Introduction
In very broad terms, cognition can be defined by the enabling conditions for adaptive, flexible behavior, where behavior also includes what people commonly call thinking (Wheeler 2005). Under these lenses, the key aspect to understand cognition becomes interactivity as opposed to the more traditional view of the isolated individual and his/her processing mechanisms (Chemero 2009). Thus, these enabling conditions lean on people, artifacts and processes working together to ensure that tasks are accomplished. In this paper, our interest is on how, in practice, organizations and their members act, make plans and implement decisions (Hodgkinson & Healey 2008) providing the ground for us to examine this interactive perspective on cognition. One turns to how individuals and groups can be effective as they identify problems and sustain, improve or set up various practices and modes of action. This systemic view is not recent in the management literature because we see it very close to the work of some of the founding fathers of the discipline (Simon 1997; Cyert & March 1963).
Consistently with the tradition of organizational behavior research (e.g., Gavetti et al. 2007), we focus on cognition. However, our approach moves the boundaries of early and more recent works by interpreting brain activities and processes as part of a larger set of “equipment”, enhanced and fostered by human co-operation. As we will detail in the next section, cognition is spread (better, “distributed” Hutchins 1995) over a diverse set of systemic resources that are internal and external to the individual. Clearly, in this view it is the process of exploiting external resources - natural, social, and artifactual (Magnani 2007; Secchi 2011) - that matters the most. While many distributed cognitive researchers have directed their interest towards natural or artifactual resources (e.g., Pedersen 2012; Steffensen 2013), fewer have paid attention to the effect of distributed social resources (Hollan et al. 2000; Secchi 2011). The activities that are reflected on various organizational practices seem to be the archetypical environment where these distributed processes can be studied. Building on recent work (Secchi & Cowley 2016), we study the case of peer-review. The case is interesting in that, while organized, it does not depend on a single institution. Further, the literature shows that, as quality control, peer-review shows several shortcomings and issues that let some scholars question its validity (see, Bornmann 2011). In this influential comprehensive review (2011), Bormann views it as a “social judgement process of individuals” (i.e. not as practice which arises between people in a meso scale). He finds no evidence that peer review is either reliable, valid or an effective predictor of impact. Starting from this basis, other scholars propose peer review be rethought as a socially distributed process (Cowley 2016).
This paper extends the example of peer-review to citation measures and, specifically, to impact factor (IF). While IF is susceptible to many criticisms (Vanclay 2011; Seglen 1997), the index is an important constraint on academic practice. The choice of IF was made on two grounds. One is that it is a widely used index that is employed - very controversially - to evaluate a journal’s standing and the relative weight of its articles. Hence, we assume that our readers would know what this measurement index is as they encountered it in their academic life. This is to say that IF is familiar to most readers and most of our arguments may be easily understood by the average academic. The other reason for using IF is that, while widely used, it is known for several flaws. One is that it is an unreliable indicator of article quality in that a journal’s IF correlates inconsistently with articles published in that same journal (e.g., Seglen 1997). Another is that the two- and five-year time span IF uses may be appropriate for some disciplines, but not for others (e.g., Curry 2012). Finally, papers with a high number of citations are very few, even in high IF journals (Colquhoun 2003), hence the vast majority of papers in high IF journals are not influential or less influential than other articles published in low IF journals. These issues can be anchored to at least two logical fallacies - i.e. connected claims that invoke reasoning that, while fallacious, appears sound (Woods 2004). IF is used to describe an academic journal as a whole, in relation to citations attracted by the articles published in there. This means that attributing characteristics of the whole (i.e. the journal) to any of its parts (i.e. the individual article) is falling on to the so-called composition and division fallacy. Another fallacy that can be evoqued is the appeal to authority or ad verecundiam, one which arises every time one uses IF to legitimize the inherent depth, sagacity, rigor, value, or strength of an article. These two have been linked to decision making (Secchi 2011, pp. 50-51) and are particularly useful in the study of cognition in that they help us reflect on how imprecise information can be due to mental frames and prejudices. Moreover, beliefs on IF is sometimes socially construed since it can be shared with professional associations, other faculty, members of a research group, or an institution’s leadership, hence connecting to the ‘organisational’ side of cognition.
In pursuing whether IF affects cognition as a socially distributed, we use an agent-based computational simulation (Edmonds & Meyer 2017a) of its dynamics in an organization. We show how individual preferences such as the attitude towards IF, publication outcomes, and evaluation proxies, together with more socially-nested aspects such as proximity to co-workers, group/departmental affiliation, and update of one’s beliefs affect the perception of the scientific value of a publication. In summary, one’s perception of the research undertaken is affected by individual and group attitudes to IF or, in other terms, by individual perceptions as well as social organizing. This work is oriented towards understanding if and how a theory of cognition that is not bounded by the skull (e.g., Clark 2003) but socially enabled (e.g., Hutchins 1995) applies to an organizational environment. The computational simulation considers all these aspects together and will, hopefully, help us refine the theoretical model.
In the following, we outline the theoretical assumptions on organizational cognition that constitute the background for the model. We then introduce the computational simulation and present its results, outlining implications for both the study of cognition in organizations and the use of IF. We conclude with limitations and a few suggestions for future research.
Theoretical Background
In their recent work, Secchi & Adamsen (2017) draw on literature from major journals that connect ‘organization’ and ‘cognition.’ They show that, while the number of papers on cognition in organizations has risen since the 1980s (as in many other areas), the field has never taken off. They address this failure by offering a logical classification as the basis for reviewing the field. One approach is additive - cognition and organization can be seen as mutually illuminating yet separable. For example, organizations can be used to explore consequences of the bounds on human rationality. In the second, combinatory category, organizations are treated as open to using cognitive concepts (and vice versa). If contingencies are central (as in Simon’s work, Simon 1997), organization is seen to be emergent, complex and hard to predict. However, while tied to input-output models, the perspective is blind to how the social and the cultural bear on individuals-in-interaction. In the intersectional category, however, one can develop a concern with phenomena such as shared cognition, teamwork and sense-making. Accordingly, Secchi & Adamsen (2017) adjudge this the most progressive approach and gives special weight to how Weick’s work has been used (Weick 1993; Weick & Roberts 1993; Weick & Sutcliffe 2006). Fourth, the conditional category treats cognition as a part of organizations in that it uses constructs like intelligence and motivation.
The underlying thread in the organizational research literature with a cognition focus is the almost unanimous interpretation of cognition as information processing. This happens for two main reasons. First, scholars typically adopt Simon’s early conceptualization of cognition as working like a computer (Newell & Simon 1972; Gigerenzer & Goldstein 1996). If minds are (like) machines, cognitive models are too coarse grained to capture the details of individual decision making and organizational life. Thus, while some focus on macro factors like organizational structure, change, and communication (e.g., March 1981), most turn to micro concerns such as individual motivation, knowledge, skills and decision making (e.g., Hodgkinson & Healey 2008). In considering a cognitive dimension together with standard organizational research these studies contribute to the advancement of the field (e.g., Crilly et al. 2008; Boal & Peery 1985; Dew et al. 2015) although they very seldom attempt at covering the macro-micro gap. One aspect that is often brought in to bridge this gap is to consider action (and, most notably interaction, see Steffensen 2013) as a key feature of cognition - sometimes referred to as embodiment (Clark 2003; Magnani 2007). So, where this inherently dualistic idea is replaced by some view of embodiment, organized activity becomes inherently cognitive. The isolationist and internal perspective of the mind-machine is thus seen as having evolved in an ecology (e.g., Gigerenzer & Selten 2001; Secchi 2011). This paper focuses on one way of construing this claim.
We are aware that different disciplinary traditions may overlap, to some extent, with the one presented in this article. For this reason, in declaring that our starting point is that of distributed e-cognition (see below Hutchins 1995; Clark 2003; Cowley & Vallee-Tourangeau 2013) and its applications to management (Michel 2007; Secchi & Bardone 2009; Secchi 2011), we are being upfront in (a) the take this article has on the topic and (b) the literature from other disciplines we might have overlooked. Hence, starting from cognitive science, we stress that, this century, almost all have come to see human cognition as embodied (for discussion, see Wilson 2002; Shapiro 2010). Second, we stress that the doings of living bodies allow people to act as parts of what Simon (1991) calls ‘intelligence units’ (hereafter, IU). Indeed, if one eschews mentalism, this is made necessary by the bounded nature of human rationality (Simon 1997). Successful organizing can only overcome an individual’s inherent limits by shaping engagement with other people and the world (Simon 1993). Simon thus stressed the intelligent use of contingencies and, in his writings, often drew on his experience in the team that designed what became the Marshall Plan. Importantly, the so-called plan self-assembled around a group (including Simon) who argued that the issues be seen in terms of the balance of trade. What he calls a representation (balance of trade) thus became, in Simon’s terms, a weapon and a tool. The group developed a formal organization to challenge how others saw the problem and, eventually, led to a major success in US foreign policy. Building on his experience, Simon (1991) defined communication as involving influences that shape decision premises. Far from focusing on individual expertise, he stressed how differently people understand, and, thus, how formal and informal structures compensate for individual limits. It is from this forward-looking perspective originating from Simon that we develop our view of organizational cognition, similarly to many social simulation scholars (e.g., Edmonds & Meyer 2017b; Conte 1999; Carley et al. 1998; Conte et al. 1997).
Beyond a computational theory of the mind
Classic cognitive science was dominated by the computational theory of mind (see, Boden 2006). As recently as 1975, (Fodor) was still calling it “the only game in town.” However, the first cracks had already appeared: Dreyfus (1972) emphasized know-how - not propositional knowledge of a supposedly objective world. Searle’s (1980) thought experiment in Minds, Brains and Programs picked out a major deficit in computational models. In his Chinese room thought experiment, Searle argued that if a program, or a little man in the head, carried out symbol processing, neither the man, the one in his head, nor indeed the one in that head (and so on) could possibly have any understanding. Not only was computation based on modeling propositions (see also Horst 1999) but neither experience nor consciousness could be clarified by programs. As the focus shifted to understanding and know-how, interest grew in how cognition draws on experience of action. Hurley (1998) pinpointed another fatal weakness in all input-output models, the computational posits a cognitive sandwich (i.e. where ‘cognition’ is a filling between a slice of perception and one of action). Computational views thus reduce individuals (and groups of individuals) to mere processing systems. Much have been learned by these approaches, but more current views highlight that a richer understanding can be gained through considering an extended system (Clark 1998, 2003). And yet, with very few exceptions (e.g., Weick’s sense-making approach; 1995 or Ocasio’s perspective, Ocasio 2001), management perspectives on cognition still align with these early computational views (Secchi & Adamsen 2017). Historically, concerns with the traditional computational approach became clearer with work in fields such as robot design and how brains and bodies co-evolved with living beings (e.g., Perico et al. 2014). This made researchers aware of the way individuals attune through body-based encounters with each other and the perceived world (see, Varela et al. 1991). For those designing computational models, the focus shifted from symbol processing to connectionism. Cognition was seen to spread beyond the brain or, in Clark & Chalmers (1998) well known terms, opened up the hypothesis of extended mind.
