not integrate the social, economic, environmental and policy dimensions of crop varietal diversity management. Crop genetic resources are a renewable resource, the dynamics of which depends on the interactions between socio-economic and environmental dynamics and the policy interventions. Consequently, assessment methods used to analyze the sustainability of crop varietal diversity management should integrate environmental, social, economic and policy objectives. Moreover, most previous models have not integrated both the knowledge from scientists and from social actors. Considering both the scientists’ and local farmer’s knowledge in the design of agrobiodiversity management models is necessary, as in developing countries, agrobiodiversity integrates the management of modern varieties based on scientific knowledge and of landraces based on local knowledge. Varieties are even developed through participatory breeding strategies integrating farmers’ and scientists’ knowledge as well as exotic and landrace genetic materials (Sissoko et al. 2008; vom Brocke et al. 2010). Based on the ARDI approach, Coulibaly et al. (2014) proposed a conceptual model to examine how interconnections between seed networks and the global system impact the dissemination of modern varieties. However, the authors were not interested in the simulation of the dynamics of these interconnections and their long-term impacts.
The objective of this research is to develop a new modeling approach for simulating crop varietal diversity management by farmers. Specifically, the study seeks to:
- represent crop varietal diversity management at the plot, farm and territory level while taking account the social, economic, environmental and policy dimension;
- integrate scientists’ and local stakeholders’ knowledge in the modeling process;
- propose a generic model;
- explore the impacts of climate scenarios, modern variety adoption and social networks on crop varietal diversity.
The structure of the paper is organized as follows. In the next section, the participatory approach, the stakeholders’ engagement, the modeling approach and the study sites are presented. Finally, the results of the simulations are presented and discussed.
Materials and Methods
Crop varietal diversity management, a complex system
The farmer seed network in Mali is based on the self-production of pearl millet and sorghum seeds and operates through non-commercial, community-based exchanges. The formal seed sector distributes certified seeds through cooperatives, with cost-effectiveness being the main priority. The joining of these two seed networks could allow agrobiodiversity to be considered in such a way that genetic diversity can be maintained. The various pearl millet and sorghum seed exchange networks often are considered to be in opposition, with the formal network pitted against the informal one (Coulibaly et al. 2014).
Seed networks are complex systems. They are characterized by a range of actors (farmers, policy makers, non-governmental organizations, researchers, etc.) interacting through their social and institutional networks for knowledge and seed sharing. These complex interactions, agriculture’s multifunctional activities, and the fact that crop genetic resources can be considered as common community resources, all call for a systems approach. "The systems approach can, among other things, be used to assess the effect of interactions between farms on the overall environmental impact of the farming region" (Payraudeau and van der Werf 2005).
Most relevant among systems approaches, the agent-based model (ABM) allows the heterogeneity of actors’ decision making, the multiplicity of their points of view (Papazian et al. 2017), the effect of a social network on individuals’ behavior (Haer et al. 2016; Lakon et al. 2015), feedbacks loops between social and biophysical dynamics and the natural dynamics of the environment (Belem et al. 2011; Bousquet and Le Page 2004; Drogoul et al. 2016), and finally, the impacts of policy interventions on individual behavior (d’Aquino et al. 2014; Saqalli et al. 2011) to be taken into account.
Thus, we rely on an ABM to represent the dynamics of crop varietal diversity management. A generic model named "Simulation de l’Impact des Modalités d’Accès aux Semences" (SIMAS) was developed.
This study used innovation diffusion theory (Rogers 1995) to represent the diffusion of crop varieties through social networks. Innovation diffusion theory distinguishes five categories of adopters: innovators, early adopters, early majority, late majority, and laggards. This study took account three categories of adopters: innovators, adopters and conservators (laggards).
In the model, farmers select (change) crop varieties depending on the information exchange on crop varieties in their social networks and their criteria. To implement varietal selection in the model, we used decision rules based on the concepts of bounded rationality (Simon 1955). Bounded rationality is based on the assumption that an agent seeks satisfactory solutions rather than optimal ones. Specifically, the “take-the-best” heuristic (Gigerenzer and Goldstein 1996) was used to implement the farmers’ decision rules for a varietal choice.
Modeling procedure
This study used participatory modeling (Aubert et al. 2010) to integrate knowledge from researchers, farmers and policy makers. The participatory modeling process used in this study involved (1) researchers from different disciplines: agronomists, geneticists, geographers, socio-economists, plant breeders, and modelers; and (2) representatives from non-governmental organizations (NGOs), private enterprises, and farmers’ organizations (Table 1).
