Antonio Canova (1757 – 1822). Musee du Louvre.
Introduction
"EROS" was mentioned by Rosaria Conte aloud in reply to a plea for keeping our simulated agents simple and stupid (KISS). KISS advocates starting with the simplest model, and only expand the model when this is needed to explain and understand the phenomenon of interest. However, when theories are available that describe a certain process in human behaviour, implementing these theoretical notions in the model fosters the simulation’s realism and validity of results. Enhancing Realism Of Simulation, EROS, clearly refers to including psychological realism in the agents we model. At the SIMSOC meeting in Cortona in 1997 this was debated, and later the introduction of KIDS – Keep It Descriptive Stupid (Edmonds & Moss 2005) further contributed to the discussion of how to approach certain modelling challenges. KIDS starts with modelling a certain phenomenon as straightforward as possible, taking into account the widest possible range of evidence, including qualitative data such as anecdotes and expert opinions. Simplification is only applied when and if the model and evidence justify this. In the current paper, twenty years after Rosaria called for EROS, we want to reflect on how psychological theories have been used to capture aspects of psychological reality into enhancing the models used in social simulation. The focus will mainly be at the more social-psychological theories because these are in particular relevant for the interaction between people, an aspect that is critical in social simulation. We also will highlight a few challenges and limitations we identify for modelling psychologically relevant drivers and processes into computer simulation models, and the still limited contribution of social simulation to the field of psychology. Whereas a citation analysis of JASSS demonstrates a considerable influence from psychology (and the social sciences in general) on social simulation papers, these JASSS publications seem to go quite unnoticed by the field of psychology (e.g., Squazzoni & Casnici 2013). However, considering that social simulation is increasingly being recognised as a relevant research methodology to study behavioural dynamics, there appears to be an increasing interest for applications in the more regular social scientific journals such as the European Psychologist (Conte & Giardini 2016), the Journal of Environmental Psychology (e.g. Jager & Ernst 2017) and Social Psychological and Personality Science (Jackson et al, forthcoming). Here, we also discuss the relevance of social simulation for dynamic theory development in psychology, and address how formal modelling may contribute to developing a dynamical and causal perspective on micro and macro level interactions. Such a theory development would contribute to connecting social scientific fields that study behaviour on different levels of aggregation, most notably cognitive psychology, social psychology and sociology. Considering that the field of psychology is currently still being dominated by the development of micro-level theories and is plagued by problems of replication, the sculpture of Canova fits with our ideas that enhancing realism of simulations also contributes to the awakening of the field of psychology for developing integrative and dynamic theories of behaviour that contribute to our understanding of the operation of our own species. Considering the challenges that we face as humanity, developing solid theory on behavioural dynamics appears to be an urgent research priority.
The relevance of psychology in social simulation
Social simulation proves to be a powerful tool to model individual agents, their interaction among themselves and with the environment they share. Using agent based modelling techniques allows for computer experimentation with the micro-macro relations determining the often complex social dynamics that are hard to isolate in the real world. Obviously, simulation models offer a simplified representation of the complex reality they try to study. The KISS principle stresses that Keeping It Simple Stupid contributes to the researcher’s capacity of understanding the results obtained with the models. However, at the same time we are aware of drivers and processes that are important in the context of human decision making in a social context. For example, in managing our limited cognitive resources, we often scrutinise the behaviour of similar people to identify potentially interesting behaviours. Many psychological theories address the different processes and drivers that play a role in how we learn from the experiences and opinions of other people. Including such psychological theories in social simulation models not only affects the individual agent behaviour, but also has impacts on the macro-level societal behaviour, and hence have an impact on the environment the agents live in, e.g. the availability of resources (Janssen et al. 2000). In understanding for example tipping points in social systems (Nyborg et al. 2016) where social forces flip from hampering behavioural change towards promoting it, it is therefore important to address social drivers such as conformism, anti-conformism and non-conformism as well as social learning strategies, imitation and social comparison processes. Moreover, it seems critical to address heterogeneity concerning these social drivers in the population as an important aspect of the social fabric affecting social dynamics. For example, innovation diffusion theory clarifies that innovators, opinion leaders and followers have a different perspective on social outcomes (Rogers 1993). Where innovators are more likely to experiment with new behaviours, and are hardly susceptible for the opinion of other people, opinion leaders are usually much more aware of their role in the social network. As a result, they also are likely to consider the social impacts of adopting a new behaviour. Followers are even more susceptible for social outcomes, and are only adopting new behaviour when they observe it is socially acceptable. Addressing this heterogeneity in social susceptibility is a key element in understanding how social forces affect the spreading of new behaviours through social systems (Van Eck, Jager & Leeflang 2011).
Several more generic psychological theories are available and being used to understand a variety of specific societal relevant issues such as waste reduction and recycling, energy use and food consumption, transportation behaviour, migration and acculturation, social conflict and polarisation. All these issues deal with social dynamics that develop over longer time-spans, and where the interactions between the individual behaviour at the micro-level and the societal macro-level play a central role. Hence there seems to be a valid reason for exploring the possibilities of implementing behavioural theory in agent based models to study behaviour theoretical mechanisms in a social dynamical context.
Challenges and problems in formalising psychological theory
Social simulation offers a computational tool to explore micro-macro relations in social systems, and beyond, e.g. socio-economical and socio-ecological systems. Computational experiments make it possible to experiment with large heterogeneous populations over long periods of time to systematically study how certain assumptions on behaviour have an impact on social dynamics and beyond. A classic example would be the Schelling model (1971), demonstrating how a slight preference of individuals (micro level) for being in a majority position results in a complete segregation (macro-level), which contributed to our understanding of the social dynamics of e.g. ethnic segregation.
Key to the explanatory power of such simulation experiments are the assumptions used in formalising the micro-level behavioural rules of the agents. For example, it is possible to formalise simulated agents as outcome maximising decision makers with perfect knowledge of their environment. This maximising assumption confronts the modeller with numerous challenges, such as the degree to which future outcomes are being considered (time discounting), and how agents deal with uncertainties concerning the system they are interacting with (e.g., natural environment, economy, weather, climate) and the behaviour of other agents (social reasoning). Whereas from a rational actor approach these questions open a set of very difficult to answer questions, at the same time psychology offers several theories on the mechanisms people use to process information and make decisions in a variety of situations. Hence in making more realistic assumptions on the micro-level behaviour of agents it is scientifically sound to use psychological theories as the basis for the implementation of agent rules.
Implementing behavioural theory in agent rules confronts the modeller with a number of challenges (Schlüter et al 2017; Jager & Ernst 2017). The first challenge deals with the identification of relevant theories on human cognition and (social) decision-making. There exists an abundance of theories on behaviour from social sciences such as economics, psychology, anthropology, sociology and political science. These theories range from being very detailed and specific, such as the role of emotion (anger) in the social punishment in social dilemmas (Hopfensitz & Reuben 2009) to very generic theories such as the Theory of Planned Behaviour (Ajzen 1991). The theories thus are addressing different levels of aggregation and are often overlapping (or nested), making it difficult to identify and select the most appropriate theory to implement in modelling a specific behavioural dynamic.
The second challenge deals with the lack of formalisation of many social scientific theories. Whereas most behavioural theories are based on statistical relations (correlations), in using them in agent-based models we have to make these theories computational (causal mechanisms). This implies that we have to translate the theoretical notions into causal descriptions that allow for computational modelling (Ernst 2010; Jager & Ernst 2017). One problem here is the descriptive levels of theories confront the modellers with logical gaps that must be filled in order to make a simulation work. Modellers need to make assumptions to fill such gaps (Sawyer 2004). A next problem is that most behavioural theories start with behavioural drivers and end with behaviour. In computational models, behaviour results in outcomes that serve as drivers of the behaviour in a next step. Hence, in making theories computable, this causal loop has to be closed. The statistical grounding of many theories provides us with another challenge. Whereas statistical grounding is based on average effect sizes, we need to address population heterogeneity to model social dynamics.