Evolution opened up flexibility long before the emergence of multi-cellular systems. While recent work traces the rise of brains and sensation to the Cambrian period (see, Keijzer 2015), learning is more recent and, in human worlds, adds how language co-evolved with social, technological and organizational means. Cognitive activities build on how humans learn to use interactions to discover culturally[1] appropriate ways of acting. As adults, they develop the skills used in cognitive systems that perform tasks such as navigating a ship (see, Hutchins 1995) or comparing soil samples in archeology (Goodwin 1994). As is increasingly clear, the relevant know-how connects perception with action - there is no need to posit a cognitive sandwich. Fixing a ship’s position or seeing soil color as an archeologist arise from linking bodies, central nervous systems and experience as people engage with social, linguistic and material phenomena. Humans draw on the past and imagine the future because cognition and language are distributed (see, Cowley 2011; Hutchins 2014). Given the multi-scalarity of external resources, neural functionality develops as the familiar is put to new uses (Anderson 2010). Systems self-simplify by using experience of perceivingacting in an encultured world (Berthoz 2012). Not only are contingencies useful, but what Jaynes (2000) first called excerption enables people to break from the flow of experience to attend to do/say something unexpected (Cowley & Vallee-Tourangeau 2017). Each person brings forth a world (Varela et al. 1991) through enaction and/or the perception-action coupling known as sense-making (see, Thompson 2007). Though rationality is bounded, humans ceaselessly overcome their own bodily limits: they draw on the reductions of entropy associated with what Hutchins calls a cultural ecology—this simplifies procedures, choices of discourse and ways of remembering as people come to perceive similarly (Hutchins 2014). Rather than seek a single model of cognition, most acknowledge that, at times, thinking is habitual or procedural and, at others, it is willful and uses, for example, counterfactuals (Evans 2012; Cowley & Vallee-Tourangeau 2017). In this sense, cognition is distributed and, thus, amenable to systemic investigation. Accordingly, we pursue how IF functions in relation to, not individual judgements, but how these play out in groups.
E-cognition and the management literature
The directions very shortly outlined above clearly align to those of the so-called distributed cognition perspective or e-cognition, as it has been referred to more recently (e.g., Menary 2010; Theiner 2013). According to this view, human “powers” are e-cological (sensitive to local resources), e-nacted (by sensing bodies), e-mbedded (intrinsic to perceived situations), e-mbodied (link action and perception), e-xtended (use technology and organisations) or, in a word, systemic (they bind the social, material and the temporal; Cowley & Vallee-Tourangeau 2013). By deflating the role of the brain, systemic or e-cognition ensures that individuals and groups are able to draw on a range of computational resources (Wells 2005)[2].
Placed against the recent history of cognitive science, the findings are striking. In spite of symbol flight, the turn to embodiment and rejection of the cognitive sandwich, the organizational literature is conservative. Computational assumptions ground additive, conditional and combinatory approaches (Horst 1999, 2011); in the inter-sectional view, whilst there is some embedding and enaction (Weick 1993; Weick & Roberts 1993), no allowance is made for other systemic aspects of cognition (the extended, the ecological or the distributed). In teamwork, for example, individuals act as a unit that accomplish tasks that presuppose shared goals. However, in contrast to the practical details of navigation described on the US Palau (Hutchins 1995), teams are not seen as culturally embedded, technically augmented and task-oriented systems. In our terms, little weight falls on the ecological, the embedded and the enactive.
Social organizing: A case in peer reviewing
In order to make the theoretical claims we chose a practical application to which they could be applied to. A peer-review system can be rather complex in its mix of cognitive and organizational elements (Cowley 2016) and yet it is emblematic of how modern tasks are operationalized. In fact, the nature of its activity is grounded in flexible work, voluntary and professional performance, organizational and group constraints. In an e ort to be more precise, this study limits its span to the assessment of papers (e.g., annual reviews, promotion evaluations) rather than to the process of journal double-blind peer reviewing. More precisely, using the frames from the e-cognition literature outlined above, we pursue how such a system works.
E-cological: sensitive to local resources
The cognitive process is triggered by resources that are located in the environment surrounding the decision maker. These can be classified in material or natural resources, artifacts, and socially-bound resources (Hollan et al. 2000; Secchi 2011). It is the interplay between various mixes of resources and one’s individuality (Clark & Chalmers 1998) that defines a cognitive process as such. In a review process, the article is clearly an artifact, together with the tools used to perform reviewing and revision - e.g., a printer, a pen, a highlighter, a computer, the desk in which one sits. These all enable cognitive processes leading to performing the given task. And, of course, the space/time combination of how these resources are exploited changes the dynamic of these processes. In the ecological assumption, also other individuals one interacts with are part of the cognitive environment. However, as shown elsewhere in this document (and also below), they are resources of a different kind. Finally, an ecological perspective also allows peer review to be defined in relation to the IU - that is as involving reviewer, editor, author, platform, etc. - or, in other words, to a meso area of interaction between the various players and their environments. This is particularly important due to the fact that, in standard models - especially due to the influence of Merton (e.g., 1968) - lies precisely in defining it as an individual-centered process that depends on an ideal agent (Small 2004). So it is seen in terms of the macro—as process - and the micro - what an individual does and does not do.
Consistently with some organizational scholars (e.g., Weick 1993; Weick & Roberts 1993, and also Ocasio 2001, relative to some aspects only), distributed and e-cognition keep action as an essential part of the process. This has two extremely relevant implications. First, it reminds researchers that even individual cognition happens in a time-relevant scale and that these timescales (Neumann & Cowley 2016) have important repercussions on the way individuals frames data. In peer review, it affects the way one refers and interprets (feels bound) to professional standards, for example, as to represent a long-term timescale. At the same time, one is very much affected by the particular topic of the article that, sometimes, may not perfectly fit one’s expertise, hence forcing ad hoc strategies (e.g., google searches, asking another colleague).
Second, being spread over time, any cognizant activity involves the manipulation of resources. Every time one is dealing with resources, their handling is usually more relevant than each of the resources per se. This means that, in a peer review process, having access to a computer is rather dull compared to the cognitive meaning of the word one is typing that, combined with other words and knowledge, make one think of changing it or changing its surroundings (e.g., the other words in the sentence, position, format). This continuous activity of providing new meaning to the external resource is extremely widespread in human cognition and may be referred to as re-projecting (Magnani 2007; Secchi & Bardone 2009). Moreover, any cognitive process—especially creative or knowledge-based such as assessing a manuscript—involves the possibility of tinkering, hence seeking chances and getting on something new (Bardone 2011).
E-mbedded: intrinsic to perceived situations
One of the most important assumptions of this perspective is that cognition does not exist outside of the elements in which it manifests itself as it was in the so ware/hardware perspective. This is to say that there is no process without the melding of internal (one’s brain and body) and external resources. In a review, the assessment of a given paper is necessarily the result of one’s use (or exploitation) of the resources available, through intuition, affordances and, more broadly (if not vaguely), perceptions. This also brings in the implication that a decision is almost never done the minute it is made. Quite the contrary, even though it is put externally once done (one could say externalized; Magnani 2007; Secchi & Bardone 2009), one can still “own” a decision, as in some sort of endowment effect (Kahneman et al. 1990). Once an assessment is submitted back to the author or to the head of a committee (or to an editor, in case of journal peer review), the reviewer “owns” all the arguments it contains.
E-mbodied: link action and perception
The social being requires a biological being. As obvious as it could sound, the role of the body, emotions, and overall perceptions were not taken into consideration in cognitive studies until recently (Wilson 2005; Varela et al. 1991). In a distributed e-cognition perspective, a reviewer would interpret and makes sense of the text by also ‘feeling’ it fits within one’s own domain of competence or not, for example. Also, the mental (and some-times physical) e ort required by the act of reading, understanding, interpreting, analyzing, writing, come at a cost—that of the energy dispersed to perform the task.
E-xtended: use technology and organizations
If cognition cannot be thought of without all the resources (or tools) that allow it to exist, then it is fair to state that one’s cognition extends to include these resources (Clark 2003). This is a view also called of the extended mind because it seems we ‘think’ through the use of these resources. The assessment of a paper may result differently depending on the tools used—this is to say that the making of one’s thoughts are shaped by the interaction one has with a so ware, one or more colleagues, the strategy used to “attack” the manuscript or the writing process.
The social element
Given the multi-scalarity sketched above, people shift between the more automatic (checking reference format-ting requirements are met, printing the paper) and doing things deliberately (asking advice to a colleague on a methodological point, suggesting a twist in an argument). While a combinatorial view (as in Secchi & Adamsen 2017) allows the organizational to influence the cognitive, it leaves out the socially improvised-repetition based synergies - that ground intelligence in mammals (and humans). As noted, people also experience selves and others as they use excerption to shift attention, how they act, what they say, and frames of reference. Much experience is social or, in lay terms, is bound up with collective forms of meaning. Whereas a computational view treats the social as secondary, Simon prefigures systemic approaches in seeing that information differs across persons who also vary in openness or docility (Simon 1993). This concept was originally introduced by Tolman (1932) and then developed within the bounded rationality framework in two stages in Simon’s career (Simon 1997, 1993). The idea is that humans have an attitude towards being taught (from the Latin docilis) and that this reflects on how much one listens to advice, suggestions and recommendations coming from others while making decisions. While some have elaborated on altruistic behavior stemming out of this idea (e.g., Knudsen 2003), others have pointed that the concept needs an organizational or, at least, a sense of community to effectively play its effects (Bardone 2011; Secchi 2011). Together with Secchi & Bardone (2009), we regard docility as a behavioral aspect of distributed e-cognition in that it grounds the use of external social resources to cognitive processes. By following these footsteps, we see Organisational Cognition (OC) as a field where individuals and organizations form aggregates whose functionality draws on meso scale events of social organizing. This meso scale replaces appeal to a macro-micro created by positing that Organisational Cognition is highly dependent on what has been termed social organizing.
In an attempt to connect the concepts reviewed in this theoretical framework to a practical case, Table 1 offers a sketch over one phase of peer review paper assessment. In the table, we start from a sample task, then elaborate examples for each of the e- categories and link them to a more social, or meso scale in the cognitive perspective. Finally, to further clarify the conceptually backed model, we have tried to indicate how these concepts reflect on parameters of the model as described in the next section. Here, we turn our attention to a practical example of whether OC can be framed as e-cognition to show whether and how the meso scale affects the micro (individual) and macro scale events. We use a simulated organization where two research groups have different appreciation of scientific value based on acceptance or rejection of IF.
Cognition | Short description | Sample resources | Sample meso aspect(s) | Model’s how-to |
Ecological | sensitive to local resources | The combination of knowledge, affordances of the user interface, keyboard, screen, various environmental triggers and primes | The history in using that software and familiarity with the method employed in the paper together with a possible alternative | PSV, pubv |
Enacted | cognizing "through doing" | Formulating ideas through the computer/software interaction; gaining insights on methods after a database search | Discussing implications with a colleague either in or out of one’s own research group | group,range, PSV’s α |
Embedded | intrinsic to perceived situations | Having second thoughts on the notes right before sending the email to the author | Sharing the result of the assessment with colleagues seeking an update on one’s own views | docility d, pubv |
Embodied | link action and perception | The emotional strength associated with the belief that the author is mistaken | Discounting the method currently in the paper because it falls outside the modus operandi of the research community or of the expectations of the journal the article may be sent to | docility d, group, change, aif |
Extended | use technology and organizations | The extent to which some views are carried only through the use of words in a word processing software (or email); the distraction a phone call (or a text message) brings to one’s chain of thoughts, forcing a re-evaluation once the interrupted sentence is read again | The “pressure” from the wider community of research practice in which one operates within his/her own institution; the views coming from beliefs about the appropriateness of certain methods in certain high/low IF journals | group, pub, pubif, pubv |
The Model
From the above, one should expect much cognitive processing to occur as people engage in intelligence units (IU). Due to the dynamical and complex nature of the processes, we deem an agent-based simulation model (ABM) appropriate, in line with the current organizational behavior literature (Fioretti 2013; Secchi & Neumann 2016; Secchi 2015). In fact, due to their features ABM are especially well equipped to represent the dynamic interactions of complex systems (Miller & Page 2007) and of boundedly rational agents (Secchi 2017).
Modeling impact factor
In an attempt to consistently cover most aspects of the ABM simulation, this article uses the Overview, Design concepts and Details (ODD) protocol (Polhill 2010; Polhill et al. 2008). The simulation was carried over NetLogo (Wilensky 1999), an open access computational so ware specifically designed for agent-based modeling. The model is available online in the OpenABM platform at https://www.openabm.org/model/5589/version/2/view.