Step | 1 | 2 3 4 5 | 6 | 7 | 8 |
Analysis | Conceptualization | Implementation | Validation | Experimentation | |
Participants | Modelers | Researchers, non-government organization (NGO), government organization (GO), farmers’ organization, a facilitator, a modeler | Researchers, modelers, GO, NGO, a facilitator, a modeler | Researchers, Farmers, NGO, GO, a facilitator, a modeler | Modelers |
Mali | Workshop 1 (4 days) | Workshop 2 (1 day) | Workshop 3 (1 day) |
To obtain an exhaustive representation for modeling purposes and a general description of the seed systems in Mali, the description concerns several regions of agricultural production.
The study sites, from the northeast to the southwest of Mali, are: Tassiga in Gao Region[1], Petaka in Mopti Region, Kanian in Ségou Region and, Kaniko and Siramana in Sikasso Region (Table 1).
The modeling process was conducted through three workshops and followed eight modeling steps: conceptualization (four steps), scenario building (one step), implementation (one step), validation (one step), and experimentation (one step) (Table 1).
The detailed description of the modeling procedure, the data collection and the model are given in the Supplementary material.
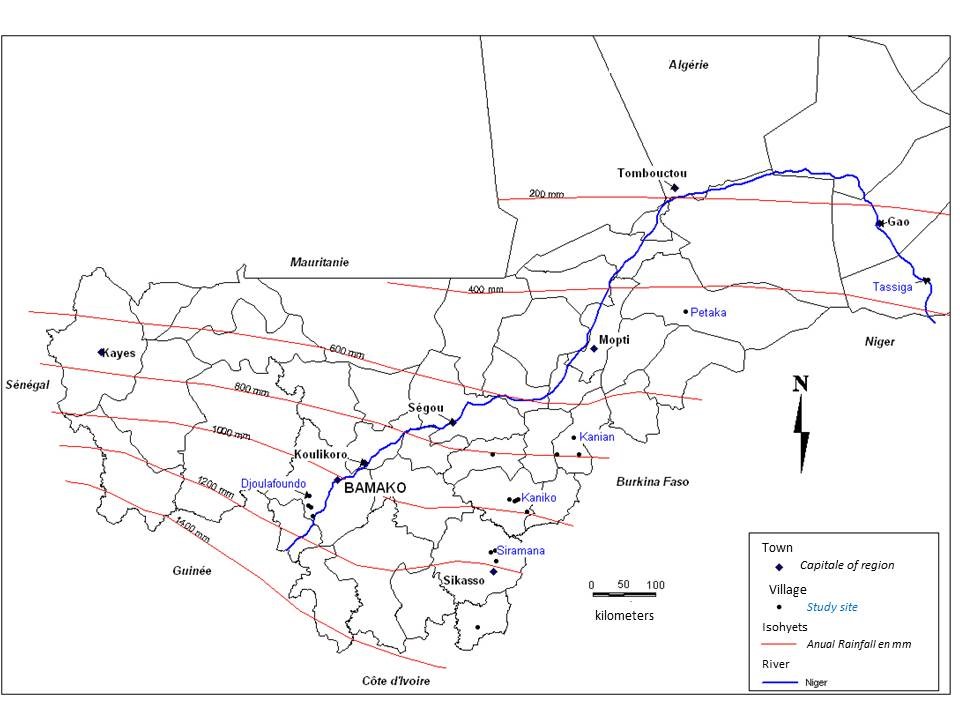
Model conceptualization
The objectives of this step were to develop with the stakeholders a common understanding of the targeted problem and a common conceptual model that represents crop varietal management in Mali. To achieve these, a first workshop was organized. During the first day of the workshop, participants identified and delimited the study domain. The objective was to identify different actors’, perceptions and the domain of the study, to define the main functions (processes) to integrate, and to identify main actors in seed system, as well as the socio-professional networks through which the actors interact.
During the second day of the workshop, participants collectively characterized the different actors, their roles in agrobiodiversity, the resources they use, and interactions between them. In addition, the main traits of varieties in which farmers are interested were identified. The third day of the first workshop, participants identified four main scenarios illustrating climate and policy interventions for introduction of improved sorghum varieties.
During the fourth day, modelers developed a generic conceptual model using Unified Modeling Language(ULM). The complete description of the conceptual model used the results from the previous steps, as well as results of a range of studies on crop varietal diversity management modeling (Abrami et al. 2008; Bazile and Abrami 2008; Vaksmann et al. 2008) and surveys in the different regions of Mali for collecting data on farm socio-economic characteristics (farm size, labor use, family size, the socio-professional network, source of seed, production use, etc.), their preferences concerning different varieties and their management strategies (Alfonso 2010; Bazile and Soumaré 2004; Coulibaly et al. 2008). Finally, the fifth day of the first workshop, the participants validated the conceptual model.