It is understandable that the rational choice model is often used in modelling behaviour because it gives some clear and relatively easy to implement guidelines. However, at the same time, we are aware of the relevance of more complicated processes in human cognition and decision making that are important in studying social dynamics. Therefore, in the following we will provide an overview of a number of social simulation applications that use social scientific theory in studying different social dynamical phenomena. This overview will be far from complete, but will serve to give insight in a few theories that have been successfully implemented to address certain social phenomena.
Examples of applications of social-psychological theory in social simulation
Recently, social simulation applications that implement psychological, sociological and behavioural theory have been increasing. In this section, we want to briefly discuss a number of simulation applications as an example of some relevant different theories being implemented in different contexts. It is not our intention to describe in detail how the theories have been implemented. Rather our exhibit is meant to provide the interested reader with an overview of existing applications, and we refer the reader to the original publications for getting more detailed information on the precise implementation. We will organise this exhibit around a number of theoretical approaches and theories.
Theory of normative conduct
One of the key drivers of behaviour that are important in understanding social influence are social norms. Hence in the field of social psychology, abundant research has been conducted on how norms operate and influence human behaviour (Cialdini & Goldstein 2004). Basically, compliance to norms serves three goals. First, accuracy refers to the goal of being accurate about vital facts. Second, affiliation refers to the goal of having meaningful relations with others. Third, maintaining a positive self-concept by keeping a consistent set of values. Cialdini, Kallgren and Reno (1991) make a distinction between three types of norms. Descriptive norms guide behaviour via the perception of how most others would behave. Injunctive norms guide behaviour via the perception of how most others would approve/disapprove of a person's conduct. Finally, personal norms guide the behaviour via the perception of how a person would approve/disapprove of his own conduct.
Within the context of social simulation, the modelling of norms is a critical issue, as normative influence is one of the key mechanisms of social influence. A recent overview on modelling norms has been provided by Elsenbroich & Gilbert (2014). A very simple implementation of normative influence can be based on a weighting function where a distinction is being made between personal and social outcomes of performing a particular behaviour. For example, Delre et al (2007) implement the impacts of norms on the diffusion of an innovation if at least one of their neighbours have already adopted the product. To do so, they make a distinction between individual preference and social influence, and define a simple weighted utility: \(U_ij = \beta_ij* x_ij + (1 - (\beta_ij) * y_ij\). In this formula, \(y_ij\) refers to the social need, and the satisfaction of this by adopting product \(j\) by agent \(i\) depends on the proportion of neighbouring agents adopting product \(j\) as well. Obviously, the value of \(\beta\) indicates the relative importance of the norm, where agents having a \(\beta\) value close to 0 are mainly focussing on the norm, whereas agents having a \(\beta\) close to 1 are only looking at the product characteristics. In the process of innovation diffusion this distinction proves to be critical.
The social-cognitive representation of norms in agents by Conte and Castelfranchi (1995), Conte and Paolucci (2001, 2002) and Conte et al. (2013) provide a theoretically elaborated modelling approach. This approach has developed a number of models that focus on particular social phenomena such as social learning, norms (Conte & Paolucci 2001), and reputation (Conte and Paolucci 2002) and relate these to the underlying cognitive basis. An important process is the internalisation of norms, which is being addressed by the framework EMIL-I-A (EMIL Internalizer Agent, Conte et al. 2013). After agents have been exposed to the normative behaviours of others and to their (explicit or implicit) normative requests, agents potentially acquire those normative beliefs. The normative beliefs are based on three components, respectively (1) the basic belief that there is a norm, (2) the belief that the norm applies to the agent (or the group the agent belongs to), and (3) the belief that compliance and violations of the norm will be rewarded or sanctioned. Before internalization, an agent can engage in a cost-benefit analysis in deciding to comply with the norm. However, once internalized, the agent will comply to the norm independently of external sanctions and rewards.
The examples above indicate that norms can be modelled with different levels of detail. If one is exploring the cognitive dynamics of norm development, a theoretically elaborated model is required. However, if just a simple social influence should be modelled in e.g. an applied model, a much simpler implementation can be justified. Moreover, additional issues can be relevant in modelling norms, such as who is interacting with who, which relates to the impact of similarity and associated opinion dynamics that will be discussed below.
Goal Frame Theory
While much work on norms does not really address the change of norms as an effect of observed behaviour, Cialdini, Reno and Kallgren (1990) showed that people are very susceptible to the littering behaviour of other people. Goal Frame Theory explicitly addresses the change of the importance of different goals as a function of public (dis)respect for a norm (Lindenberg & Steg 2007). As such, the normative influence is placed within a wider motivational framework, and the importance of the goals are subject to change. Three goal frames are distinguished by Lindenberg & Steg (2007): the hedonic goal "to feel better right now", the gain goal "to guard and improve one’s resources", and the normative goal "to act appropriately". In a littering context the hedonic motive would focus on getting rid of a piece of litter, e.g. by throwing it on the street or in a bin, whereas the normative motive would focus on keeping the environment clean, e.g. by throwing litter in a bin or to keep it with you.
The Goal Frame Theory has been implemented in the context of household appliances purchase and replacement (Polhill 2015) and in a smart metering context (Vasiljevska et al. 2017). These applications used a fixed setting for the goals. Rangoni and Jager (2017) formalised changing weights of the different goals, and addressed the dynamics of norms as depending on the degree of littering in the agent’s environment. This approach “closed the loop” in the sense that collective behaviour influences individual littering behaviour, which feeds forward to agent’s decision to litter in a next time-step. In the model an agent \(i\) is initialized having a norm goal weight \(N_i\) (0, 100) and a hedonic goal (inverse). The agent \(i\) looks at the tiles surrounding him. \(L_{it}\) (0, 100) is defined as the proportion of tiles holding one or more units of litter at time \(t\). A threshold \(TN_i\)T (0, 100) is defined for agent \(i\), representing his tolerance to litter. Hence, agent i will regard the environment as clean if \(TN_i>L_{it}\). Agent \(i\) updates his current normative goal weight \(N_{it}\). For \(TN_iTN_i<\) he will regard the norm as disrespected and his normative goal weight will be weakened. Hence, \(N_{it}=N_{it-1}-N_{it}\ast R\) where \(R\) is the reinforcement magnitude, being the same for all agents. On the other hand, for \(TN_i>L_{it}\) agent \(I\) will regard the norm as respected and his normative goal weight will be reinforced. Hence, \(N_{it}=N_{it-1}+N_{it}\ast R\)
While it was possible to parameterise the simulation model to replicate the empirical results of a littering field experiment (Keizer, Lindenberg & Steg 2011), the model used had to make some crude assumptions. In particular, the function that described magnitude of the reinforcement was not based on empirical data because of a lack of experimental data on that. Whereas the principles of reinforcement learning are rooted in behaviourist psychology, which originates from principles of classical and operant conditioning (Pavlov 1927; Skinner 1953), not much is known from specific functions that can be used to parameterise the reinforcement process. Next, also no data is available concerning the heterogeneity in the population concerning the existence of specific thresholds for litter, and it can be assumed that these thresholds differ depending on the situation (e.g. own garden versus festival area). As such, the implementation of dynamic norms confronts the modeller with a number of unknowns that requires the use of crude assumptions.
Similarity Theory
An important finding in studying interpersonal influence and attraction is the role of similarity. This is often expressed in popular language as “birds of a feather flock together”. Theory on similarity/attraction has been proposed by Festinger (1954), Berscheid & Walster (1969) and Byrne (1971). The basic idea is that people find it pleasant to have similar attitudes as other people in their environment. This will contribute to a feeling of group belongingness and safety, and prevent people from having a fear of social exclusion. Furthermore, the concept of homophily is being used in addressing congruency or similarity on attributes such as demographic variables, beliefs and values (e.g., Infante, Rancer & Womack 1997). Additionally the behaviour of similar people is more likely to be copied because it falls in the range of a person's possibilities (comparable abilities/resources). Hence, people with a moderate income are acquiring more personal relevant information from observing the behaviour of about equal income others than from observing much poorer or richer people.