The unit of analysis is cognition as it materializes among individuals in two separate intelligence units (IUs). Our approach has been that of following applied psychology and organizational behavior. Scholars from these fields sometimes measure cognition through psychometric scales and/or indices. These are summarized by numbers. Hence, we have assigned each agent a score for various elements (e.g., docility, perceived scientific value) in a way that mimics the results of a data collection exercise. Each agent in the simulation has a score for this attitude towards socially-bound distributed e-cognition processes. Thus, each agent is more or less prone to use information coming from the social channels surrounding it, i.e. the other agents. As explained below in further details, other aspects affect agent ‘thinking’ and behavior. The simulation pursues how agents may show different understandings of the same content over different periods of time and depending on their affiliation to a specific IU. The environment can be pictured as an academic or research institute where individuals are employed and per-form their work duties. As an aspect of the broader ecological and extended characteristics of one’s thinking, the simulation uses impact factor (IF) to exemplify how academics may change their understanding of what grants scientific value (PSV, see below) to their work. Consistently with our framework, we assume that this understanding depends, in part, on how cognitive processes are structured by IUs. Time, evaluation of other people’s publications, and professional exchanges with colleagues may also bring a diversity of contents to the same item (i.e. IF). The fact that the IF is a numeral is peculiar in that, superficially, this appears to place its face value beyond dispute. In examining this idea, the simulation investigates when and how a simple numeral can be perceived and dealt with differently, exploring whether this is more likely to happen when individuals interact in their IU even though they all are part of the same institute, university, or organization. All parameters and notations are specified in Table 2.
The meso scale
The model includes two “intelligence units” (labeled IU1 and IU2) that each describe how a group of academics interpret their career, and especially their publications, in relation to macro-concerns. While members of IU1 have a less restrictive interpretation of the IF, IU2 treat it as the sole metric to be used in judging publication and success. The two IUs might be two departments or, indeed, two research groups/centers in the same organization. There is also a third residual group of agents who fit between the two views of career, publication, etc. They are not part of an intelligence unit but, depending on certain conditions (described below), they may join one of the units. This general attitude to career is defined by what is labeled ‘perception of scientific value’ (PSV) in the simulation, with values distributed normally at random to all agents, with differences due to being affiliated with IU1, IU2, or no affiliation (see Table 2 for details). We interpreted non affiliation to be reflected in a wider variation of PSV values, because of lack of any anchor, while IU1 and IU2 have the same dispersion. IU2 members (i.e. IF enthusiasts) have higher PSV, on average, reflected by IU1 average + parameter alpha (see Table 2), in the sense that they ‘believe’ to have higher standards for science.
Agent characteristics
To represent organizational/social cooperation and interactivity, each agent is assigned a tendency to take on social information or a so-called ‘docility’ level (Simon 1993; Secchi 2016; Secchi & Bardone 2009). This is fixed on a random-normal distribution with standard deviation fixed at 0:2 and mean taking three values [0:6; 0:9; 1:2]. Thus, all agents have a level of docility that is assigned at the beginning of the simulation and is independent of their IU affiliation. Given that this parameter is important for interactions (see below) it is crucial it does not depend on the IU but it is something that agents have independent of affiliation. This attribute has been used in ABM before (e.g., Bardone & Secchi 2017; Miller & Lin 2010; Thomsen 2016) and it indicates the extent to which information coming from others affects agent decision making and behavior. To some extent, it is a measure of how much one is susceptible to the interactivity in a group and it operationalizes socially-oriented cognitive aspects (Secchi & Gullekson 2016). The values used to generate the three distributions have a small standard deviation such that values concentrate mostly around the mean and thus allow for clearer observation of the ‘docility effect’ (Secchi & Bardone 2009).
Independent of their IU, agents are also associated with attitudes towards the IF of a particular journal. The attitudes are distributed in the population of agents normally at random with fixed mean and standard deviation (see Table 2). Possible alignment between IF attitudes and IU are le to the dynamics of the simulation.
One assumption of this model is that each published article is associated with the IF of the journal in which it appears. Another is that, we believe, certain papers do have higher scientific value than others. Both assumptions can be challenged as unrealistic. On the one hand, many journals lack such metrics and other outlets do not qualify (e.g., books, chapters, conference proceedings). On the other hand, some social constructionists would argue that all publications are of equal value qua ‘texts’. While disagreeing with the latter view, we accept that the model simplifies by treating different IF levels as representative of various kinds of journals. However, what matters in this simulation model is how the agents perceive IF. For hard-liners (members of IU2), scientific value is a judgement about the journal and the article’s scientific value is identical to the journal’s IF. By contrast, for members of IU1, IF is a less meaningful criterion for evaluating publications since they rely on the perceived scientific ‘value’ of the article - this may or may not be aligned to the IF of the journal in which it appears.
Finally, every agent connects to other agents, preferably of the same IU through a ‘proximity rule’, indicated by the parameter range that takes two values [4; 8]. This means that each agent screens a mind space around it to see whether it is possible to connect to other agents and establish a link. The two values [4; 8] for the parameter were selected to give a relatively narrow and relatively wide reach to each agent.
Parameter | Values | Description |
steps | 500 | The maximum number of opportunities that agents have to interact with each other when dealing with problems. The simulation may stop earlier, if pub_waves reaches 24 sooner than when steps = 500. |
runs | 25 | Number of times a simulation is performed with each given configuration of parameters. |
publications, pub | ∼ [0, 4] | This is a number of publications, between 0 and 4, that each agent presents every time there is an evaluation (publication wave, see below). It is randomly assigned. |
publication IF, pubIF | ∼ [0, 5] | This is the IF associated with the journal where the publication is found, hence it is attributed to the publication. It is randomly assigned and varies between 0 and 5. |
publication value, pubv | ∼ [0, 1] | The value of a scientific contribution assigned to each publication on a random basis. |
IF attitudes, aif | ∼ N(0, 0.5) | The attitude each agent has towards the impact factor (IF) of a journal publication |
docility, d | ∼ N(0.6, 0.2) ∼ N(0.9, 0.2) ∼ N(1.2, 0.2) | The docility level associated randomly to each agent in the simulation - higher values indicate higher probability to adapt to the respective IU as well as less autonomy from the respective IU. |
perceived scientific value, PSV | ∼ N(1, 0.25) | This is the value attributed to a scientific output by an agent that is not affiliated with any IU. |
∼ N(1, 0.15) | This is the value attributed to a scientific output by an agent that is affiliated with IU1 - smaller st.dev means that values are more grouped around the mean. | |
N(1 + α, 0.15) | This is the value attributed to a scientific output by an agent that is affiliated with IU2 - the higher mean signifies stricter criteria to assess PSV. | |
PSV difference between IU1 and IU2, α | 0.25, 0.50 | The average difference in the perceived scientific value that members of IU2 have in relation to those from IU1 - this value affects directly the mean of the random normal distribution for IU2; st.dev. is unaffected. |
group | [true, false] | The tendency to be socio-cognitively closer to the other members of the IU the agent is affiliated with. |
change | [true, false] | The possibility to change IU depending on the distance of one’s PSV to the mean PSV of either IU1 or IU2. |
leaving, l | [0, 3, 5] | A random number of agents between 0 and l exits the system. |
joining, j | [0, 5, 15] | A random number of agents between 0 and j enters the system. |
range | 4, 8 | The value used to explore the environment that surrounds each agent. |
Publications
Every agent is assigned a random number of publications (max 4) at the beginning and this number is recalculated every time that there is an ‘evaluation event’. These events are called publication waves in the simulation and happen with fixed frequency (e.g., every year, semester, month). After a few pilot tests, frequency is set at every 10 seconds. Every time a publication wave comes, all previous publications are archived and excluded from the pool that is available for the current round of evaluation. This mechanism is implemented to replicate assessments done by the government or by universities, where the same publication cannot be submitted for evaluation twice.
Each publication is also assigned some intrinsic value between 0 and 1, that differs from IF (and it is not an IF function). This is intended to imply, above all, that scientific value exists independently of metrics (Vanclay 2011; Seglen 1997). Second, it allows the value of a scientific contribution and the IF of the journal to be at odds. As already mentioned above, each and every publication has an IF; this is randomly assigned and can be any number between 0 and 5. To make the simulation mimic disruptive innovation in science, one in four low IF publications are granted high scientific value. At every assessment round, one random low IF publication has its value calculated using the transformation \(y = 0.8 + IF_{pi} \cdot 0.2\), where \(IF_{pi}\) is the impact factor of the \(i^{th}\) publication. This procedure is implemented to make sure that some articles published in so-called “second tier” journals carry very high meaning for the scientific enterprise. This is to make sure that, in this case, IF enthusiasts (IU2) are necessarily wrong, because they would at first evaluate the paper badly even though it has very high scientific value.
Evaluation procedures
When a publication wave is completed, each agent evaluates papers according to the rules of their unit. IU2 members (hard core IF enthusiasts) evaluate papers on the sole basis of the journal’s IF score. Conversely, both agents from IU1 and those unaffiliated to a IU attend to their perceived value (i.e. independently of a journal’s IF). This step one of the evaluation procedure occurs as each agent evaluates one paper by another.
Step two adds a second paper to the evaluation. Each agent selects another paper to assess which is likely to be from one of the other agents in their own IU. The evaluation rules are as for the first paper (i.e. it covaries with IF in IU2 and with perceived value in IU1). In this case, the paper is ‘suggested’ by another individual who prompts the colleague to read what it thinks is ‘best’. There is one exception to the procedure. If the closer agent is from a different IU than the agent, then the evaluation focuses on the paper coming from that closer agent.
Together, the two steps ensure that each agent seeks to evaluate two papers. At this point - i.e. after two-step evaluations of, on average, two papers - a ‘socialization’ element is introduced. Agents will tend to match their evaluation with the rule of their own IU; however, they also use their docility and that of those around them to set evaluation to other standards (if they are so able). This is done to reflect social influences on individual thinking and behavior in organizations (e.g., Simon 1993; Secchi 2011). The mechanism passes through a function that adjusts both evaluations and their beliefs depending on how far they are from the others in the IU and in the system in general. The first phase of step three is an internal update. Agents with high docility (i.e. higher than the mean) update their paper evaluations by adjusting as a function of their docility. This is an internal update because agents with high docility second guess themselves and re-evaluate papers by slightly updating their initial judgement (Secchi & Bardone 2009). This step is done to allow highly docile individuals to come up with a more differentiated evaluation than the other agents (see the online materials for details).
The second phase of step three is an external update. This depends on interactions between agents. If both agents have high docility, then each updates its evaluations following the other agent (i.e. if that is higher, the value goes up and, if it is lower, the value goes down). The agents also update PSV depending on whether or not the ‘other’ individual is from the same or another IU. If docility levels are misaligned, whereas the highly docile updates its evaluations in relation to the other, the agent with lower docility updates its evaluations only when the other individual is from the same IU. It is important to remember that all the evaluation phases in step three arise between agents that are connected through a link and in a given range.
Model dynamics
The initial set up starts with a fixed number of agents (100) that randomly appear on a two-dimensional space. Their position is intended to be a ‘mental space’—not just a physical location. The initial number can be altered with those leaving and joining, to mimic retirements, employee turnover, hiring, and other organizational processes of this kind. Where a number of agents leave the organization permanently they are removed from the simulation right after a publication wave. The number can range randomly between 0 and the upper limit that is fixed in the simulation runs to [0, 3, 5] (see Table 1). The number of agents that join the organization creates new agents right after a publication wave and can be of any number between 0 and the upper limit (randomly fixed at [0, 5, 15]). These two conditions of leaving and/or joining start from publication wave 3 and add more dynamism to the simulation, with rapid expansion, when joining tends to 15, and leaving ranges between 0 and 3, or decline, when joining is 0 and leaving can be up to 5. This is in line with Simon’s characterization of IU (Simon 1991).
Soon after their appearance, agents characterize themselves as members of IU1, IU2; a minority will have lack of membership. Agents then initially connect together with other same-IU members based on proximity (or range in Table 1) and start paper evaluations as soon as the new publication wave arises. Agents also connect with other agents from outside their own IU by using a subset of range - i.e. only if these are closer than members of their own IU. This is done under the assumption that it takes a bit more ‘likemindedness’ to be willing to connect to someone from outside of one’s own group.