Model implementation and validation
In the sixth step of the modeling process, the modelers implemented a generic ABM using the Mimosa platform (Müller 2004). After the model implementation, a second workshop was organized to present the model to participants and to discuss the structure of the model, input and output data, and some preliminary results from first simulations. In a third workshop, participants undertook to validate the ABM using role playing games (RPGs) that assessed the model’s structure and dynamics: objects, spatial and temporal scales, actors and their decisions, and the system dynamics.
Experimentation
As a final step, we used the ABM to simulate the scenarios identified in the first workshop with a focus on sorghum varietal diversity management.
The simulations conducted for this paper were based on the configuration of a virtual village corresponding to southern Malian conditions including 800 mm of precipitation and a 6-month rainy season. The objective of the simulations was to assess the impacts of policy and climate on the adoption of two modern varieties: Wassa and Grinka (see the supplementary material on data collection).
The simulated population was composed of 37 farmers divided into three groups: small, medium and large. Initially, all farmers cultivated all three local varieties of sorghum – Kalosabani, Seguetana and Tiemarifing – as well as one variety of maize. In these simulations, farmers were assumed to have crop yield as the major criterion for varietal selection. Although this is not realistic, it facilitated the assessment of the systems dynamics and the comparison of the farmers’ strategies.
Two set of scenarios were simulated. In the first set, the impacts of policy intervention on the introduction of the improved varieties were simulated under four climatic regimes (Figure 2). The four climatic regimes were defined to have different lengths of growing season and beginning dates of the rainy season. In these simulations, we assumed that the climate was variable. We call these simulations "variable climate simulations" (VCS).
Climate regimes | |||||
Beginning of season | Precipitation | ||||
Climate regimes | Description | Average (Day of year) | Std deviation | Average (mm) | Std deviation |
C1 | Normal precipitation at beginning of season | 165 | 15 | 800 | 100 |
C2 | Normal precipitation with late beginning of season | 165 | 45 | 800 | 100 |
C3 | Low precipitation with normal beginning of season | 165 | 15 | 800 | 400 |
C4 | Poor season with late beginning of season | 165 | 45 | 800 | 400 |
Policy interventions | |||||
Interventions | Description | ||||
P1 | Donation of fertilizers and equipment to all farmers | ||||
P2 | Donation of fertilizers and equipment only to farmers that cultivate Wassa and Grinkan varieties. |
In the second set of scenarios, we assumed that the climate is static. Then, we set nine possible climate configurations between rainy season length (short, medium, long) and precipitation level (low, medium, good) (Table 3). The nine possible climate configurations were combined with the two policy interventions (general agricultural versus variety-specific) to generate a total of 18 scenarios. We call these simulations "stable climate simulations" (SCS). In total, 26 scenarios have been simulated. Given that there is some stochasticity in agent decision making, each scenario was simulated 100 times and the mean values of the simulation calculated and described in this paper.
Climate scenario | Season duration | Rainfall |
S1 | Long | Low |
S2 | Long | Medium |
S3 | Long | Good |
S4 | Medium | Low |
S5 | Medium | Medium |
S6 | Medium | Good |
S7 | Short | Low |
S8 | Short | Medium |
S9 | Short | Good |
Description of the model
The SIMAS model is developed to provide a decision support tool to assess the impacts of climate change and variability and policies on crop varietal diversity at village territory level. The model provides a decision support tool to assess how the policy support could contribute to the adoption of modern varieties in the context of climate variability and change.
SIMAS is based on the multi-faceted simulation of agrobiodiversity by taking account the social, economic, environmental and policy dimensions and the interactions at different scales (plot, farm and village levels). For the social dimension, the model integrates the heterogeneity of farmers’ practices and behaviors for individual or collective crop varietal diversity and crop production management. The model also integrates the dynamics of social networks, food security issues, the importance of formal and informal seed exchanges, and seed varietal diffusion of one or several crop species through specific networks. For the economic dimension, the model accounts for income generation, availability of labor at farm level, and input access. The model’s environmental dimension principally concerns climate through the variability of annual precipitation, length of the rainy season, and crop yield responses to soil diversity. For the policy dimension, the model simulates the impacts of policy support on crop varietal diversity, poverty reduction, access to inputs and varietal diffusion.