A first implementation of similarity theory has been done by Schelling (1971), who demonstrated that in an ethnically mixed society, where the individuals prefer merely not to be in a minority in their local neighbourhood, a completely segregated society emerges as a result. Schelling’s social computational model was one of the first where a certain preference for being in the neighbourhood of similar others was implemented.
The similarity principle is widely being used in social simulation as a mechanism to regulate the interaction between agents. A critical issue here is the factors that are being used for determining the similarity between agents. One possibility is using one or several fixed traits of agents to determine the distance between them. This can be used to model e.g. similarity on e.g. ethnicity (Schelling 1971) or calculate a Euclidian distance between agents based on several traits such as age, spatial location and gender. This allows creating a fixed similarity based network of interacting agents. Another possibility is to make the probability of two agents interacting dependent on their similarity. This produces a network where in principle all agents can interact, but the more similar the agent, the more likely they interact.
Instead of using fixed factors in determining the similarity in the beginning of the simulation, in some studies it may be relevant to make similarity a dynamic factor and allow for networks to change. For example, when the actions of agents matter in determining their relevance for social comparison, agents may be equipped with a mechanism to (continuously) follow the similarity of other agents. For example, Speelman (2014) used a similarity function for modelling the chance of farmers to interact on their physical proximity, the crop they were growing and the income they generated. As a result, changing of crop and change in income would also affect the chances of farmer agents to interact. This makes sense concerning the relevance of comparisons, as it is more relevant for a farmer to exchange information with farmers growing the same crop and having about the same financial means.
Using concepts of similarity theory in static or dynamic ways contributes to developing more psychologically relevant network structures between agents. Instead of using random networks, small-world networks (e.g. Watts 1999), scale-free networks (e.g. Barabasi & Albert 1999), and apply principles of preferential attachments based on the existing number of links (e.g. Amaral et al 2000), it is also possible to develop both static and dynamic networks using insights on similarity theory. However, as discussed in the above, there are different ways of implementing this, and existing theories on similarity principles only address the micro level, and do not provide substantive evidence on the relations between different types of similarity and the resulting network structures. For the social simulator it is a challenge to reflect on what dimensions of similarity are relevant in exploring the behavioural dynamics in a particular context. In some contexts, more simple and computational efficient implementations can be used than in other contexts. Ultimately, a thorough understanding of the empirical case is needed to make a grounded decision on how to implement principles of similarity in modelling the interactions between agents.
Social Judgement Theory and opinion dynamics
Opinion dynamics is a very relevant domain that is being studied with social simulation models. Much literature and simulation models address the attraction between people and their opinions (e.g., Hegselmann & Krause 2002). The field of opinion dynamics is a specific case where similarity effects play an important role. People are being attracted to other people having similar beliefs and opinions, and adjust their beliefs and opinions to match them. However, also the opposite effect may happen, where people contrast themselves with people having significantly different beliefs and opinions. In addressing the impact of similarities/differences in opinions on the development of these opinions, Sherif and Hovland (1961) propose a theory that describes how individuals change their position after being confronted with another position. Social Judgement Theory states that if people’s advocated positions are relatively close, an assimilation effect will occur. If the positions are far apart, a contrast effect will occur, driving their positions apart. The latter effect seems to be very important in understanding effects of polarisation. However, the contrast effect often seems to play a minor role in comparison to the positive attitude shifts following positive interactions (Takács et al. 2016). In modelling acculturation processes such as assimilation, integration, separation and marginalisation (Berry 1984), contract effects may be relevant in understanding the lack of further interactions between groups after some first negative interactions.
An implementation of Social Judgement Theory (SJT) has been done by Jager and Amblard (2005). In simulating opinion dynamics, they formalised two thresholds. If an opinion difference is smaller than u, and assimilation effect will occur (lattitude of acceptance). If a difference is larger than \(t\), a contrast effect will occur (lattitude of rejectance). If the difference is between \(u\) and \(t\) no change will happen (lattitude of non-commitment. In formula:
$$ \begin{split} \text{If}\; |x_i-x_j|<u_i\;\text{ then }\; dx_i=\mu \cdot (x_j-x_i)\\ \text{If}\; |x_i-x_j|>t_i\; \text{ then }\; dx_i=\mu\cdot\; (x_i-x_j)\\ \end{split} $$ |
This formalisation opens the possibility of experimenting with heterogeneity in the population concerning their threshold levels and their impact on the resulting opinion dynamics. However, the argumentative part of this model is very simple, addressing only a one-dimensional opinion, and having a very simple rule for changing the opinion. The multidimensionality of opinions, and the impact of e.g. reputation and expertise of the agents are not included in this formalisation. Stefanelli and Seidl (2017) address the opinions concerning deep geological nuclear waste repositories in Switzerland, where dimensions of safety and economic benefits are included. More elaborate models addressing processes of persuasion are proposed by e.g. Dignum et al (2001) and Dykstra et al (2015), who include processes of reasoning on when to engage in an interaction and the degree of persuasive power. Salzarulo (2006) implemented the principle of Self Categorisation Theory (Turner et al. 1987) in simulating processes of opinion dynamics. Considering that the field of opinion dynamics is a very relevant research domain it seems important to further explore how insights and theories originating from smaller scale laboratory experiments can be implemented and tested in a social simulation context.
Elaboration Likelihood Model
A well known theory on human persuasion processes is the Elaboration Likelihood Model of Petty and Cacioppo (1986). They make a distinction between central processing, which deals with the processing of arguments and information, versus peripheral processing, which is based on the processing of cues rather than arguments and information. Cues here refer to source effects, such as the respect a person has, the charisma of e.g. politicians and the trust one has in the knowledge of friends and acquaintances. When a person is not motivated or capable to process information, often these social cues will serve as a driver of opinion formation and change.
In implementing this process in a simulation model, Jager and Amblard (2007) expanded the formalisation of the Social Judgement Theory by adding a second opinion dimension. The basic idea was that people like to have similar opinions as people they interact with (Festinger 1954). Hence when people assimilate on an important opinion dimension (central processing), it is likely that they will also assimilate unimportant - and unrelated - opinions following peripheral processing. Even if these unimportant opinions are far apart, due to the assimilation effect on the basis of experienced similarity on an important dimension, the assimilation effect will occur here as well. Formalised for an important opinion A and an unimportant opinion B this results in the following formalisation:
$$ \begin{split} \text{If}\; |xA_i-xA_j|<u_i\; \text{then}\; dxA_i=\mu\cdot (xA_j-xA_i)\;\text{, and }\;dxB_i=\mu\cdot (xB_j-xB_i)\\ \text{If}\; |xA_i-xA_j|>t_i\; \text{ then }\; dxA_i=\mu\cdot (xA_i-xA_j)\;\text{, and }\;dxB_i=\mu\cdot (xB_i-xB_j) \end{split} $$ |
Here the change on the unimportant dimension B is completely dependent on the change on the important dimension A. This formalisation is very simple, and does not account for the empirical fact that attitude changes following peripheral processing are usually not persistent. Other issues that appear to be relevant in this approach are the parameterisation of how much opinion change occurs (\(\mu\)) as a function of the strength of arguments and the strength of peripheral cues. Whereas empirical effects have been found, these do not provide clear indications for parameterising \(μ\) in a valid manner.
A theoretically more elaborated application of the Elaboration Likelihood Model is presented by Mosler et al. (2001). They formalised the ELM in an agent-based model and compared simulated individuals' reactions to stimuli with the experimental and statistical observed reactions of real participants in experiments. Their model describes a systems theoretical description of the elaboration Likelihood model, and in formulas the transitional dynamics are being defined. Hence, the model is composed of functional blocks, such as elaboration motivation, ability and likelihood, bias position and attitude. The input received by every block is being processed within the block following an algorithm, and generates output towards another block. The simulation model was tested against empirical studies that had been published before by other researchers. Replication of the results was considered a successful implementation. A difficulty in the implementation was that the empirical data were not complete. For example, no data were available on the initial attitude of the respondents, and hence assumptions had to be made concerning the agents’ properties.