Once relationships are established, the three-step evaluation can take place. One of the implications of this is that, at some point, unaffiliated agents may decide to join one of the two IU groups. These agents join groups depending on whether PSV is closer to the average of IU1 or that of IU2.
In the group condition, agents from the same IU stay clustered together and thus limit interactions with other agents outside their own group. This condition is implemented to separate the two IUs so that it is easier to observe emerging coalitions. This is the case when an organization prompts groups (departments, centers) to develop an independent climate or, roughly speaking, to live the organization in different ways. The change condition allows for all agents to shift from their initial IU placement. The mechanism whereby this happens is fixed by PSV such that, where one’s PSV is closer to the mean PSV of the other IU, this agent ‘feels’ closer to the others and shifts to their IU. There is no limit as to how often an agent changes affiliation.
Findings
After a few pilot runs to study convergence and sensitivity, we settled on the set of conditions described in Table 1 and framed as a factorial design of \(2^7 \cdot 3^3\). This triggers the question of how often the simulation should run per each configuration of parameters (Ritter et al. 2011; Lee et al. 2015). Provided that computational experiments are assimilated to real life experiments (e.g., Hoser 2013), statistical power analysis can be used for this purpose (Secchi & Seri 2017). Using a smallest effect size of interest (SESOI) approach (Seri & Secchi 2017), we calculate a number that satisfies the conditions for power \(1 - \beta = 0.95\) at the \(0.01\) significance level for an effect size of \(0.1\) (Cohen 1988), in an attempt to be conservative. The factorial design leads to 3456 different configurations of parameters and power calculations indicate that 11 runs are sufficient. However, in striving to make sense of the four conditions (group x change = 2 \(\cdot\) 2) separately, we also need to know how many runs are sufficient for 3456=4 = 864 configurations. This leads to 21 runs per configuration, given the SESOI approach, we set on 25 times per configuration of parameters, leading to an estimated expected power of 0.98.
As far as time for each run is concerned, the simulation spans 24 evaluation periods. If we assume that evaluations happen every year and that every academic may publish between 0 and 4 peer reviewed articles a year[3], then we have 24 years in the life of a hypothetical organization. At every evaluation round (i.e. publication wave) we take a snapshot of agent’s characteristics and affiliation.
Analytical strategy
The data produced by the simulation was computed on the Abacus 2.0 supercomputer, available through the Danish e-infrastructure cooperation (DeIC) to universities and research centers in the Europe. The information in the file amounted at approximately 1.05 Gb of data. Specific simulation results are calculated with the aid of graphics and the generic impact of parameters is presented in fixed effects panel regression models as reported in the Appendix. All calculations are performed using R, an open source platform for statistical computing.
As usual with ABM simulations, the results presented here are a selection from a set of findings that would require more than one paper to be properly introduced. While overall results are presented in the Appendix, we deem those that follow to be most relevant in pursuing our enquiry on whether how meso scale events and interactions contribute to organizational cognition[4].
A range effect
The scope of the analysis is to unveil the circumstances (if any) under which cognitive processes involve a wider social context that is located in between the macro and the micro scales, specifically in what we call meso scales or social organizing. Figure 1 - graphs (a) to (f) - pictures regression lines that estimate how PSV for members of IU1 (blue lines) and IU2 (red lines) evolves as average IF increases due to the variation of range, leaving, and joining the organization. All six graphs compare regression lines where agents are allowed (dotted lines) or not allowed (solid lines) to change intelligence unit.
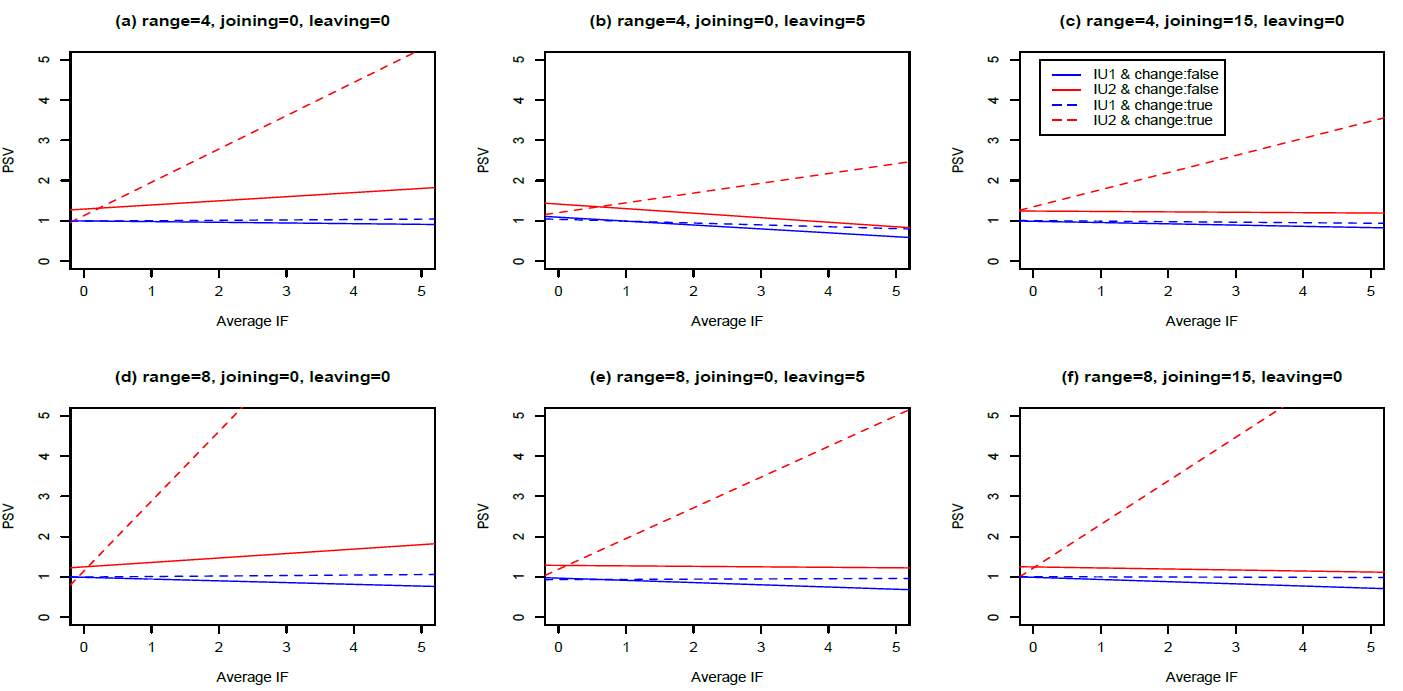
The parameter range indicates the approximate reach of each agent; the higher its value, the more likely it is that agents interact with others outside their own IU. This should have significant repercussions on agents’ PSVs because, conditional on their docility levels, they might update their evaluations more often. In the graphs presented in Figure 1, mean docility is held constant at 0.6, while range varies. Actually, between graphs (a) and (d), (b) and (e), and (c) and (f), range is the only parameter that changes from 4 to 8. This leads to an increase in the effects that take place, on average, for both IU1 and IU2. Under all conditions, IU1’s PSV seems to be little affected by either range values or change and, accordingly, there is only a slight strengthening of the registered effect. Being able to change IU leads to an increase in the agents’ PSVs; this is minimal for IU1 and very strong for the IU2 members which are also affected by an increase in the range of interactions.
Strengthening group ties
Figure 2 to 7 build on findings from Figure 1 and specify what happens to PSV when IU members intensify their relations and group ties (parameter group = 'true' or 'on') as opposed to when their ties weaken (parameter group = 'false' or 'off'). Figure 2 and 3 show the impact of the group effect as a selection of up to 0, 3, or 5 agents leave the organization at every evaluation round, with docility = 0.6, joining = 0 and change = 'false'. They can be intended as the baseline cases, i.e. those figures that are used as a benchmark for the others. In 3, as we get closer to the end of the rounds (i.e. 24), IU1’s PSVs suffers a very slight decline, moving from 1.0 to ca. 0.9, where a group effect is not detectable at all (see also Model 1 in Table 3, in the Appendix). Figure 2 shows values for IU2’s PSVs. From the figure we can observe that the overall tendency is for an increase of PSVs, except when fewer agents leave the system (leaving = 5). Here too, the group effect does not seem to be particularly strong or detectable at all and changes in PSV appear mild.
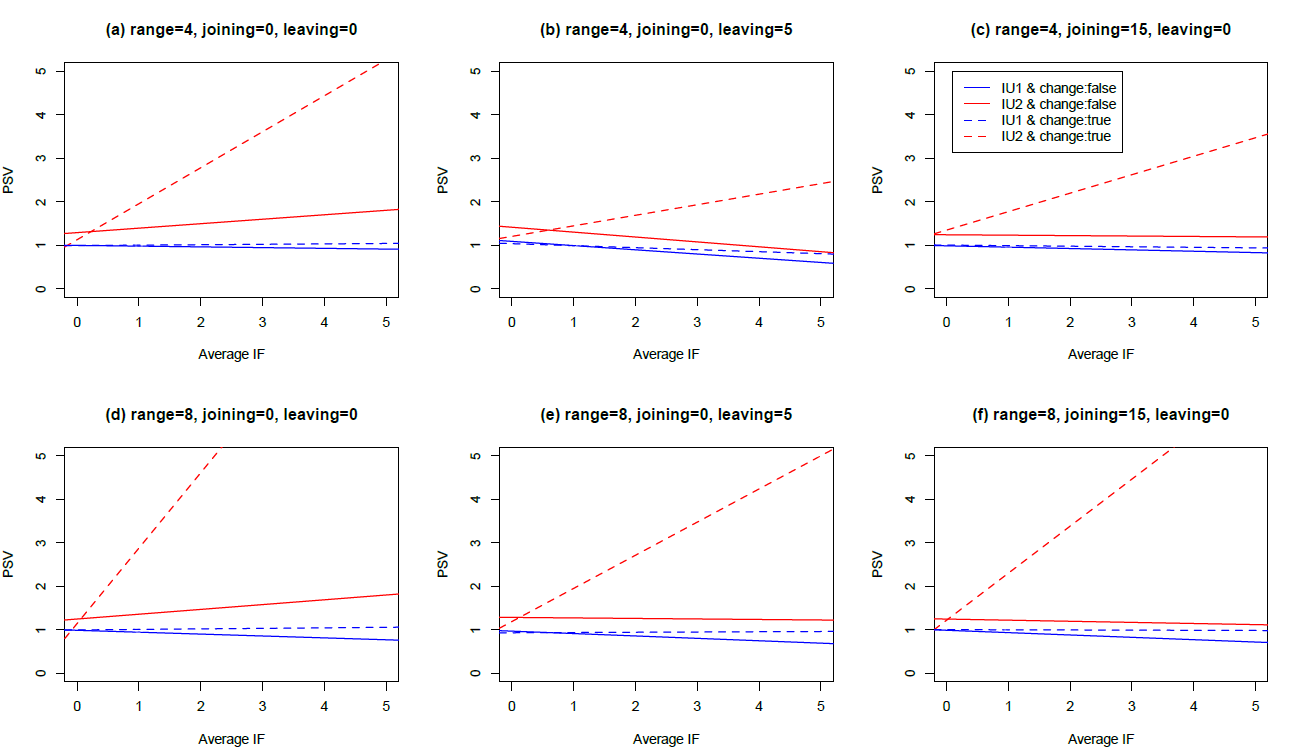
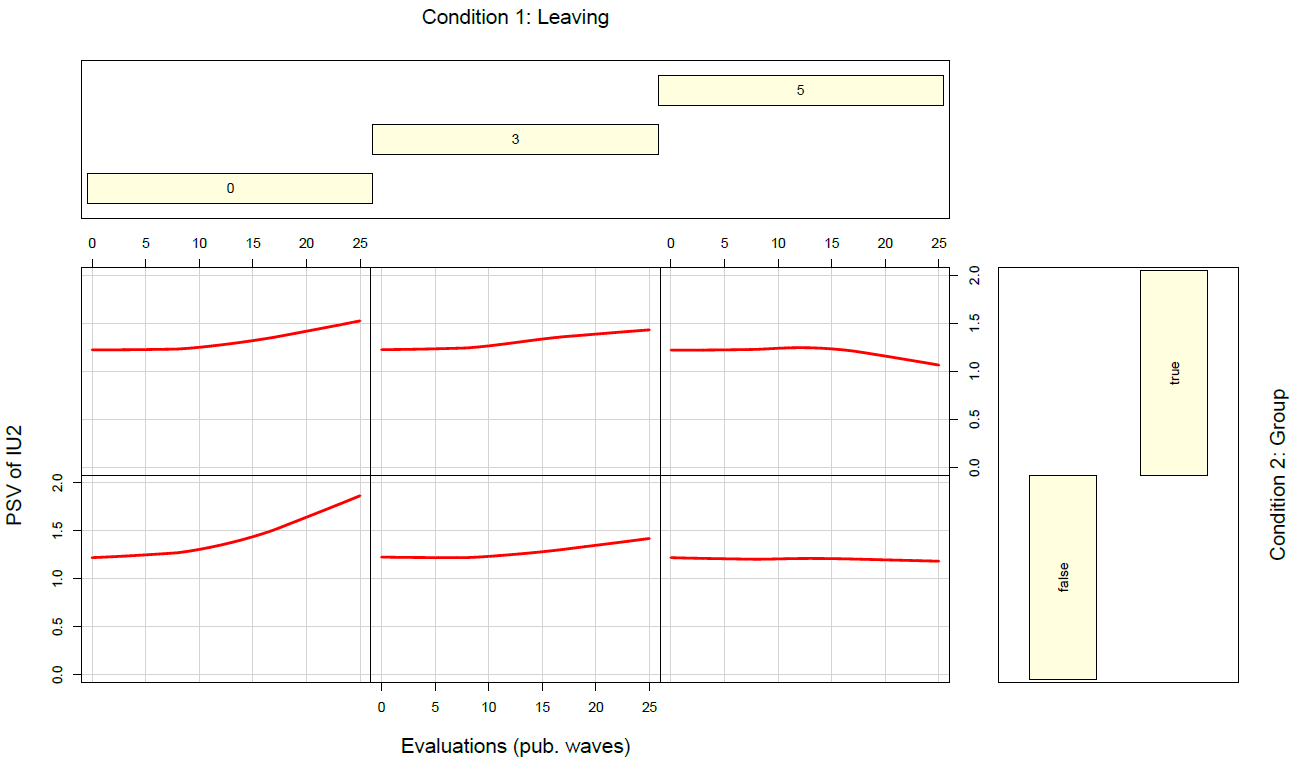
Changing affiliation
A better indication of how the group affects the model becomes apparent from Figure 4, showing how IU2’s PSV evolves over time when agents can change affiliation (change = ‘true’ or ‘on’). Results for IU1 are omitted because they do not vary from what already presented in Figure 3. As more agents leave the organization, IU2’s PSV slows the pace of its increase. This seems not to be proportional to the declining number of agents that belong to IU2 (Figure 5), hence suggesting that the variation in PSVs is to be associated with group effects and numbers of agents leaving the organization. However, there might be an effect of agents moving away from IU2 because of higher requirements for PSV.