The structure of the model (Figure 2) is based on two types of agents – Organization and Farmer – and different types of objects representing the agents’ knowledge, farmers’ preferences, crop species and varieties, and agricultural interventions. An organization is both an agent and a group of farmers interacting to pursue the same goals. An organization can represent a GO, NGO, a village, a research organization, an enterprise or a farmers’ organization . The farmer is the main agent of the model. Farmers’ type determines their characteristics. A farmer is a social individual interacting with other farmers through a socio-professional network that determines seed access. Farmer can belong simultaneously to several socio-professional networks and interact only with the farmers of the same socio-professional networks.
The dynamics of the model integrated the following modules:
- The climate module: computes the climate variability based on the determination of beginning and end of rainy seasons and the precipitation.
- Crop production: simulates the farmers’ decision making for crop production management. The crop production depends on the climate since the crop yields depend on the precipitation and the beginning and end of the rainy season.
- Farm decision module for varietal management: simulates the crop varietal diversity management by the farmers. Specifically, it represents how farmers seek seeds, share and get information about varieties, and manage crop varietal diversity using different innovation strategies.
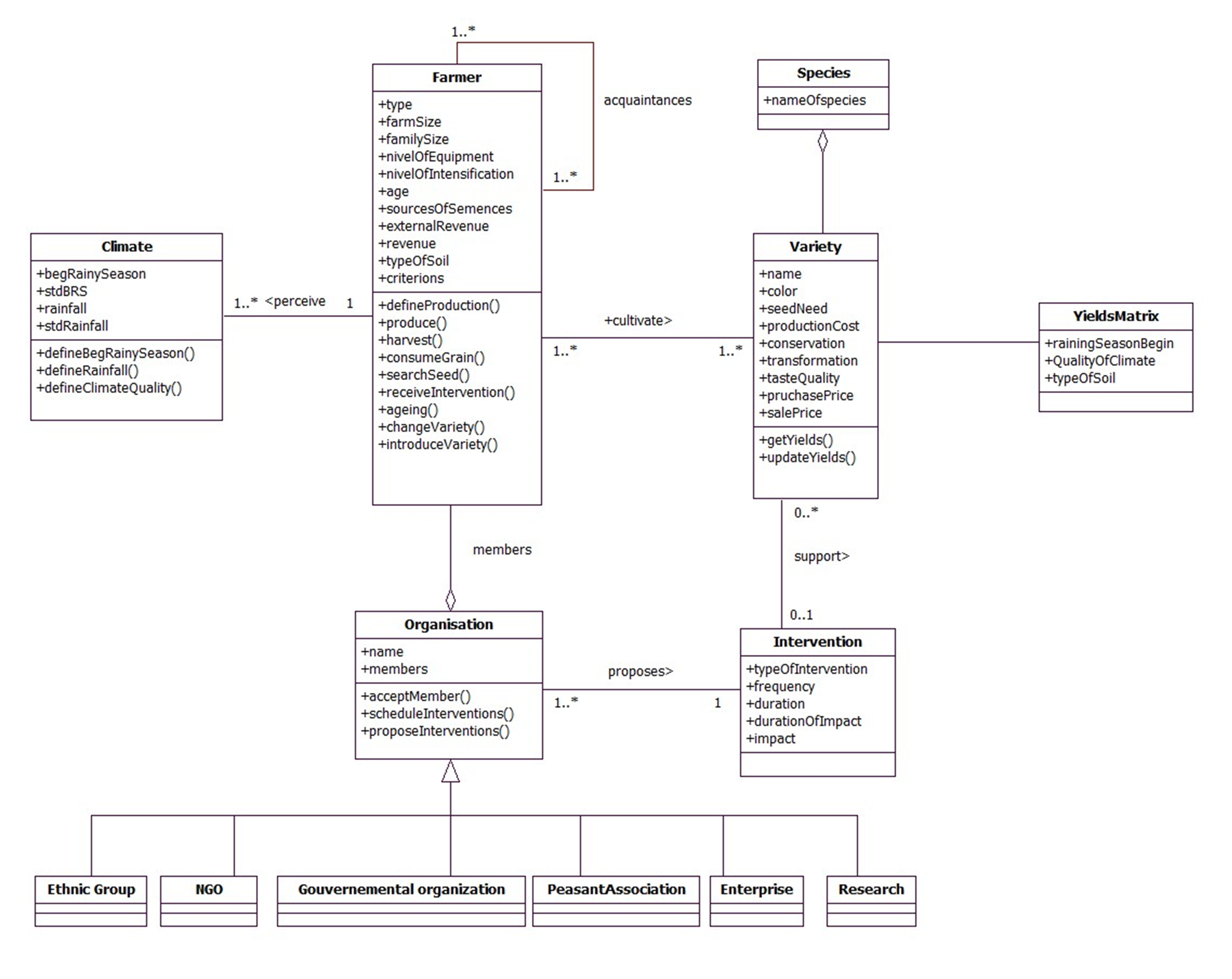
Results
Impacts of policy interventions and climate variability on variety adoption
The simulations for variable climate simulations showed how policy interventions affected crop varietal diversity and farmers’ adoption of the improved Wassa and Grinkan varieties. P1, the general agricultural intervention, favors the adoption of Wassa and Grinkan over P2 (Figure 3).