The results of the experiments are interesting in the sense that dynamical processes of attitude change are generated, demonstrating for example how strong versus weak arguments may influence attitudes at different times during the process, and agents may switch between central and peripheral processing. As such Mosler et al. (2001) provide an interesting example of how a psychological theory can be translated in a dynamical context using social simulation.
These examples demonstrate that the more close a modeller gets to the original studies, the more assumptions have to be made concerning the precise implementation and settings of the model. The results are important because they address how changes in beliefs and opinions spread in a social setting depending on the elaboration processes agents engage in as a function of their motivation, cognitive capacity and appeal of the source.
Theory of Planned Behaviour (TPB)
The Theory of Planned Behaviour (Ajzen 1991) brings together a number of components in predicting human behaviour. Behaviour (action) is considered to be driven by a behavioural intention, which in turn is affected by a person’s attitude, the social norm associated with the behaviour in question and the behavioural control. The attitude towards performing a behaviour is basically a weighted multi attribute subjective utility function, where attitudes concerning the evaluation of behavioural outcomes are weighted in a total attitude score. The social norm combines the perceived behaviour of relevant other people and the motivation to comply with this behaviour. The behaviour of relevant other people is closely related to the earlier discussed theory of normative conduct, goal frame theory and similarity theory. The motivation to comply refers to personal characteristics, and may be used to make a distinction between conformists, nonconformists and anti-conformists. Finally, behavioural control is an important driver, addressing the (perceived) possibility to perform the behaviour. This refers to possible financial, technical and physical barriers to perform a particular behaviour.
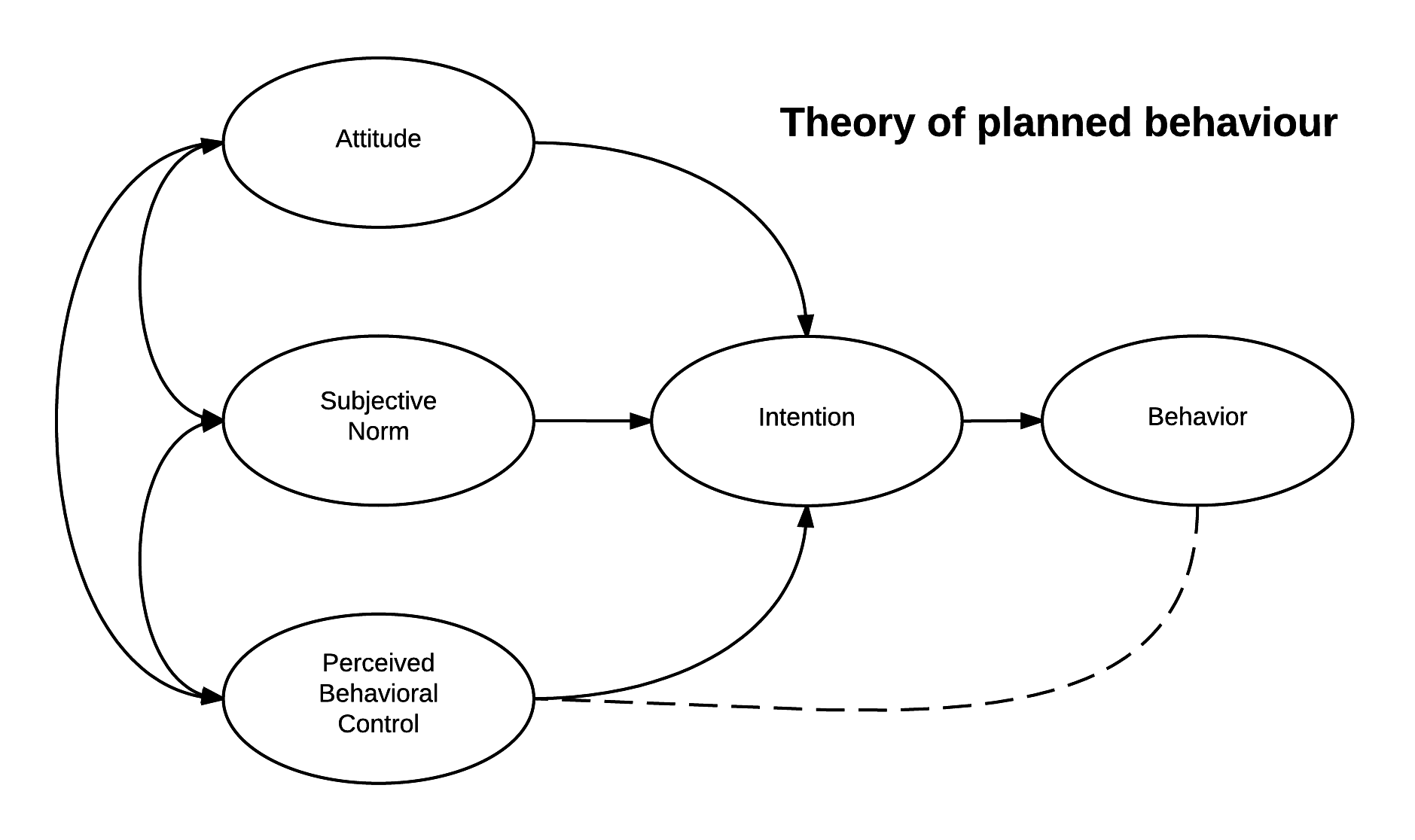
Using TPB in social simulation models brings this framework in a dynamical context, where the behaviour of one agent serves as the (normative or informative) input for another agent later. Moreover, in principle a social simulation model would allow for formalising (groups of) agents that differ concerning e.g. the relative importance of beliefs, susceptibility for norms and control beliefs.
In the context of innovation diffusion, the TPB allows for translating empirical data on the beliefs concerning the different outcomes of behaviours/technical adoptions into the valuation function of agents. For example, Mosler et al. (2001) used the TPB to model in studying the dynamics of interventions aimed at recycling, composting and re-use of materials. Empirical data collected from a project in Cuba were being used to parameterise the model. The simulation experiments showed the potential efficacy of different interventions, including the impact of social dynamics.
Schwarz & Ernst (2009) studied the diffusion of water-saving shower-heads, a toilet flush and a rain harvesting system given scenarios of future droughts and different policies. Empirical data were being collected and used to construct the agents’ attitude, social norm and perceived behavioural control based on data on environmental performance, ease of use, saving of costs, compatibility with existing infrastructure and investment costs. Whereas in most empirical studies using the TPB framework the focus is on average statistical effects, in social simulation there is the important option to address heterogeneity in the agents. Schwarz & Ernst addressed this using a segmentation strategy based on socio-cultural characteristics of groups, e.g. established conservatives, escapists and traditionalist. The results demonstrated that attitudes and perceived behavioural control had an important impact on the adoption of water saving innovations, whereas communication in social networks was less important. Their study was capable of generating policy scenarios demonstrating how water-saving innovations would spread through different socioeconomic groups throughout the country of Germany.
The TPB has inspired several modellers in developing social simulation models. Early applications are for example addressing resource management (Ernst 1998) and household waste management (Tucker & Smith 1999). The TPB is currently being used in a variety of simulation models addressing different topics, such as political attitudes and voting behaviour (Kottonau & Pahl-Wostl 2004), alcohol consumption dynamics (Moyo et al. 2015), Child Maltreatment prevention (Hu & Keller 2015), the use of smart energy metering in Europe (Vasiljevska et al. 2017) and waste management (Scalco et al. 2017).
Many social simulations have also employed the BDI (Belief Desire Intention) approach in formalising agents’ decision making (see for a review e.g. Balke & Gilbert 2014). This approach can be interpreted as a way of formalising the attitude-intention relation within the TPB context. However, the BDI approach does not include social interaction and normative influence, which are important aspects in behavioural dynamics.
Integrated models
The Theory of Planned Behaviour already offers a more integrated model compared to the more specific processes addressed by norms, similarity and persuasion models. As such, principles of e.g. similarity and norms can be logically applied in a TPB framework. Several researchers have been working on developing modelling architectures that incorporate different behavioural mechanisms and principles. These integrated models are often used to model a variety of behaviours and decision-making processes in a wider or multi-layered context.