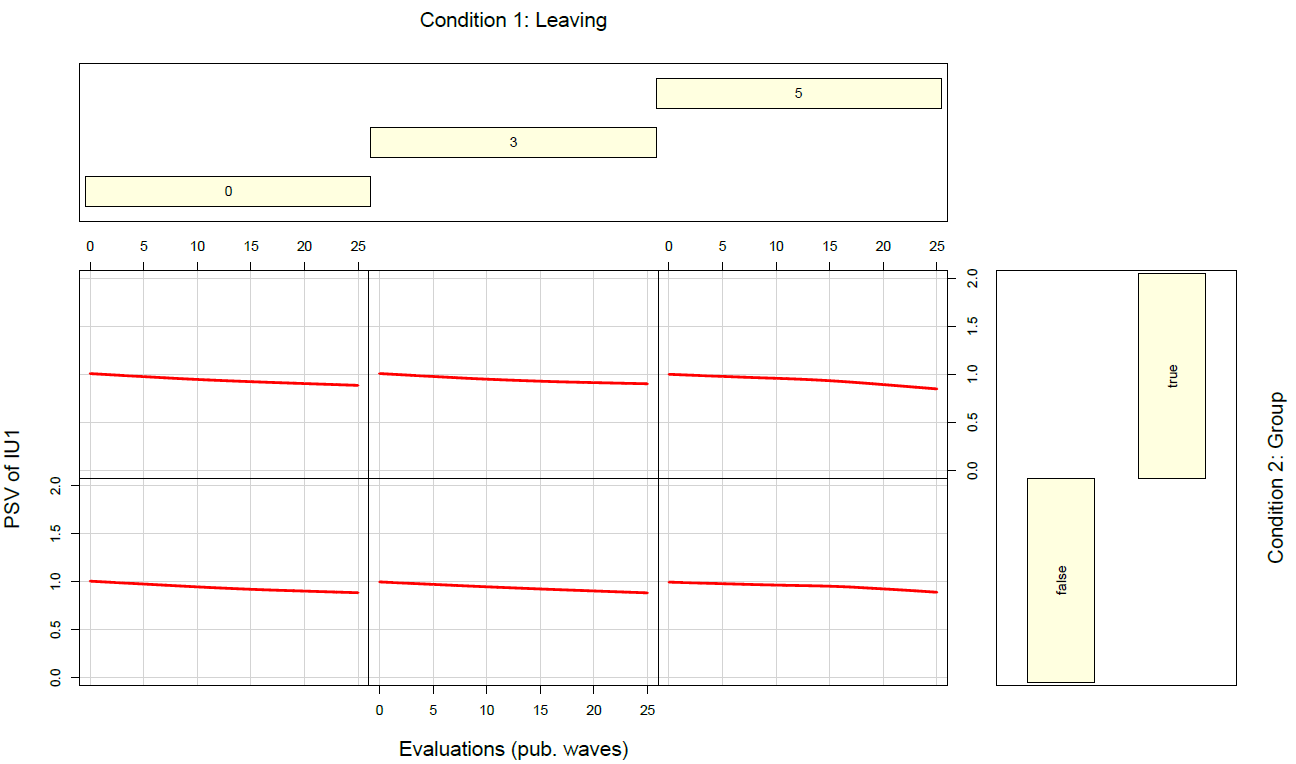
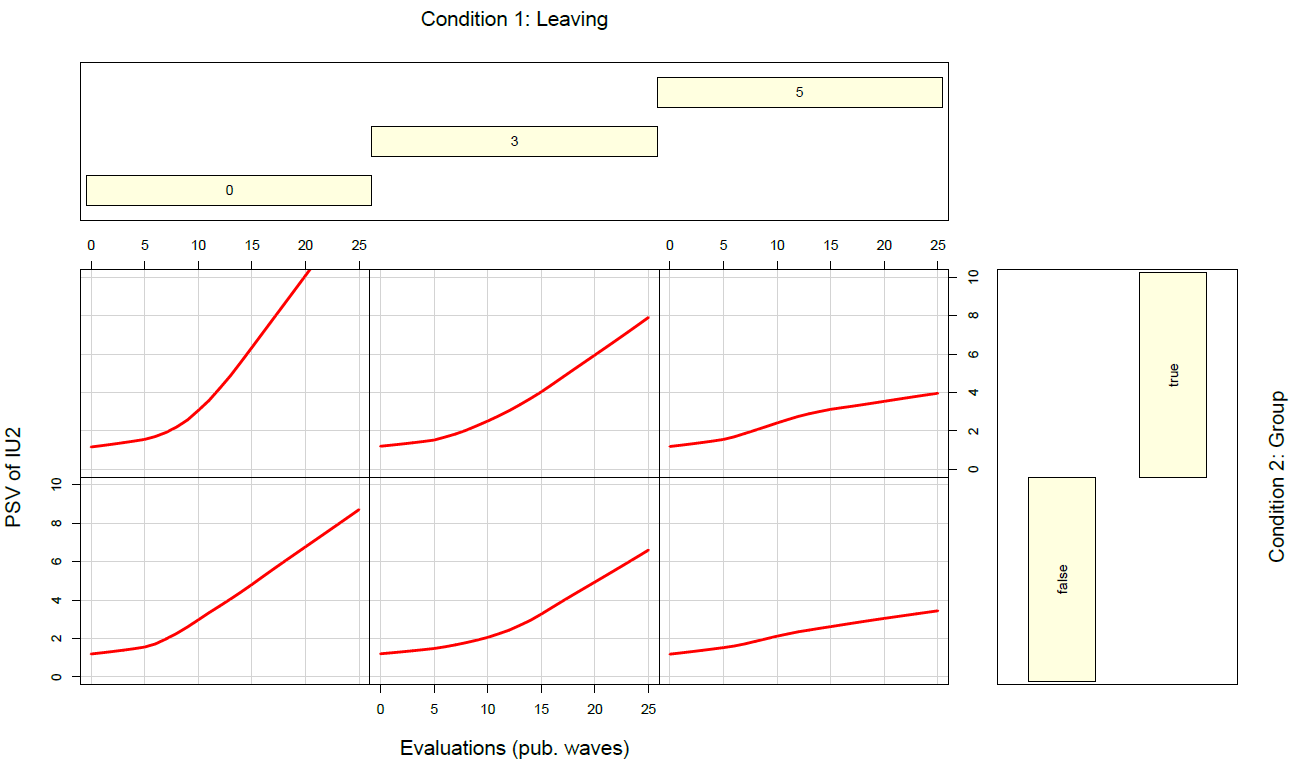
One step forward in this direction is offered by looking at the following figures where PSV levels for IU2 are calculated when docility mean levels increase to 1.2 (Figure 6) and, in addition to this condition, when joining is also set to its maximum (Figure 7). All the other conditions are the same as in Figure 4. For IF-purists an increase in docility means that they would be, on average, more open to external (different) influences on the way to assess scientific value (PSV in our simulation). Comparing Figure 7 with Figure 6 it can be seen that this effect does not influence IU2 when there is little variability (i.e. leaving is set to wither 0 or 3). However, it shows that PSVs decline towards the end of the evaluation periods when leaving can be up to 5. After examination of the data, we found that the number of IU2 agents is down to zero at round 19 under that condition (Figure 6), meaning that all agents are affiliated with IU1 (non-IU are difficult to find under change = true). So, a higher degree of docility seems to wipe out IU2 in an organization facing decline (i.e. leaving = 5), because some leave while all remaining others move to IU1.