Though the production costs of Wassa and Grinkan are higher than those of Kolasabani, Seguetana and Tiemarifing, their potential yields are also higher, and when farmers have the necessary resources (related to the intensification level) to cultivate the new varieties, they will introduce them. By targeting everyone, the general agricultural intervention increases all farmers’ intensification levels and thus increases the willingness of all farmers to adopt the new greater potential varieties. However, the variety-specific intervention increases the intensification level for only farmers cultivating the supported varieties, thus limiting the adoption of the new varieties by the large farmers.
Impact of climate on varietal distribution
The simulations showed that the portfolio of sorghum varieties each farmer cultivates depends on the climatic conditions. We observed that the portfolio of sorghum varieties is higher in the S2, S3, S5 and S6 climate configurations than in the other climate configurations (Figure 4). In the S2, S3, S5 and S6 climate configurations, the seasonal duration is medium or long and the precipitation is medium or good. These climate configurations allow higher crop yields as they cover the production cycle of all varieties and provide more water for crop production. In this situation, farmers are willing to introduce new varieties and to increase the portfolio of varieties.
The yields of modern varieties generally fluctuate widely depending on the climatic variance, are considered as an important risk factor in low-input farming systems and lead farmers to use improved varieties only when the risk of crop failure is low, i.e., only when the climatic condition is good. In poor climatic conditions, farmers prefer local varieties (Figure 2) that produce more consistent, less climate-dependent yields and they are not willing to adopt new varieties or to enlarge their portfolios due to the increased risk of production failure (Bellon, 2006; Cavatassi et al. 2010; Niangado 2001; Traoré et al. 2007). That explains why the adoption of Wassa and Grinkan is greater under stable and good climate configurations (S2, S3, S5 and S6) than in variable and poor climate configurations (Figure 3, 5 and Figure 6).
Relationships between land use and crop varietal management
The simulations showed that the climate impacts land allocation. In variable climate simulations, we observed that land allocation is variable for all varieties (Figure 7) contrary to the stable climate simulations, where the land allocation is more or less stable (Figure 8 and Figure 9). Because the different varieties have different yields under different climate configurations, farmers frequently change their varieties to obtain higher yields as the climate changes. This means that farmers likewise frequently change their allocation of land by planting preferred varieties in the largest areas, which could explain why land allocation varies in direct relation to the variability of the climate. Thus, in short and medium seasons, farmers allocated the largest areas to short-cycle varieties. In short season and medium rainfall, the area allocated to Kalosabani, a short-cycle variety, is larger than those of the other sorghum varieties (Figure 8). In long seasons with medium rainfall, the areas allocated to Grinka, Wassa and Tiemarafing – modern and long-cycle varieties – are larger than those of Kalosabani and Seguetana – local and short and medium-cycle varieties (Figure 9). In long seasons with low rainfall, the areas allocated to Seguetana, Tiemarifing and Kalosabani – local race varieties – are larger than those of Wassa and Grinkan – modern varieties (Figure 9).
Discussion
Stakeholders’ involvement
Voinov et al. (2016) identified seven components of participatory modeling. Our modeling process involved stakeholders in the main components of the participatory modeling (Table 4). In the current study, stakeholders were involved in the study delimitation, the development of the conceptual model and of the ABM. The scenarios used in the simulations were formulated by the stakeholders. Finally, the RPGs enabled validation of the model structure based on the stakeholders’ point of view.
The stakeholders involved in this study were the researchers, policy makers, farmers and other institutions. This is important because, “while the participants may determine the questions that the model should answer and may supply key model parameters, and processes, the structure of the model must be scientifically sound and defensible” (Voinov and Bousquet 2010).
PM component | Stakeholders engagement |
Scoping & abstraction: | The objective of the modeling process was identified with the stakeholders who delimited the domain and the site of the study. |
Envisioning & goal-setting | The conceptual model was developed with and by the stakeholders based on their respective point of views. |
Model formulation | The ABM was developed by the modelers. However, the simulated scenarios and related indicators were identified by the stakeholders. The model was validated based on stakeholders’ points of view through RPGs. |
Collection of original data and cross-checking of expert data | The data were collected by the scientists, completed during the RPG, with the review of previous works and based on expert knowledge. |
Apply model to decision making | Outside the case study the model is not applied to decision making |
Evaluation of outputs | The RPGs and presentation of the model outputs allowed stakeholders to discuss the model structure, outputs and to contribute to its evaluation. |
Genericity of the model
The main contribution of this paper is to develop a generic ABM of crop varietal management integrating knowledge from both social actors and scientists.