Brousmiche et al (2016) address cognition and attitudes in a model of opinion dynamics. They propose Polias, a modelling framework that is mainly based on Fazio’s (2007) cognitive model of attitude formation. Polias is grounded on different insights from social psychology, and it combines ideas on attitude formation and change. In Polias attitudes are composed of a cognitive and emotional component. They are represented as memory associations between evaluations and the social object with varying accessibilities. Information can be obtained through direct observation, communication in the community following a Small World network structure (Watts 1999), and actor specific information (informative strategy). Communication results in belief revision, which is relevant to study opinion dynamics. Polias is a generic model of attitude formation, and could be applied to any application domain. In Brousmiche et al. (2016) the model is being applied to study the attitude impact of French forces conducting stabilization operations in Afghanistan.
Another example of a more integrated psychological model is the InnoMind simulation model (Schröder & Wolf 2017). InnoMind is based on theories of emotional cognition from cognitive science, theories of attitudes and persuasion from social psychology, and theories of social networks from sociology. Agents’ attitudes are represented as constraint-satisfaction networks. InnoMind uses artificial neural networks to model individual attitudes as parallel constraint satisfaction. The nodes in the neural network represent single concepts, and the links between them represent relationships between the concepts. The activation of nodes corresponds with cognitive salience. The attitude change following interaction can follow a more central route or a peripheral route, as discussed earlier in the section on the Elaboration Likelihood Model. The communication within the simulated society is based on an artificial social network. This network is based on geographic proximity of agents, the size of agents’ egocentric social networks, which may vary depending personality, and on similarity concerning socio-demographic variables. Schröder & Wolf (2017) use empirical data from inhabitants of Berlin to study the attitude dynamics on car-sharing in the city of Berlin. The model offers possibilities to explore the potential attitude dynamics as a consequence of different campaigns to promote car-sharing.
In the context of land use change the FEARLUS framework (Polhill et al. 2001; Gotts & Polhill 2009) addresses different aggregation layers. Land use is being affected by the biophysical attributes of the land, the decisions that are being made on the land use, the ownership structure of the land and finally the climate and economy. Concerning the behaviour of the agents, the decisions on land-use address farmers’ decision making in this multi-layered environment. The agents can engage in satisficing, imitation, and innovation. Which of these strategies is being used depends on both the agent characteristics as their (economic) performance. For example, the agent’s aspiration level indicates how satisfied it is with a certain yield. When satisfied, the agent will continue to use the land as before, which refers to habitual behaviour. Obviously, this yield is partly determined by climatic and economic circumstances, and hence both personal and situational factors come together in the agents’ decision-making. When the agent is not satisfied, it can either engage in imitation of innovation, depending on the personal settings of the agent. This setting refers back to the social susceptibility in TPB and normative influence. Imitation can be formalised in different ways, e.g. simple imitation based on a weighted random choice of all neighbours, or yield-based imitation including the success as a decisive factor.
The Consumat approach (Jager 2000) is an example of a framework that offers a generic conceptual framework that combines and connects different decision strategies such as habitual behaviour, imitation, social learning and deliberate decision-making. A recent application addresses the diffusion of hybrid and full-electric cars (Kangur et al. 2017). In this simulation model, data of 1795 respondents were used to parameterise 1795 agents. Using detailed data on driving behaviour, attitudes towards charging speed, range, environmental concern, social susceptibility, involvement with cars, preferences for models and the like an artificial population was constructed. Experiments allowed for testing effects of technical innovation and supportive (financial) policies. Especially in the context of innovation diffusion such integrated models appear to be capable of capturing how behavioural change spreads in a context where habits play an important role.
Challenges ahead
In the previous sections, a number of applications were discussed of implementations of psychological theory in social simulation. These applications demonstrate that EROS - enhancing realism of simulation - is supported by the implementation of different psychological theories. The above mentioned listing of applications is not meant to be a complete review of all possible applications, and is biased towards the different projects the author was working on earlier, but the key message is that there is a clear case for implementing psychological theory in social simulation model to improve the realism of the behavioural dynamics we are interested in to explore.
A first insight is that just as in empirical experimental social scientific research, also within social simulation research several theories are being implemented independently. These applications are relevant to study how the insights from the theories would operate in a dynamical context. A good example here is the work of Conte (e.g., Conte & Giardini 2016), implementing rich ideas from cognitive social science in social simulation models to improve our thinking about the propagation of norms through society. At this level we may consider psychological grounded social simulation research as contributions towards the development of behaviour dynamical theories.
A second insight is that there is an increased interest for integrating behaviour theoretical insights in a modelling framework. Specifically when processes of behavioural change are the topic of interest, be it farmers’ choice behaviour, recycling behaviour or citizens’ future mobility, the aforementioned examples indicate that the integration of different psychological mechanisms in a modelling framework contributes to the development of policy relevant models. Because the psychological drivers and dynamics of behaviour are included in these models, they can address social psychological responses to policy that go beyond the capacity of traditional economic and spatial models. Moreover, they offer the possibility to study impacts of e.g. informational campaigns, and include the opinion dynamics as second order effects of informational campaigns. At this level of modelling we see glimpses of a more integrated dynamical theory of human behaviour emerging, closing the gap between (social) psychology at the micro-level and sociology at the macro-level, allowing to conduct experiments on more realistically behaving artificial populations.
In both types of implementation, the researcher will by definition run into the limitations concerning our knowledge on how various psychological processes work. Whereas modelling human behaviour in computer models forces the researcher to develop running code, and hence make inferences on the causal mechanisms for the simulated agent, the psychological theories are not formalised at this level. Currently, we see social simulation increasingly being used by psychologists because it opens new possibilities to do research after how we operate in groups, and how we as a species interact with our natural environment. The more we are capable of implementing behavioural theory in computer coded models, the more we are capable of simulating relevant behavioural processes that inform us about the dynamics of our own behaviour. Hence, social simulation opens the possibility to study the dynamics of behaviour, and provides a platform to develop dynamical social psychological and sociological theory. Moreover, the social simulation of human behaviour in more realistic settings forces the modeller to implement different psychological mechanisms in an integrated model. As such, the formalisation of theory requires making causal links between different mechanisms (e.g. similarity and attitudes and opinion dynamics), and thus contributes to the development of more integrated or meta-behavioural theory.
In further developing a more integrated theory of behaviour, or perhaps less ambitious, an integrated framework for modelling human behaviour, two challenges that seem to be relevant are the modelling of human needs as the drivers of behaviour, and modelling more elaborate cognitive processes to better represent learning and decision-making.
Modelling human needs is a complicated challenge for social simulation, as there exists a relation between different brain systems, their evolutionary background and associated needs (e.g.,Deci & Ryan 2000). Needs refer to the motivation all living creatures share as a drive to maintain or improve the well-being of the organism. Whereas for plants the needs seem to be relatively simple, more developed species may have different needs, and humans are a species where needs have evolved into a complicated set of drives that sometimes conflict with one another. Whereas most simulated agents have a goal they aspire for, and sometimes even multiple, sometimes conflicting goals, there are no agent architectures that capture the different needs that people have, their specific time-dimensionality (e.g. short term like thirst versus long-term like self-actualisation) and their activation (focus) in combination. Yet different needs are implicitly or explicitly modelled in agents, ranging from simple needs addressing food, to social needs that are quite common in social simulation models, to complex needs such as self-actualisation. Concerning the modelling of a more complex need such as self-actualisation, the simulation model of Heise (2013) addressing small group behaviour is a nice example. In this model, affect-control theory (MacKinnon 1994) is being used to model self-actualisation as a more-dimensional (Evaluation, Potency, and Activity) affective meaning of behaviour. However, developing a framework that addresses e.g. Maslow’s needs pyramid (1954) or the lesser-known taxonomies proposed by e.g. Max-Neef (1992) and Kenrick et al. (2010) remains a challenge in simulating the multiple behavioural drivers of human behaviour.