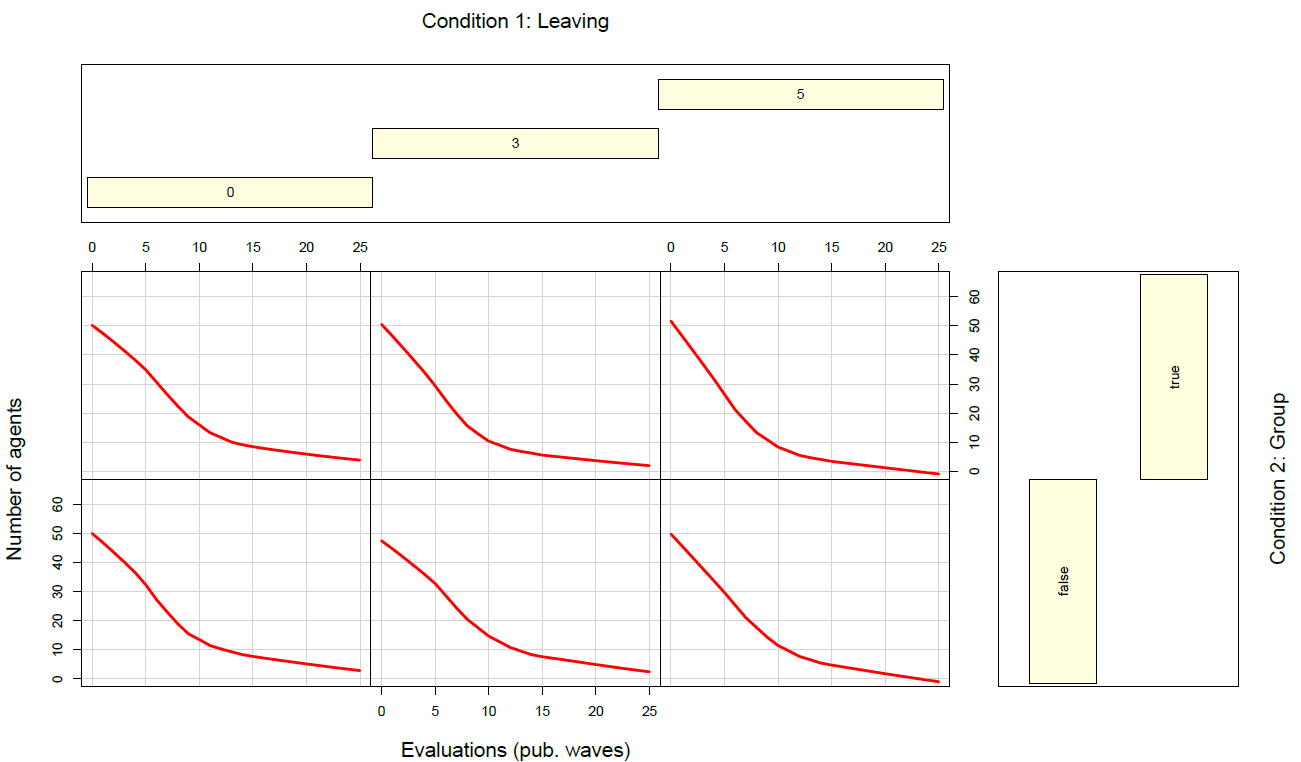
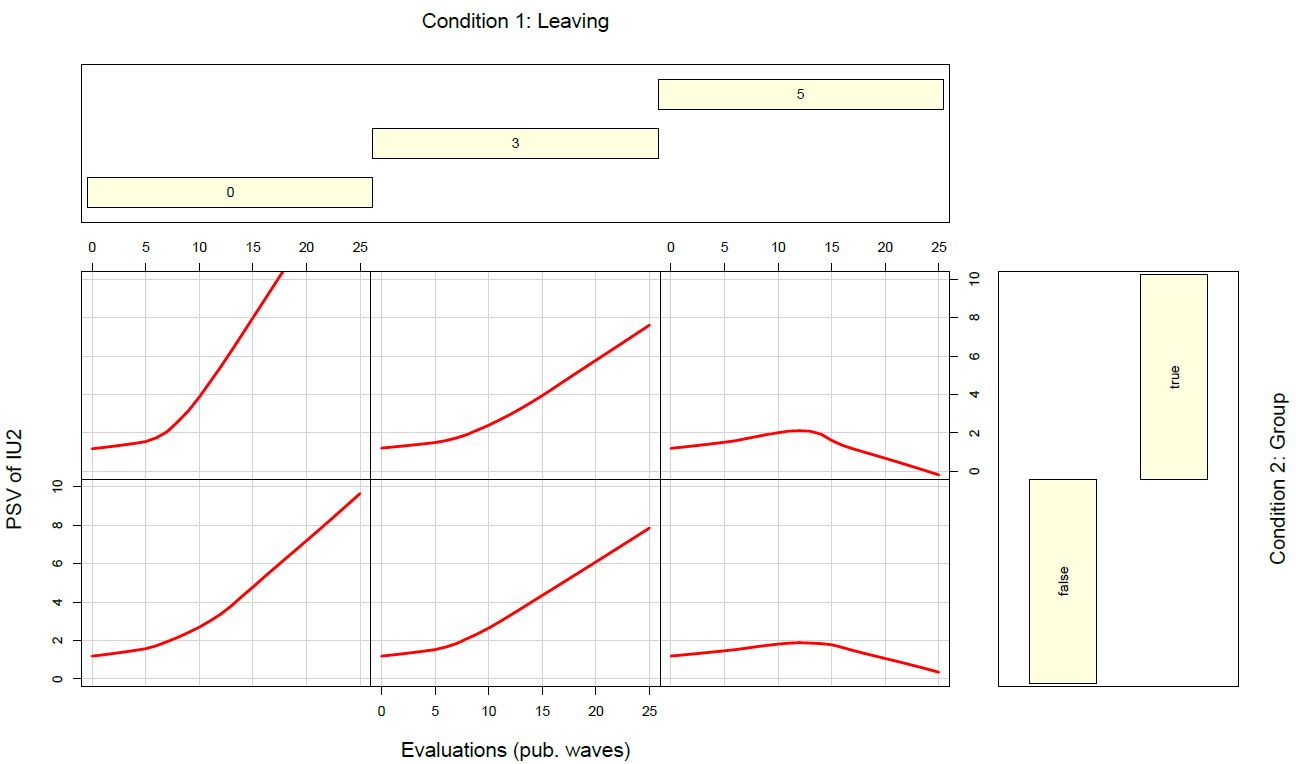
These findings are confirmed by data in Figure 7 where the organization faces high turnover, with many agents leaving but, on average, more coming in (i.e. joining = 15). While the group effect does not impact on the shape of the curves, there is a decrease in PSV - i.e. a more consistent evaluation over time - when more agents leave. The effect of docility on IU2 seems to be that of the less radicalized view, as if IU2 agents would abandon a rigid interpretation of the IF and converge towards a more stable PSV (more like IU1). Less variability in numbers radicalizes the positions instead. Figure 8 presents what happens to IU2’s PSV when individuals leave and join the organization (with change = true, group = true, docility = 1.2). Highly turbulent organizational environments (higher agent turnover rates) seem to flatten the curve while more static organizational environments appear to increase PSV, as if the IF-based evaluations need to always be better at every round.
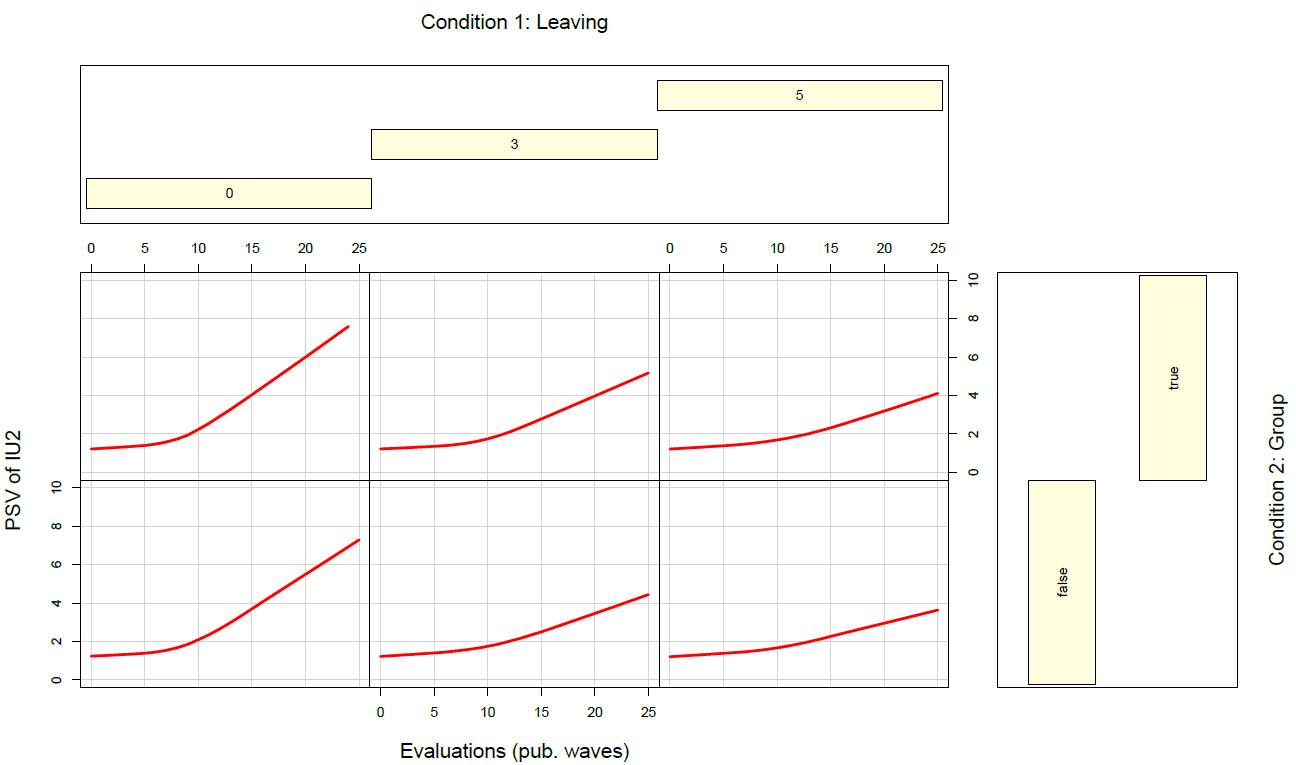
Implications and Conclusions
We interpret the ABM model of IF and PSV in relation to two main implications, each of which draws on a scale from the perspective of organizational cognition defined in the theoretical framework above.
The effect of macro scales
These results are partially consistent with what we might expect from members of the two IUs. On the one hand, under the specific conditions analyzed above, members of IU1 are not affected by the value of IF because they tend to base PSV on other parameters and, as shown, this is what happens. Even when IU1 interact more with non-IU1 agents (i.e. when range takes higher values), PSV increases slightly and it seems not affected by an in-crease in IF values. On the other hand, there is a positive impact of IF on PSV for IU2; this applies especially when there are opportunities to change affiliation status (i.e. IU). In this case, it seems that interactions strengthen how IF positively affects PSV for IU2 members [5]. The parameter change fine tunes the IU, making them more orthodox so that IU1 becomes populated by more IF-indifferent agents and IU2 is populated by IF-enthusiasts. In general, however, IU1 members are less affected by institutional or macro pressures, such as the opportunity to change affiliation. IU2, by contrast, tend to increase PSV as IF increases; this becomes more radical as change of IU becomes possible (i.e. bears no institutional costs) and in relatively stable organizational environments (i.e. agent turnover is low). This leads us to reflect on how meso scales filter macro or institutional scales in that they make agents process them differently. By implication, not only are macro aspects perceived differently by different individuals but how they are perceived is a function of the IU that constitutes the medium through which these elements come to the agent’s knowledge.
Relative reliance on the social individual
The impact of macro scales varies depending on the different conditions in which it unfolds. In our simulation of the effect of IF on cognitive processes as they relate to scientific value, a macro framework is filtered by the IU but, of course, this does not reduce the role of individual characteristics. For example, results show that average high levels of docility (i.e. d = 1:2) lead agents affiliated with IU2 to an increase in PSV as evaluations (i.e. publication waves) pass by, when change of IU is possible, and when there is a relatively stable organizational environment (leaving and joining are set to zero). Under the same configuration of parameters, agents affiliated with IU1 do not behave similarly; on the contrary, they show stable PSV (results omitted here but available online). Hence, this underlines that individual-bound cognitive processes have values that are, perhaps, less than expected given that the same characteristic - e.g., docility is distributed at random so that the means of IU1 and IU2 are approximately the same - brings forth different results. All we can tell by using the results from the simulation model is that individual cognition still plays a role, but it does so only within the boundaries of an IU.
Another explanation for the role of docility in the simulation is that this attitude towards the information from social channels may only be effective within the framework of an agent’s IU. This interpretation is consistent with previous research in that it shows docility to promote openness in the short run but conformity in the long run (Secchi 2016; Secchi & Gullekson 2016). In the perspective of social organizing - and of organizational cognition -, it seems to be one of the core ingredients allowing it to work.