Generic models aim to represent a range of systems in a domain of interest and to provide a general explanation of the problem to be resolved. This allows researchers and modelers to interact in order to improve the modeling process and knowledge concerning the domain of interest. Thus, model becomes more than a simple tool; it becomes a support to research since " … by recognizing certain classes of processes, modeling efforts would be greatly reduced and researchers would begin to see the unique and different as exceptions, rather than the rule itself" (Innis et al. according to Reynolds and Acock 1997). In addition, “the time required in the simulation study should be reduced because the model need only be populated with data from various systems and not constructed from scratch" (Steele et al. 2002).
As shown in the methodology, SIMAS concerned a range of regions in Mali. However, the model is not specific to Mali but is generic. In addition, the model was developed to simulate the management of a range of crop varietal diversity depending on the interests of the user. The conceptual model based on stakeholders’ perspectives and a range of regions is very detailed to provide a detailed and comprehensive description of agrobiodiversity management by the farmers and to permit the use of the model in different areas.
Consequently, our model is a relevant support for knowledge sharing and integration between various stakeholders and from different sources concerning agrobiodiversity management.
Conclusion
This study concerned the simulation of climate change and policy interventions impacts on agrobiodiversity in Mali with a focus on sorghum crop production. Simulations showed that farmers manage crop varietal diversity to cope with climate variability for annual crop production. The study found that climate variability impacts the amount of land allocated to different crop varieties, the number of crop varieties in a farmer’s portfolio, and the farmer’s adoption of improved varieties.
Supporting both crop varietal diversity and the adoption of improved varieties requires decreasing the negative environmental impacts (climate variability for instance), which can be achieved by improving farmers’ production capacity, first through agricultural interventions directed toward all farmers, and not focused only on farmers adopting improved varieties; and second, by developing high yielding, but less climate-dependent varieties.
For future research, the model will be improved to integrate explicit soil and crop models. In addition, the model will be improved by integrating the soil spatial heterogeneity at farm level as well as landscape heterogeneity to consider pest and disease management.
The social networks in the model are static, which does not allow an explicit analysis of the impact of social networks dynamics on crop varietal dynamics, and improvements to the model should integrate networks’ dynamics. Finally, the model will be extended to larger spatial scales such as regions by considering spatial variations in climate and environment.
Acknowledgements
The authors thank all of the IMAS project (ANR-AAP Biodiversity) staff with special thanks to François Bousquet and Jean-Pierre Müller of the Agricultural Research Centre for International Development (CIRAD, France), and all of the farmers, researchers and stakeholders who took part in the various modeling sessions in Mali and France. In addition, the authors thank also Karen Greenough from WASCAL for her helpful comments and improvement of the revised manuscript. Finally, the authors thank the anonymous reviewers for their useful comments.Notes
- Region is the first administrative division of the country.
Appendix
Supplementary materials, including the ODD description of the SIMAS model, can be found in a separate file by clicking here.References
ABRAMI, G., Bazile, D., Trebuil, G., Le Page, C., Bousquet, F., Dionnet, M., & Vejpas, C. (2008). Accompagner l’évolution des systèmes semenciers céréaliers au Mali et en Thaïlande. Cahiers Agricultures, 17(2), 210-215.
ALFONSO Becares, D. (2010). Gestion paysanne de l’agrobiodiversité. Le cas du mil et du sorgho au Mali. CIHEAM: Institut Agronomique Méditerranéen de Montpellier., Montpellier, p. 103 p. + annexes.
AUBERT, S., Müller, J.P., & Ralihalizara, J., (2010). 'MIRANA: A socio-ecological model for assessing sustainability of community-based regulations.' In David A. Swayne, W.Y., Alexey A. Voinov, Andrea Rizzoli, Tatiana Filatova (Ed.), iEMSs Firth Biennial Meeting: International Congress on Environmental Modelling and Software (iEMSs 2010), Ottawa, Canada.
BAZILE, D., & Abrami, G., (2008). Des modèles pour analyser ensemble les dynamiques variétales du sorgho dans un village malien. Cahiers Agricultures, 17(2), 203-209.
BAZILE, D., Dembélé, S., Soumare, M., & Dembélé, D. (2008). Utilisation de la diversité variétale du sorgho pour valoriser la diversité des sols au Mali Cahiers Agricultures, 17(2), 86-94.