Modelling cognition is another big challenge in social simulation, and some interesting examples have been discussed in this paper such as the work of Conte et al. (2013), Brousmiche et al. (2016) and Schröder & Wolf (2017). These examples bring a richer cognitive modelling perspective within the context of simulating social interaction. The field of cognitive modelling is currently mainly oriented on modelling a single actor, and these cognitive-social models are an important step in bringing cognitive modelling into a social context. Looking at the field of cognitive modelling, a main distinction can be made between rule-based, connectionist, and Bayesian approaches (see e.g., Thagard 2012) for an overview. In particular, the advances in the connectionist approach, where cognitive processes are modelled at the neural level are impressive. With computer models mimicking dynamic neural networks, it is possible to simulate these processes on a biological level. Models composed of millions of simulated neurons are being used to simulated complex cognitive processes such as pattern recognition and associated actions (e.g., Eliasmith 2012). However, realizing the vast complexity of the many different interacting brain systems and regions, and the many different chemical-electric processes that have been identified, building an artificial but realistically functioning brain that can be used in a social simulation context to simulate human society seems at least a very distant goal. Whereas much research is focusing on simulating a human brain, for example the EU Flagship Human Brain Project endowed with a funding of a billion euro for more than 100 institutions (https://www.humanbrainproject.eu/en/), problems remain in replicating a single brain because of the incompatibility of many data on the human brain.
Simpler, but still rather complex cognitive models have been developed to simulate human cognition, such as SOAR (Wray & Jones 2006; Laird, Newell & Rosenbloom 1987), ACT-R (Anderson et al. 2004; Anderson 1983), and CLARION (Sun 2006, 1997). Whereas these architectures address individual cognition, in particular Sun (2012) advocates the importance of connecting cognitive models to social simulation models.
Whereas a stronger collaboration, and possible assimilation of social simulators and behavioural and cognitive theorists would certainly contribute to the further development of more theoretically realistic and sound simulation models, we should bear in mind that there is a fundamental difficulty in quantifying behavioural principles into simulation models. We thus have to keep in mind the quote of Box (1979), stating that “all models are wrong, but some are useful”. Just as the metaphors of humans being like steam engines with subconscious pressures and the need to let of steam (e.g. Freud), or the brain operating like a telephone exchange (behaviourists), the computer metaphor that we use today does not capture how human drives and cognition operate (e.g. Chemero 2013). Hence, while it is tempting to model a memory and the process as decision-making as an information storage system (memory) and a rule based system for selecting a behaviour (computing algorithms), this neglects for example the importance of subconscious processes (gut feeling) that appear to be highly relevant in human perception, evaluation, motivation and behaviour, (e.g., Dijksterhuis et al. 2006; Bargh & Morsella 2008). Whereas experiments for example demonstrate better decision-making in complex situations when people process subconsciously, the underlying cognitive processes are hard to access and difficult to formalise. In a same vein, differences between forward looking (imaginative) versus backwards (experience, memory) thinking that seem to be important in society (e.g., Fortunato & Furey 2012), remain largely unexplored in social simulation contexts because these would require very elaborate cognitive models.
Implementing human cognition and behaviour in social simulation models ultimately remains a translation task of a wide variety of psychological processes into binary computer code, where by definition information is lost. Hence, modesty should be upheld concerning the realism of the models we develop, because often we do not know the fundamental limitations of using the computer metaphor. Yet, as the papers discussed here demonstrate, progress is being made in modelling behavioural dynamics using cognitive, social psychological and sociological theory. Whereas this computer modelling of human behaviour and cognition is still in its infancy, and huge challenges are ahead of us in developing a better understanding of how we as a species operate on Earth, important steps are made by formalising these theories in dynamical contexts. With a sufficient dose of imagination this development of a dynamical psychology driven by social simulation may be interpreted as Psyche being waked up by the kiss of Eros.
Acknowledgements
Thanks to the many colleagues who have been working and still are working on the implementation of psychological theory in social simulation models. Thanks to two anonymous reviewers who pinpointed some blind spots in an earlier version and provided valuable suggestions for improvement. A special thanks goes to Bruce Edmonds for the initial discussions leading towards the writing of this paper. And finally a salute to Rosaria Conte. Her passion for life and science will be remembered and her contributions will inspire future generationsReferences
AJZEN, I. (1991). The theory of planned behavior. Organizational Behavior and Human Decision Processes, 50, 179-211. [doi:10.1016/0749-5978(91)90020-T]
AMARAL, L.A.N, Scala, A., Barthemely, M., and Stanley, H.E. (2000). Classes of small-world networks. Proceedings of the National Academy of Sciences USA, 97, 11149-11152. [doi:10.1073/pnas.200327197]
ANDERSON, J. R. (1983). The architecture of cognition. Cambridge, MA: Harvard University Press.
ANDERSON, J. R., D. Bothell, M. D. Byrne, S. Douglass, C. Lebiere & Y. Qin. (2004). An integrated theory of mind. Psychological Review, 111(4), 1036–1060. [doi:10.1037/0033-295X.111.4.1036]
BALKE, T., and Gilbert, N., (2014). How Do Agents Make Decisions? A Survey. Journal of Artificial Societies and Social Simulation, 17 (4), 13: https://www.jasss.org/17/4/13.html. [doi:10.18564/jasss.2687]
BARABASI, A.L., and Albert, R., (1999). Emergence of Scaling in Random Networks. Science, 286: 509-512. [doi:10.1126/science.286.5439.509]
BARGH, J. A., & Morsella, E. (2008). The Unconscious Mind. Perspectives on Psychological Science: A Journal of the Association for Psychological Science, 3(1), 73–79. [doi:10.1111/j.1745-6916.2008.00064.x]
BERRY, J.W. (1984). 'Cultural Relations in Plural Societies: Alternatives to Segregation and Their Sociopsychological Implications'. In Miller, N., Brewer, M. (eds.), Groups in Contact. Academic Press, New York. [doi:10.1016/b978-0-12-497780-8.50008-5]
BERSCHEID, E, and Walster, E.H. (1969). Rewards Others Provide: Similarity. Interpersonal Attraction, 69-91. Reading, MA: Addison-Wesley.
BOX, G. E. P. (1979). 'Robustness in the strategy of scientific model building'. In: R.L. Launer & G.N. Wilkinson, (Eds.). Robustness in Statistics. Academic Press, 201–236.
BROUSMICHE, K.L, Kant, J.D., Sabouret, N. and Prenot-Guinard, F., (2016). From Beliefs to Attitudes: Polias, a Model of Attitude Dynamics Based on Cognitive Modeling and Field Data. Journal of Artificial Societies and Social Simulation 19 (4), 2: https://www.jasss.org/19/4/2.html. [doi:10.18564/jasss.3161]
BYRNE, D. (1971). The Attraction Paradigm. New York: Academic Press.
CHEMERO, A., (2013). Radical embodied cognitive science. Review of General Psychology, 17(2): 145-150. [doi:10.1037/a0032923]
CIALDINI, R.B., and Goldstein, N.J., (2004). Social influence: Compliance and conformity. Annual Review of Psychology, 55 (1): 591–621. [doi:10.1146/annurev.psych.55.090902.142015]
CIALDINI, R.B., Kallgren, C.A. & Reno, R.R. (1991). 'A focus theory of normative conduct: a theoretical refinement and reevaluation of the role of norms in human behavior'. In: M.P. Zanna (Ed.). Advances in Experimental Social Psychology, 24: 201–234. San Diego, CA: Academic. [doi:10.1016/s0065-2601(08)60330-5]
CIALDINI, R.B., Reno, R.R. & Kallgren, C.A. (1990). A focus theory of normative conduct: Recycling the concept of norms to reduce littering in public places. Journal of Personality and Social Psychology, 58(6), 1015. [doi:10.1037/0022-3514.58.6.1015]
CONTE, R., Andrighetto, G. & Campennì, M. (2013). Minding Norms: Mechanisms and Dynamics of Social Order in Agent Societies. Oxford University Press. [doi:10.1093/acprof:oso/9780199812677.001.0001]
CONTE, R., Giardini, F., (2016). Towards Computational and Behavioral Social Science. European Psychologist, 21(2), 131-140 [doi:10.1027/1016-9040/a000257]
CONTE, R. & Castelfranchi, C. (1995). Cognitive and social action. London: University College of London Press.