Limitations
The simulation helped us specify practical mechanisms through which organizational cognition can be conceptualized. In so doing, it also pointed to limitations of the model. One of these is that we selected features of the individual (e.g., docility, IF attitudes, affiliation) and the organization (e.g., change, turnover, grouping) while le out some others that might play a role in PSV such as motivation, performance, job satisfaction, leadership, role models, and positions. We believe these are points to carry over on the simulation to shape future research. Another limitation of the simulation is that it models generic aspects for the micro/individual scale that may have been played more consistently with mainstream literature. However, the computational aspect of agents’ decision making is in line with mainstream management literature. In fact, it is worth noting that we have not abandoned the computational view but, rather, rejected any notion of an input/output sandwich. In fact, the error of classic cognitive science - but not Simon - was the form of individualism that arose with un-networked computers. This is the error of assuming that ‘social judgements’ somehow occur ‘within’ individuals when, in fact, they are part of interaction (with people and artifacts). The assumptions of the theory have been tested by computational simulation even if the nature of what it is computed and how it is calculated is very different from classic computational models of mind.
Concluding remarks
The most relevant aspect of the theoretical framework is exemplified by IF because, as all academics know, it is indicative of diversity in PSV. IF thus provides a macro framework that many organizations use as a point of reference in evaluation of scientific outcomes. We hope that readers will find it self-evident that how IF is interpreted and framed varies. The simulation emphasizes the differences and links PSV of academic articles to the way a research group (IU) interprets it. In fact, individual evaluations are based on a function of the agent’s characteristics (e.g., docility, location), the IU to which it belongs (e.g., IF estimators, IF detractors, tightness of links among members), and to wider social pressures (e.g., turnover, no cost for a change of affiliation within the organization). Results show that organizational cognition cannot be described without an intermediate meso scale that filters and enables the many kinds of socially enabled perception, action and behavior that are so characteristic of human cognition.
Notes
- Another obvious addition to the list is that of defining cognition as also e-mbrained. Even though the brain plays a role that is less central, it is still relevant - a conditio sine qua non (i.e. necessary but not sufficient) for cognitive processes to happen. After careful consideration, we decided to leave this one ‘e’ out of the framework. This is because one of the objectives of the paper is to highlight the novelty of systemic cognition, hence focusing on the more disruptive aspects of the approach seemed more consistent. Moreover,we have modeled this aspect only indirectly
- The use of the word ‘culture’ here refers loosely to values and norms established by a community of individuals. As such it has very little to do with the use of the word in either the sociological or organizational literature (Abrahamson & Fombrun 1994; Cameron & Quinn 2011; Schein 1996). Instead, it is in line with the recent Hutchins (2014) indicating a relatively stable ecosystem of individuals interacting together. This may entail something sporadic such as a single task, or something done more systematically such as a routine. In the latter case, the word ‘culture’ becomes closer to its well-known use in the literature
- Five peer-reviewed publications per year would be close to Herbert Simon 1993’s—a Nobel laureate—average; given that the numbers are calculated randomly, we wanted to avoid cases when most academics have very high number of publications. Hence, we excluded Nobel laureate’s performance style from our simulation.
- Results of non-IU agents have been excluded from this section because of small numbers for non affiliated agents and their tendency to become part of IU1 when the change condition is ‘on’. More details, together with the model, the code, specification documentation, and a selected data file are available on the open access plat-form for agent-based simulations OpenABM at https://www.openabm.org/model/5589/version/2/view.
- If one looks at results when all conditions are factored in, then the regression Table 3 (Appendix) shows that the overall effect of IF is statistically significant for IU1’s PSV (the beta coefficient is very small 0.006 but
significant at p < 0.001). In other words, and very surprisingly, members of IU1 respond positively to IF. Further, since the effects of grouping (i.e. strengthening in-group ties), the possibility to change IU, and the effect of docility are small, it seems that, unlike IU2 members, other factors do not counterbalance their reactions to IF change. Their own parameters co-function strongly with their affiliation. Members of IU2 are thus less reactive, overall, to changes in IF and more so to a range of other factors. However, leaving speculation aside, we prefer to emphasize that the overall results show clearly that the evolution is not explicable by single factors and, above all, not solely in relation to agent characteristics.
Appendix
A series of Hausman tests (Hausman 1978) were performed to evaluate whether the fixed was preferred to the random coefficient panel regression using the R package plm (Croissant & Millo 2008). These tests resulted significant with a p value < 0.01, indicating that the fixed effects was a better fit to the data.
Model 1 DV=PSV of IU1 | Model 2 DV=PSV of IU2 | Model 3 DV=\(\frac{PSV_{IU1}}{PSV_{IU2}}\) | |
Average IF | 0.006 (0.0008) | 0.010 (0.0238) | 0.173 (0.0200) |
PSV difference | 0.048 (0.0008) | 0.086 (0.0261) | 0.014 (0.0225) |
Leaving | 0.001 (0.0000) | 0.0039 (0.0016) | 0.037 (0.0014) |
Joining | 0.001 (0.0000) | 0.0012 (0.0005) | 0.021 (0.0005) |
Group (true) | 0.000 (0.0002) | 0.0034 (0.0065) | 0.007 (0.0056) |
Change (true) | 0.025 (0.0002) | 1.268 (0.0070) | 0.124 (0.0059) |
Mean docility | 0.007 (0.0004) | 0.028 (0.0133) | 0.023 (0.0115) |
Range | 0.004 (0.0000) | 0.164 (0.0016) | 0.005 (0.0014) |
IU1 publications (mean) | 0.101 (0.0003) | 1.093 (0.0096) | |
IU1 publications (sd) | 0.244 (0.0005) | 2.207 (0.0167) | |
IU2 Mean PSV | 0.011 (0.0000) | ||
IU2 publications (mean) | 0.397 (0.0090) | 1.468 (0.0079) | |
IU2 publications (sd) | 0.931 (0.0159) | 4.261 (0.0141) | |
IU1 Mean PSV | 8.708 (0.0263) | ||
R-squared | 0.38 | 0.16 | 0.16 |
F-statistic | 53771 | 16755.4 | 15454.8 |
Degrees of freedom | 11 and 962858 | 11 and 962858 | 12 and 962857 |
p-value | 0.000 | 0.0000 | 0.0000 |
References
ABRAHAMSON, E. & Fombrun, C. (1994). Macroculture: Determinants and consequences. Academy of Management Review, 19(4), 728–785.
ANDERSON, M. L. (2010). Neural reuse: A fundamental organizational principle of the brain. Behavioral and Brain Sciences, 33(4), 245–266.
BARDONE, E. (2011). Seeking Chances. From Biased Rationality to Distributed Cognition. New York, NY: Springer. [doi:10.1007/978-3-642-19633-1]
BARDONE, E. & Secchi, D. (2017). Inquisitiveness: Distributing rational thinking. Team Performance Management, 23(1/2), 66–81.
BERTHOZ, A. (2012). Simplexity: Simplifying Principles for a Complex World. New Haven and London: Yale University Press. [doi:10.12987/yale/9780300169348.001.0001]
BOAL, K. B. & Peery, N. (1985). The cognitive structure of corporate social responsibility. Journal of Management, 11(3), 71–82.
BODEN, M. A. (2006). Mind as Machine: A History of Cognitive Science. Oxford: Clarendon.
BORNMANN, L. (2011). Scientific peer review. Annual Review of Information Science and Technology, 45, 197–245.
CAMERON, S. & Quinn, R. (2011). Diagnosing and Changing Organizational Culture. San Francisco, CA: Jossey-Bass.
CARLEY, K. M., Prietula, M. J. & Lin, Z. (1998). Design versus cognition: The interaction of agent cognition and organizational design on organizational performance. Journal of Artificial Societies and Social Simulation, 1(3), 4: https://www.jasss.org/1/3/4.html.
CHEMERO, A. (2009). Radical Embodied Cognitive Science. Cambridge, MA: MIT Press.
CLARK, A. (1998). Being there: Putting Brain, Body, and World Together Again. Cambridge, MA: MIT Press.
CLARK, A. (2003). Natural-Born Cyborgs. Minds, Technologies, and the Future of Human Intelligence. Oxford, UK: Oxford University Press.
CLARK, A. & Chalmers, D. J. (1998). The extended mind. Analysis, 58, 7–19. [doi:10.1093/analys/58.1.7]
COHEN, J. (1988). Statistical power analysis for the behavioral sciences. Hillsdale, NJ: LEA, 2nd edition edn.
COLQUHOUN, D. (2003). Challenging the tyranny of impact factors. Nature, 423, 479.
CONTE, R. (1999). Social intelligence among autonomous agents. Computational and Mathematical Organization Theory, 5(3), 203–228. [doi:10.1023/A:1009634206383]
CONTE, R., Hegselmann, R. & Terna, P. (1997) (eds). Simulating Social Phenomena. New York: Springer-Verlag.
COWLEY, S. J. (Ed.) (2011). Distributed Language. Amsterdam: Benjamins. [doi:10.1075/bct.34]
COWLEY, S. J. (2016). How peer-review constrains cognition: On the frontline in the knowledge sector. Frontiers in Psychology, 6, 1706.
COWLEY, S. J. & Vallee-Tourangeau, F. (Eds.) (2013). Cognition Beyond the Brain. Computation, Interactivity and Human Artifice. London: Springer, second edn. [doi:10.1007/978-1-4471-5125-8]
CRILLY, D., Schneider, S. C. & Zollo, M. (2008). Psychological antecedents to socially responsible behavior. European Management Review, 5(3), 175–190.
CROISSANT, Y., & Millo, G. (2008). Panel data econometrics in R: The PLM package. Journal of Statistical Software, 27(2), 1-43. [doi:10.18637/jss.v027.i02]
CURRY, S. (2012). Sick of impact factors. blog post: Occam’s typewriter. http://occamstypewriter.org/scurry/2012/08/13/sick-of-impact-factors/.
CYERT, R. M. & March, J. G. (1963). A Behavioral Theory of the Firm. Englewood Cli s: Prentice-Hall.
DEW, N., Grichnik, D., Mayer-Haug, K., Read, S. & Brinckmann, J. (2015). Situated entrepreneurial cognition. International Journal of Management Reviews, 17(2), 143–164.
DREYFUS, H. L. (1972). What Computers Can’t Do. New York: MIT Press.
EDMONDS, B. & Meyer, R. (Eds.) (2017a). Simulating Social Complexity. A Handbook. Heidelberg: Springer, 2nd edn.
EDMONDS, B. & Meyer, R. (2017b). 'Introduction to the handbook.' In B. Edmonds & R. Meyer (Eds.), Simulating Social Complexity. A Handbook. Heidelberg: Springer, 2nd edn. [doi:10.1007/978-3-319-66948-9_1]
EVANS, J. (2012). 'Dual process theories of deductive reasoning: facts and fallacies.' In K. J. Holyoak & R. G. Morrison (eds), The Oxford handbook of thinking and reasoning. Cambridge, MA: pp. 115–133.
FIORETTI, G. (2013). Agent-based simulation models in organization science. Organizational Research Methods, 16(2), 227–242. [doi:10.1177/1094428112470006]
FODOR, J. A. (1975). The language of Thought. Cambridge MA: Harvard University Press.
GAVETTI, G., Levinthal, D. & Ocasio, W. (2007). Neo-Carnegie: The Carnegie school’s past, present, and reconstructing for the future. Organization Science, 18(3), 523–536. [doi:10.1287/orsc.1070.0277]
GIGERENZER, G. & Goldstein, D. (1996). Mind as a computer: Birth of a metaphor. Creativity Research Journal, 9(2-3), 131–144.
GIGERENZER, G. & Selten, R. (2001). Bounded Rationality. The Adaptive Toolbox. Cambridge, MA: MIT Press.
GOODWIN, C. (1994). Professional vision. American Anthropologist, 96(3), 606–633.