BAZILE, D., & Soumaré, M. (2004). Gestion spatiale de la diversité variétale en réponse à la diversité écosystémique : le cas du sorgho au Mali. Cahiers Agricultures, 13(6), 480-487.
BELEM, M., Manlay, R.J., Müller, J.P., & Chotte, J.-L. (2011). CaTMAS: A multi-agents model for the simulation of carbon resources dynamics of West African villages. Ecological Modelling, 222(20-22), 3651-3661. [doi:10.1016/j.ecolmodel.2011.08.024 ]
BELLON, M.R. (2006). Crop research to benefit poor farmers in marginal areas of the developing world: A review of technical challenges and tools. CAB Reviews: Perspectives in Agriculture, Veterinary Science, Nutrition and Natural Resources, 1(70), 1-11.
BOUSQUET, F., & Le Page, C. (2004). Multi-agent simulations and ecosystem management: A review. Ecological Modelling, 176(3-4), 313-332. [doi:10.1016/j.ecolmodel.2004.01.011 ]
CAVATASSI, R., Lipper, L., & Narloch, U. (2010). Modern variety adoption and risk management in drought prone areas: insights from the sorghum farmers of eastern Ethiopia. Agricultural Economics, 42(3), 279–292.
COULIBALY, H. (2011). Rôle des organisations paysannes dans la diffusion des semences de céréales: articulations des réseaux semenciers étatiques et traditionnels paysans pour une conservation in situ des variétés, Ecole Doctoral Milieux, Cultures et Sociétés du Passé: Composante Espace et Société. Université de Paris Ouest Nanterre La Défense.
COULIBALY, H., Bazile, D., & Sidibé, A. (2014). Modeling Seed System Networks in Mali to Improve Farmer Seed Supply. Sustainable Agriculture Research, 3(4), 18-32.
COULIBALY, H., Bazile, D., Sidibé, A., & Abrami, G. (2008). Les systèmes d’approvisionnement en semences de mils et sorghos au Mali: production, diffusion et conservation des variétés en milieu paysan. Cahiers Agricultures, 17(2), 199-202.
D’AQUINO, P., Bah, A., & Turner, B.L. (2014). Multi-level participatory design of land use policies in African drylands: A method to embed adaptability skills of drylands societies in a policy framework. Journal of Environmental Management, 132, 207-219.
DROGOUL, A., Huynh, N.Q., & Truong, Q.C. (2016). Coupling Environmental, Social and Economic Models to Understand Land-Use Change Dynamics in the Mekong Delta. Frontiers in Environmental Science, 4, 19. [doi:10.3389/fenvs.2016.00019 ]
DUSEN, E.V., Gauchan, D., & Smale, M. (2007). On-Farm Conservation of Rice Biodiversity in Nepal: A Simultaneous Estimation Approach. Journal of Agricultural Economics, 58(2), 242–259.
GIGERENZER, G., & Goldstein, D.G. (1996). Reasoning the fast and frugal way: Models of bounded rationality. Psychological Review, 103(4), 650-669. [doi:10.1037/0033-295X.103.4.650 ]
HAER, T., Botzen, W.J.W., & Aerts, J.C.J.H. (2016). The effectiveness of flood risk communication strategies and the influence of social networks—Insights from an agent-based model. Environmental Science & Policy, 60, 44-52. [doi:10.1016/j.envsci.2016.03.006 ]
HE, Y.-b., & Cai, W.-M. (2016). Linking a farmer crop selection model (FCS) with an agronomic model (EPIC) to simulate cropping pattern in Northeast China. Journal of Integrative Agriculture, 15, 2417-2425.
KHUMALO, S., Chirwa, P.W., Moyo, B.H., & Syampungani, S. (2012). The status of agrobiodiversity management and conservation in major agroecosystems of Southern Africa. Agriculture, Ecosystems & Environment, 157, 17-23. [doi:10.1016/j.agee.2012.01.028 ]
LAKON, C.M., Hipp, J.R., Wang, C., Butts, C.T., & Jose, R. (2015). Simulating Dynamic Network Models and Adolescent Smoking: The Impact of Varying Peer Influence and Peer Selection. American Journal of Public Health, 105(12), 2438-2448.
MATLON, P.J. (1990). Improving productivity in sorghum and pearl millet in semi-arid Africa. Food Research Institute Studies, 22(1). 1-43.
MCCORD, P.F., Cox, M., Schmit-Harsh, M., & Evans, T. (2015). Crop diversification as a smallholder livelihood strategy within semi-arid agriculture systems near Mount Kenya. Land Use Policy, 42, 738-750.