CONTE, R. & Paolucci, M. (2001). Intelligent social learning. Journal of Artificial Societies and Social Simulation, 4(1), 3: https://www.jasss.org/4/1/3.html.
CONTE, R. & Paolucci, M. (2002). Reputation in artificial societies: Social beliefs for social order. Dordrecht: Kluwer Academic. [doi:10.1007/978-1-4615-1159-5]
DECI, E.L., & Ryan, R.M. (2000). The "What" and "Why" of Goal Pursuits: Human Needs and the Self-Determination of Behavior. Psychological Inquiry,11 (4), 227-268. [doi:10.1207/S15327965PLI1104_01]
DELRE, S. A., Jager, W., Bijmolt, T. H., & Janssen, M. A. (2007). Targeting and timing promotional activities: An agent-based model for the takeoff of new products. Journal of Business Research, 60(8), 826-835. [doi:10.1016/j.jbusres.2007.02.002]
DIGNUM, F., Dunin-Keplicz, B., Verbrugge, R., (2001). Creating collective intention through dialogue. Logic Journal of the IGPL, 9 (2), 305-319. [doi:10.1093/jigpal/9.2.289]
DIJKSTERHUIS, A., Bos, M. W., Nordgren, L. F., & Van Baaren, R. B. (2006). On making the right choice: The deliberation-without-attention effect. Science, 311(5763), 1005-1007. [doi:10.1126/science.1121629]
DYKSTRA, P., Jager, W., Elsenbroich, C., Verbrugge, R., & de Lavalette, G. R. (2015). An agent-based dialogical model with fuzzy attitudes. Journal of Artificial Societies and Social Simulation, 18(3), 3: http://www.soc.surrey.ac.uk/JASSS/18/3/3.html. [doi:10.18564/jasss.2813]
EDMONDS, B. and Moss, S. (2005). From KISS to KIDS – an ‘anti-simplistic’ modelling approach. In P. Davidsson et al. (Eds.): Multi Agent Based Simulation 2004. Springer, Lecture Notes in Artificial Intelligence, 3415:130–144.
ELSENBROICH, C., & Gilbert, N. (2014). Modelling norms. Berlin, Germany: Springer. [doi:10.1007/978-94-007-7052-2]
ELIASMITH, C, Stewart, T.C., Choo, X., Bekolay, T., DeWolf, T., Tang, Y., Rasmussen, D., (2012). A Large-Scale Model of the Functioning Brain. Science338 (6111), 1202-1205
ERNST, A.M. (1998). 'Resource dilemmas, computer simulated actors and climate change – a methodology to investigate human behavior in a complex domain'. In: E.A. Stuhler and D. De Tombe (Eds.), Complex problem solving - Cognitive psychological issues and environmental policy applications. München: Hampp press.
ERNST, A. (2010). 'Social simulation: A method to investigate environmental change from a social science perspective'. In M. Gross & H. Heinrichs (Eds.), Environmental Sociology: European Perspectives and Interdisciplinary Challenges (pp. 109-122). Berlin: Springer. [doi:10.1007/978-90-481-8730-0_7]
FAZIO, R. H. (2007). Attitudes as object-evaluation associations of varying strength. Social Cognition, 25(5), 603. [doi:10.1521/soco.2007.25.5.603]
FESTINGER, L. (1954). A theory of social comparison processes. Human Relations, 7, 117-140. [doi:10.1177/001872675400700202]
FORTUNATO V.J, Furey, J.T. (2012). An Examination of Thinking Style Patterns as a Function of Thinking Perspective Profile. Personality and Individual Differences, 2012 (53), 849–856. [doi:10.1016/j.paid.2012.06.017]
GOTTS, N. M. and Polhill, J. G., (2009). When and How to Imitate Your Neighbours: Lessons from and for FEARLUS. Journal of Artificial Societies and Social Simulation 12(3), 2: https://www.jasss.org/12/3/2.html.
HEGSELMANN, R. and Krause, U., (2002). Opinion dynamics and bounded confidence: models, analysis and simulation. Journal of Artificial Societies and Social Simulation, 5(3), 2: https://www.jasss.org/5/3/2.html .
HEISE, D.R. (2013). Modeling Interactions in Small Groups. Social Psychology Quarterly, 76(1), 52–72. [doi:10.1177/0190272512467654]
HOPFENSITZ, A., Reuben, E., (2009). The importance of emotions for the effectiveness of social punishment. Economic Journal, 119, pp. 1534–1559. [doi:10.1111/j.1468-0297.2009.02288.x]
HU, X., & Keller, N., (2015). Agent-Based Modeling and Simulation of Child Maltreatment and Child Maltreatment Prevention. Journal of Artificial Societies and Social Simulation, 18 (3), 6: https://www.jasss.org/18/3/6.html. [doi:10.18564/jasss.2783]
INFANTE, D.A., Rancer, A.S., Womack, D. F. (1997). Building Communication Theory. Illinois: Prospect Heights.
JACKSON, J. C., Rand, D.G., Lewis, K., Norton, M.I., Gray, K., (forthcoming). Agent Based Modeling: A Guide for Social Psychologists (December 5, 2016). Social Psychology and Personality Science (Forthcoming). Available at SSRN: https://ssrn.com/abstract=2880587
JAGER, W., & Amblard, F. (2005). Uniformity, bipolarization and pluriformity captured as generic stylized behavior with an agent-based simulation model of attitude change. Computational & Mathematical Organization Theory, 10(4), 295-303. [doi:10.1007/s10588-005-6282-2]
JAGER, W., & Amblard, F. (2007). 'Guess you’re right on this one too: Central and peripheral processing in attitude changes in large populations'. Advancing Social Simulation: The First World Congress, 249-260. [doi:10.1007/978-4-431-73167-2_23]
JAGER, W., Ernst, A., (2017). Social Simulation in Environmental Psychology, Introduction of the special issue. Journal of Environmental Psychology, 52.
JAGER, W., Janssen, M. A., De Vries, H. J. M., De Greef, J., & Vlek, C. A. J. (2000). Behaviour in commons dilemmas: Homo economicus and Homo psychologicus in an ecological-economic model. Ecological Economics, 35(3), 357-379. [doi:10.1016/S0921-8009(00)00220-2]
JANSSEN, M. A. (2006). Evolution of cooperation when feedback to reputation scores is voluntary. Journal of Artificial Societies and Social Simulation, 9(1), 17: https://www.jasss.org/9/1/17.html.
KANGUR, A., Jager, W., Verbrugge, R., & Bockarjova, M., (2017). Hybrid or full electric, that’s the question: An agent-based model exploring the diffusion of electric vehicles. Journal of Environmental Psychology, 52. [doi:10.1016/j.jenvp.2017.01.002]
KEIZER, K., Lindenberg, S. & Steg, L. (2011). The reversal effect of prohibition signs. Group Processes & Intergroup Relations, 14(5), 681–688. [doi:10.1177/1368430211398505]
KENRICK, D. T., Griskevicius, V., Neuberg, S. L., & Schaller, M. (2010). Renovating the pyramid of needs: Contemporary extensions built upon ancient foundations. Perspectives on Psychological Science, 5, 292-314. [doi:10.1177/1745691610369469]
KOTTONAU, J., and Pahl-Wostl, C., (2004). Simulating political attitudes and voting behavior. Journal of Artificial Societies and Social Simulation, 7 (4), 6: https://www.jasss.org/7/4/6.html.
LAIRD, J. E., A. Newell & P. S. Rosenbloom. 1987. Soar: An architecture for general intelligence. Artificial Intelligence 33(1). 1–64. [doi:10.1016/0004-3702(87)90050-6]
LINDENBERG, S. & Steg, L. (2007). Normative, gain and hedonic goal frames guiding environmental behavior. Journal of Social Issues, 63(1), 117–137. [doi:10.1111/j.1540-4560.2007.00499.x]
MACKINNON, Neil J. (1994). Symbolic Interactionism as Affect Control. Albany, NY: State University of New York Press.
MASLOW, A. H. (1954). Motivation and personality. New York: Harper and Row.