HAUSMAN, J. A. (1978). Specification tests in econometrics. Econometrica, 46(6), 1251–1271. [doi:10.2307/1913827]
HODGKINSON, G. P. & Healey, M. P. (2008). Cognition in organizations. Annual Review of Sociology, 59, 387–417.
HOLLAN, J., Hutchins, E. & Kirsh, D. (2000). Distributed cognition: toward a new foundation for human-computer interaction research. In Proceedings of the ACM Transactions on Computer-Human Interaction (TOCHI), vol. 7, (pp. 174–196). New York: ACM Digital Library [doi:10.1145/353485.353487]
HORST, S. W. (1999). Symbols and computation a critique of the computational theory of mind. Minds and Machines, 9(3), 347–381.
HORST, S. W. (2011). Symbols, Computation, and Intentionality. A Critique of the Computational Theory of Mind. Berkeley and Los Angeles: University of California Press.
HOSER, N. (2013). Public funding in the academic field of nanotechnology: A multi-agent based model. Computational and Mathematical Organization Theory, 19(2), 253–281.
HURLEY, S. L. (1998). Vehicles, contents, conceptual structure, and externalism. Analysis, 58(1), 1–6. [doi:10.1093/analys/58.1.1]
HUTCHINS, E. (1995). Cognition in the Wild. Cambridge, MA: MIT Press.
HUTCHINS, E. (2014). The cultural ecosystem of human cognition. Philosophical Psychology, 27(1), 34–49. [doi:10.1080/09515089.2013.830548]
JAYNES, J. (2000). The origin of consciousness in the breakdown of the bicameral mind. Houghton Mifflin Harcourt.
KAHNEMAN, D., Knetsch, J. L. & Thaler, R. H. (1990). Experimental tests of the endowment effect and the Coase theorem. Journal of Political Economy, 98, 1325–1348. [doi:10.1086/261737]
KEIJZER, F. (2015). Moving and sensing without input and output: early nervous systems and the origins of the animal sensorimotor organization. Biology & Philosophy, 30(3), 311–331.
KNUDSEN, T. (2003). Simon’s selection theory: Why docility evolves to breed successful altruism. Journal of Economic Psychology, 24, 229–244. [doi:10.1016/S0167-4870(02)00205-2]
LEE, J.-S., Filatova, T., Ligmann-Zielinska, A., Hassani-Mahmooei, B., Stonedahl, F., Lorscheid, I., Voinov, A., Polhill, G., Zhanli, S., & Parker, D. C. (2015). The complexities of agent-based modeling output analysis. Journal of Artificial Societies and Social Simulation, 18(4), 4: https://www.jasss.org/18/4/4.html. [doi:10.18564/jasss.2897]
MAGNANI, L. (2007). Morality in a Technological World. Knowledge as a Duty. New York: Cambridge University Press.
MARCH, J. G. (1981). Footnotes on organizational change. Administrative Science Quarterly, 26(4), 563–577. [doi:10.2307/2392340]
MENARY, R. (2010). Introduction to the special issue on 4e cognition. Phenomenological Cognitive Science, 9, 459–463.
MERTON, R. K. (1968). The Matthew effect in science: The reward and communication systems of science are considered. Science, 159(3810), 56-63. [doi:10.1126/science.159.3810.56]
MICHEL, A. A. (2007). A distributed cognition perspective on newcomers’ change processes: The management of cognitive uncertainty in two investment banks. Administrative Science Quarterly, 52(4), 507–557.
MILLER, J. H. & Page, S. E. (2007). Complex Adaptive Systems. An Introduction to Computational Models of Social Life. Princeton, NJ: Princeton University Press.
MILLER, K. D. & Lin, S.-J. (2010). Different truths in different worlds. Organization Science, 21(1), 97–114.
NEUMANN, M. & Cowley, S. J. (2016). 'Modelling social agency using diachronic cognition: Learning from the Mafia.' In D. Secchi & M. Neumann (Eds.), Agent-Based Simulation of Organizational Behavior. New Frontiers of Social Science Research. New York: Springer, pp. 289–310. [doi:10.1007/978-3-319-18153-0_14]
NEWELL, A. & Simon, H. A. (1972). Human Problem Solving. Englewood Cliffs, NJ: Prentice-Hall.
OCASIO, W. (2001). How do organizations think? In T. K. Lant & Z. Shapira (Eds.), Organizational Cognition: Computation and Interpretation, (pp. 33–49). Mahwah, NJ and London: Lawrence Erlbawm.
PEDERSEN, S. B. (2012). Interactivity in health care: bodies, values and dynamics. Language Sciences, 34(5), 532–542.
PERICO, D. H., Silva, I. J., Vilão, C. O., Homem, T. P., Destro, R. C., Tonidandel, F. & Bianchi, R. A. (2014). Hardware and software aspects of the design and assembly of a new humanoid robot for Robocup soccer. In Robotics: SBR-LARS Robotics Symposium and Robocontrol, IEEE, (pp. 73–78). Joint Conference. [doi:10.1109/SBR.LARS.Robocontrol.2014.39]
POLHILL, J. G. (2010). ODD updated. Journal of Artificial Societies and Social Simulation, 13(4), 9: https://www.jasss.org/13/4/9.html.
POLHILL, J. G., Parker, D., Brown, D. & Grimm, V. (2008). Using the ODD protocol for describing three agent-based social simulation models of land-use change. Journal of Artificial Societies and Social Simulation, 11(2), 3: https://www.jasss.org/11/2/3.html.
RITTER, F. E., Schoelles, M. J., Quigley, K. S. & Cousino-Klein, L. (2011). 'Determining the numbers of simulation runs: Treating simulations as theories by not sampling their behavior.' In L. Rothrock & S. Narayanan (Eds.), Human-in-the-loop simulations: Methods and practice. London: Springer, pp. 97–116.
SCHEIN, E. H. (1996). Culture: The missing concept in organization studies. Administrative Science Quarterly, 41, 229–240. [doi:10.2307/2393715]
SEARLE, J. R. (1980). Minds, brains, and programs. Behavioral and brain sciences, 3(3), 417–424.
SECCHI, D. (2011). Extendable rationality. Understanding decision making in organizations. New York: Springer. [doi:10.1007/978-1-4419-7542-3]
SECCHI, D. (2015). A case for agent-based model in organizational behavior and team research. Team Performance Management, 21(1/2), 37–50.
SECCHI, D. (2016). 'Boundary conditions for the emergence of ‘docility:’ An agent-based model and simulation.' In D. Secchi & M. Neumann (Eds.), Agent-Based Simulation of Organizational Behavior. New Frontiers of Social Science Research. New York: Springer, pp. 175–200. [doi:10.1007/978-3-319-18153-0_9]
SECCHI, D. (2017). Guest editorial: Agent-based models of bounded rationality. Team Performance Management, 23(1/2), 2–12.
SECCHI, D. & Adamsen, B. (2017). 'Organizational cognition: A critical perspective on the theory in use.' In S. J. Cowley & F. Vallee-Tourangeau (Eds.), Cognition Beyond the Brain: Computation, Interactivity and Human Artifice. Heidelberg: Springer, 2nd edn, pp. 305–331. [doi:10.1007/978-3-319-49115-8_15]
SECCHI, D. & Bardone, E. (2009). Super-docility in organizations. An evolutionary model. International Journal of Organization Theory and Behavior, 12(3), 339–379.
SECCHI, D. & Cowley, S. J. (2016). Organisational cognition: what it is and how it works. Presented at the European Academy of Management Annual Conference. Paris, France. In D. Secchi & M. Neumann (Eds.), Agent-Based Simulation of Organizational Behavior. New Frontiers of Social Science Research. New York: Springer, pp. 175–200.
SECCHI, D. & Gullekson, N. (2016). Individual and organizational conditions for the emergence and evolution of bandwagons. Computational and Mathematical Organization Theory, 22(1), 88–133. [doi:10.1007/s10588-015-9199-4]
SECCHI, D. & Neumann, M. (Eds.) (2016). Agent-Based Simulation of Organizational Behavior. New Frontiers of Social Science Research. New York: Springer.
SECCHI, D. & Seri, R. (2017). Controlling for ‘false negatives’ in agent-based models: A review of power analysis in organizational research. Computational and Mathematical Organization Theory, 23(1), 94–121. [doi:10.1007/s10588-016-9218-0]
SEGLEN, P. O. (1997). Why the impact factor of journals should not be used for evaluating research. British Medical Journal, 314(7079), 498.
SERI, R. & Secchi, D. (2017). 'The problem of determining the number of runs in your simulation.' In B. Edmonds & R. Meyer (Eds.), Simulating Social Complexity. A Handbook, Heidelberg: Springer, 2nd edn.
SHAPIRO, L. (2010). Embodied cognition. London: Routledge.
SIMON, H. A. (1991). Bounded rationality and organizational learning. Organization Science, 2(1), 125–134. [doi:10.1287/orsc.2.1.125]
SIMON, H. A. (1993). Altruism and economics. American Economic Review, 83(2), 156–161.
SIMON, H. A. (1997). Administrative Behavior. New York: The Free Press, 4th edn.
SMALL, H. (2004). On the shoulders of Robert Merton: Towards a normative theory of citation. Scientometrics, 60, 71–79.
STEFFENSEN, S. V. (2013). 'Human interactivity: problem-solving, solution-probing and verbal patterns in the wild.' In S. J. Cowley & F. Vallee-Tourangeau (Eds.), Cognition Beyond the Brain: Computation, Interactivity and Human Artifice. Dordrecht: Springer, pp. 195-221. [doi:10.1007/978-1-4471-5125-8_11]
THEINER, G. (2013). Writing in mind. Introduction to the special issue of AVANT. Avant, IV(2), 15–29
THOMPSON, E. (2007). Mind in Life: Biology, Phenomenology, and the Sciences of Mind. Cambridge, MA: Harvard University Press.
THOMSEN, S. E. (2016). 'How docility impacts team efficiency. An agent-based modeling approach.' In D. Secchi & M. Neumann (Eds.), Agent-Based Simulation of Organizational Behavior. New Frontiers of Social Science Research, (pp. 159–173). New York: Springer.
TOLMAN, E. C. (1932). Purposive Behavior in Animals and Men. Berkeley and Los Angeles: University of California Press.
VANCLAY, J. (2011). Impact factor: outdated artefact or stepping-stone to journal certification? Scientometrics, 92(2), 211–238.
VARELA, F. J., Thompson, E. & Rosch, E. (1991). The Embodied Mind: Cognitive Science and Human Experience.Cambridge, MA: MIT Press.
WEICK, K. E. (1993). The collapse of sensemaking in organizations: The Mann Gulch disaster. Administrative Science Quarterly, 38(4), 628–652.
WEICK, K. E. (1995). Sensemaking in Organizations. Thousand Oaks, CA: Sage, 1st edition edn.
WEICK, K. E. & Roberts, K. H. (1993). Collective mind and organizational reliability: The case of flight operations on an aircraft carrier deck. Administrative Science Quarterly, 38, 357–381.
WEICK, K. E. & Sutcliffe, K. M. (2006). Mindfulness and the quality of organizational attention. Organization Science, 17(4), 514–524. [doi:10.1287/orsc.1060.0196]
WELLS, A. (2005). Rethinking cognitive computation: Turing and the science of the mind. New York: Palgrave McMillan.
WHEELER, M. (2005). Reconstructing the cognitive world: The next step. Cambridge, MA: MIT Press.
WILENSKY, U. (1999). NetLogo. Center for Connected Learning and Computer-Based Modeling, Northwestern University, Evanston, IL.
WILSON, M. (2002). Six views of embodied cognition. Psychological Bulletin and Review, 9(4), 625–636. [doi:10.3758/BF03196322]
WILSON, R. A. (2005). Collective memory, group minds, and the extended mind thesis. Cognitive Processing, 6, 227–236.
WOODS, J. (2004). The death of argument: Fallacies in agent-based reasoning. Dordrecht: Kluwer. [doi:10.1007/978-1-4020-2712-3]