MÜLLER, J.P. (2004). The Mimosa generic modelling and simulation platform, ABS’04, Lisbon.
NIANGADO, O. (2001). 'The state of millet diversity and its use in West Africa.' In Cooper, H.D., Hodgkin, T. (eds.), Broadening the Genetic Base of Crop Production. CABI Publishing, Wallingford.
PAPAZIAN, H., Bousquet, F., Antona, M., & d’Quino, P. (2017). A stakeholder-oriented framework to consider the plurality of land policy integration in Sahel. Ecological Economics, 132, 155-168. [doi:10.1016/j.ecolecon.2016.10.020 ]
PAYRAUDEAU, S., & van der Werf, H.M.G. (2005). Environmental impact assessment for a farming region: a review of methods. Agriculture, Ecosystems & Environment, 107, 1-19.
REYNOLDS, J.F., & Acock, B. (1997). Modularity and genericness in plant and ecosystem models. Ecological Modelling, 94(1), 7-,16. [doi:10.1016/S0304-3800(96)01924-2 ]
ROGERS, E. (1995). Diffusion of Innovations. NY: Free Press., New York.
SAQALLI, M., Gérard, B., Bielders, C.L., & Defourny, P. (2011). Targeting rural development interventions: Empirical agent-based modeling in Nigerien villages. Agricultural Systems, 104(4), 354-364. [doi:10.1016/j.agsy.2010.12.007 ]
SIART, S., Weltzien, E., Kanoute, M., & Hoffmann, V. (2008). Provenances des semences de sorgho après une année de sécheresse au sud du Mali. Cahiers Agricultures, 17(2), 195-198.
SIMON, H.A. (1955). A behavioral model of rational choice. The Quarterly Journal of Economics, 69(1), 99-118. [doi:10.2307/1884852 ]
SISSOKO, S., Doumbia, S., Vaksmann, M., Hocde, H., Bazile, D., Sogoba, B., Kouressy, M., Vom Brocke, K., Mamadou, M.C., Toure, A., Dicko, B.G. (2008). Prise en compte des savoirs paysans en matière de choix variétal dans un programme de sélection = Accounting for farmer knowledge in varietal choice in a plant breeding programme. Agricultures, 17(2), 128-133.
SOUMARÉ, M., Bazile, D., Vaksmann, M., Kouressy, M., Diallo, K., & Diakité, C.H. (2008). Diversité agroécosystémique et devenir des céréales traditionnelles au sud du Mali. Cahiers Agricultures, 17(2), 79-85.
STEELE, M. J., Mollaghasemi, M., Rabadi, G. & Cates, G. (2002). Generic simulation models of reusable launch vehicles. In E. Yücesan, C.-H. Chen, J. L. Snowdon & J. M. Charnes (Eds.), Proceedings of the 2002 Winter Simulation Conference.
TRAORÉ, P.C.S., Kouressy, M., Vaksmann, M., Tabo, R., Maikano, I., Traoré, S.B., & Cooper, P. (2007). 'Climate Prediction and Agriculture: What Is Different about Sudano-Sahelian West Africa?' In Sivakumar, M.K., Hansen, J. (eds.), Climate Prediction and Agriculture. Springer Berlin Heidelberg, pp. 189-203.
VAKSMANN, M., Kouressy, M., Chantereau, J., Bazile, D., Sagnard, F., Toure, A., Sanogo, O., & Diawara, G. (2008). Utilisation de la diversite genetique des sorghos locaux du Mali. Cahiers Agricultures, 17, 140-145.
VOINOV, A., & Bousquet, F. (2010). Modelling withstakeholders. Environmental Modelling & Software, 25(11), 1268-1281. [doi:10.1016/j.envsoft.2010.03.007 ]
VOINOV, A., Kolagani, N., McCall, M.K., Glynn, P.D., Kragt, M.E., Ostermann, F.O., Pierce, S.A., & Ramu, P. (2016). Modelling with stakeholders – Next , generation. Environmental Modelling & Software, 77, 196-220. [doi:10.1016/j.envsoft.2015.11.016 ]
VOM BROCKE, K., Trouche, G., Weltezien, E., & Chantereau, J. (2010). Participatory variety development for sorghum in Burkina Faso: Farmers’ selection and farmers’ criteria. Field Crops Research, 119(1), 183-194.
WALE, E. (2008). A study on financial opportunity costs of growing local varieties of sorghum in Ethiopia: Implications for on-farm conservation policy. Ecological Economics, 64(3), 603-610. [doi:10.1016/j.ecolecon.2007.04.005 ]