MAX-NEEF, M. (1992). Development and human needs. In P. Ekins, & M. Max-Neef (Eds.), Real-life economics: Understanding wealth creation (197-213). London, New York: Routledge.
MOSLER, H.J., Schwarz, K., Ammann, F., Gutscher, H., (2001). Computer Simulation as a Method of Further Developing a Theory: Simulating the Elaboration Likelihood Model. Personality and Social Psychology Review, 5(3), 201 - 215. [doi:10.1207/S15327957PSPR0503_2]
MOYO, D., Ally, A. K., Brennan, A., Norman, P., Purshouse, R. C. and Strong, M. (2015). Agile Development of an Attitude-Behaviour Driven Simulation of Alcohol Consumption Dynamics. Journal of Artificial Societies and Social Simulation 18 (3), 10: https://www.jasss.org/18/3/10.html. [doi:10.18564/jasss.2841]
NYBORG, K., Anderies, J.M., Dannenberg, A., Lindahl, T., Schill, C., Schlüter, M., Adger, W.N., Arrow, K.J., Barrett, S., Carpenter, S., Chapin III, S.F., Crépin, A.S., Daily, G., Ehrlich, P., Folke, C., Jager, W., Kautsky, N., Levin, S.A., Madsen, O.J., Polasky, S., Scheffer, M., Walker, B., Weber, E.U., Wilen, J., Xepapadeas, A., De Zeeuw, A., (2016). Social norms as solutions: Policies may influence large-scale behavioral tipping. Science, 07 Oct 2016: Vol. 354, Issue 6308, 42-43. [doi:10.1126/science.aaf8317]
PAVLOV, I.P. (1927). Conditioned reflexes. New York: Oxford University Press.
PETTY, R.E., Cacioppo, J.T., (1986). Communication and persuasion: central and peripheral routes to attitude change. New York: Springer Verlag. [doi:10.1007/978-1-4612-4964-1]
POLHILL, J.G. (2015). Extracting OWL Ontologies from Agent-Based Models: A Netlogo Extension. Journal of Artificial Societies and Social Simulation, 18 (2), 15: https://www.jasss.org/18/2/15.html [doi:10.18564/jasss.2810]
POLHILL, J.G., Gotts, N.M. and Law, A.N.R., (2001). Imitative versus non-imitative strategies in a land use simulation. Cybernetics and Systems, 32(1-2), 285-307. [doi:10.1080/019697201300001885]
RANGONI, R., and Jager, W. (2017): Social dynamics of littering and adaptive cleaning strategies explored using agent based modelling. Journal of Artificial Societies and Social Simulation, 20 (2), 1: https://www.jasss.org/20/2/1.html. [doi:10.18564/jasss.3269]
ROGERS, E. M. (1993). Diffusion of innovations. 4th ed. New York: The Free Press.
SALZARULO, L. (2006). A Continuous Opinion Dynamics Model Based on the Principle of Meta-Contrast. Journal of Artificial Societies and Social Simulation, 9(1), 13: https://www.jasss.org/9/1/13.html.
SAWYER, R.K., (2004). Social explanation and computational simulation. Philosophical Explorations, 7(3), pp. 219–231. [doi:10.1080/1386979042000258321]
SCALCO, A., Ceschi, A., Shiboub, I., Sartori, R., Frayret, J.M. & Dickert, S., (2017). 'The Implementation of the Theory of Planned Behavior in an Agent-Based Model for Waste Recycling: A Review and a Proposal'. In: A. Alonso-Betanzos, N. Sánchez-Maroño, O. F. Romero, G. Polhill, T. Craig, J. Bajo, & J. M. Corchado (Eds.), Agent-Based Modeling of Sustainable Behaviors. Switzerland: Springer International Publishing. [doi:10.1007/978-3-319-46331-5_4]
SCHELLING, T.C., (1971). Dynamic models of segregation. Journal of Mathematical Sociology, 1, 143-186. [doi:10.1080/0022250X.1971.9989794]
SCHLÜTER, M., Baeza, A., Dressler, G., Frank, K., Groeneveld, J., Jager, W., Janssen, M.A., McAllister, R.R.J., Müller,B., Orach, K., Schwarz, N., & Wijermans, N. (2017). A framework for mapping and comparing behavioural theories in models of social-ecological systems. Ecological Economics, 131, 21–35. [doi:10.1016/j.ecolecon.2016.08.008]
SCHRÖDER, T., Wolf, I., (2017). Modeling multi-level mechanisms of environmental attitudes and behaviours: The example of carsharing in Berlin. Journal of Environmental Psychology, 52.
SCHWARZ, N. & Ernst, A., (2009). Agent-based modeling of the diffusion of environmental innovations — An empirical approach. Technological Forecasting and Social Change, 76(4), 497-511 [doi:10.1016/j.techfore.2008.03.024]
SHERIF, M. and Hovland, C.I. (1961). Social Judgment. Yale University Press, New Haven, CT.
SKINNER, B.F. (1953). The behavior of organisms. New York: Appleton-Century-Crofts.
SPEELMAN, E.N. (2014). Gaming and simulation to explore resilience of contested agricultural landscapes. Wageningen University, ISBN 9789461739049.
SQUAZZONI, F., and Casnici, N., (2013) 'Is Social Simulation a Social Science Outstation? A Bibliometric Analysis of the Impact of JASSS'. Journal of Artificial Societies and Social Simulation, 16(1), 10: https://www.jasss.org/16/1/10.html. [doi:10.18564/jasss.2192]
STEFANELLI, A. and Seidl, R. (2017). Opinions on contested energy infrastructures: An empirically based simulation approach. Journal of Environmental Psychology, 52.
SUN, R. (1997) Learning, action, and consciousness: A hybrid approach toward modelling consciousness. Neural Networks, 10(7), 1317–1331. [doi:10.1016/S0893-6080(97)00050-6]
SUN, R. (2006). 'The CLARION cognitive architecture: Extending cognitive modeling to social simulation'. In R. Sun (ed.), Cognition and multi-agent interaction: From cognitive modeling to social simulation, 79–102. Cambridge: Cambridge University Press.
SUN, R. (2012). Grounding Social Sciences in Cognitive Sciences. MIT Press: London.
TAKÁCS K, Flache A, Mäs M (2016) Discrepancy and Disliking Do Not Induce Negative Opinion Shifts. PLoS ONE, 11(6): e0157948. [doi:10.1371/journal.pone.0157948]
VAN ECK, P. S., Jager, W., & Leeflang, P. S. (2011). Opinion leaders' role in innovation diffusion: A simulation study. Journal of Product Innovation Management, 28(2), 187-203. [doi:10.1111/j.1540-5885.2011.00791.x]
THAGARD, P. (2012). 'Cognitive architectures'. In K. Frankish & W. Ramsay (Eds.), The Cambridge handbook of cognitive science (pp. 50-70). Cambridge: Cambridge University Press. [doi:10.1017/CBO9781139033916.005]
TUCKER, P., Smith, D., (1999). Simulating Household Waste Management Behaviour, Journal of Artificial Societies and Social Simulation 2(3), 3: https://www.jasss.org/2/3/3.html.
TURNER, J. C., Hogg, M. A., Oakes, P. J., Reicher, S. D. and Wetherell, M. S. (Eds.) (1987). Rediscovering the social group: A self-categorization theory, Blackwell, Oxford.
VASILJEVSKA, J., Douw, J., Mengolini, A., and Nikolic, I., (2017). An Agent-Based Model of Electricity Consumer: Smart Metering Policy Implications in Europe. Journal of Artificial Societies and Social Simulation, 20(1), 12: https://www.jasss.org/20/1/12.html. [doi:10.18564/jasss.3150]
WATTS, D.J. (1999). Small Worlds: The Dynamics of Networks Between Order and Randomness. Princeton: Princeton University Press.
WRAY, R. E. & R. M. Jones (2006). 'Considering Soar as an agent architecture'. In R. Sun (ed.), Cognition and multi-agent interaction: From cognitive modeling to social simulation, 53–78. Cambridge: Cambridge University Press.