Introduction
Social simulation methods have recently been applied to substance use phenomena through the development of agent-based models (Perez et al. 2005; Gorman et al. 2006; Lamy et al. 2011; Hoffer et al. 2012; Perez et al. 2012; Bobashev et al. 2014; Lamy et al. 2011). This type of simulation allows researchers to embed characteristics of research participants into virtual entities (called 'agents') and to recreate their trajectories of drug engagement inside an artificial society. In this virtual context, agents interact with their environment and other agents in an autonomous and dynamic way (Wooldridge 1995; Ferber 1998; Gilbert 2008). These models provide the capacity to examine how changes in key factors related to substance use (e.g., public policies, drug market characteristics) shape the substance use and related ‘trajectories’ of agents allowing simulation of the effects of these changes in real life.
In this study we developed an agent-based model of key aspects of methamphetamine use in Melbourne, Australia’s second largest city, and how these shape experience of harms and use of treatment services. Methamphetamine (methylamphetamine) is a synthetic psychostimulant that can produce a wide range of effects valued by the drug’s consumers, including euphoria, enhanced self-confidence and increased alertness when consumed (Feldman et al. 1997). However, use of the drug has also been associated with a range of physical (e.g., teeth grinding, cardiac arrhythmia, stroke) and psychological (acute psychosis, depression and anxiety) harms (Julien et al. 2008). These adverse consequences appear gradually as the results of iterated usages and can underpin individuals’ motivations for accessing treatment (Quinn et al. 2013a). In this context, this study’s simulation aimed to reproduce trajectories of methamphetamine use and identify elements shaping such trajectories to more comprehensively investigate utilisation of drug treatment by methamphetamine users over time. With these trajectories were modelled, the final goal of this research was to identify the difficulties (i.e., barriers) that methamphetamine users searching for treatment can experience and to assess innovative and evidence-based strategies to improve their access to relevant treatment services.
Surveys suggest that, after cannabis, methamphetamine is the second most frequently used illicit drug in Australia with an estimated 2.1% of the Australian population above 14 years old reporting past-year use of the drug (AIHW 2014). This figure has remained relatively stable since 2007 and includes those who may report only infrequent use of the drug (Lim et al. 2015). The precise number of people who use methamphetamine (hereafter termed 'methamphetamine users') and experience problems is unknown, but McKetin and colleagues estimated that there were 102,000 ‘dependent’ and/or ‘regular’ methamphetamine users in 2002/2003 (McKetin et al. 2005). However, few of these people receive treatment for their use in a given year (Quinn et al. 2013b).
Despite this relative stability in population-level estimates of use, indices of methamphetamine-related harms have been increasing in parts of Australia. For example, increases in both the number of methamphetamine-related ambulance attendances and treatment episodes have been documented in Victoria (Hellbroon et al. 2013). In the mainstream media these increases have typically been attributed to escalating use among the general population (Lim et al. 2015), but recent research suggests that they have occurred in the context of dramatic changes in the methamphetamine market, in which a sharp decline in the purity-adjusted price of the drug has been observed (Scott et al. 2015). These market changes may underpin the increased methamphetamine-related harms that have been observed in Victoria; in this context, the concurrent rise in methamphetamine-related treatment episodes is unsurprising given previous research which indicated that treatment utilisation is associated with greater experience of methamphetamine-related consequences (Quinn et al. 2013a).
This paper describes the development of TreatMethHarm, an agent-based model of methamphetamine use and related service utilisation that draws on data collected during the course of a wider project conducted in Melbourne during 2009-2012. This wider project collected both qualitative (Duff et al. 2014) and quantitative/epidemiologic data that were used to develop and parameterise the key features of the model. In the first section we describe the conceptual model and its principal components; the second section presents the calibration of the model by testing key model parameters and shows the evolution of our population of methamphetamine users; lastly, the third section discusses the outcomes and limitations of TreatMethHarm in its final form, and the potential for its future use in modelling policy scenarios.
Modelling methamphetamine use and access to treatment
The two main objectives of the conceptual model are to reproduce (1) the evolution of a known population of methamphetamine users and (2) their access to service structures that can provide treatment or referral into treatment. The work was underpinned by the epidemiological data collected during the baseline and follow-up surveys of regular (at least monthly) methamphetamine users conducted for the wider study (Quinn et al. 2013a; Quinn et al. 2013b).
At a basic level, these data indicated that all participants reported use of additional drugs (e.g., cannabis, heroin, alcohol). Further, 62% were classified as ‘methamphetamine-dependent’ according to the Severity of Dependence Scale (SDS) and almost 60% reporting injecting the drug at baseline. A large minority (41%) of participants could be described as ‘service-avoiders’ (i.e., they considered any form of treatment or support service to be unnecessary), while 59% were described as ‘service-inclined’ (i.e., participants who described their use as problematic or harmful).
In TreatMethHarm, each agent or user represents a methamphetamine user characterised by a set of individual attributes. The two most important attributes are Stage of use (defined by frequency of consumption and other drug use patterns) and health attributes (defined through parameters related to agents’ overall physical and psychological states). Users also display a set of ‘belief’ attributes characterising the opinions users have of methamphetamine and related treatment services. These different beliefs underpin the decisions agents make concerning their consumption and choices regarding both treatment and referral mechanisms (e.g., police, general practitioners (GPs), social services).
In addition to their attributes, users are part of a social network of users randomly chosen from the population. In the model, networks shape the judgment and actions of their members (see below). Furthermore, TreatMethHarm displays a range of locations representing the different support services and settings that users might access throughout their career. The next section presents the main components shaping the trajectory of users in TreatMethHarm and how changes in agent trajectories can in turn affect their own choices as well as the actions of other agents.
Stages of methamphetamine use
The trajectory of a given methamphetamine user was modelled within a hierarchy of four use ‘stages’ defined by frequency of use and a related set of practices (Becker 1953; Abbott 2001). The data collected during the epidemiological survey suggested three main stages of methamphetamine use within the cohort:
- Occasional users (stage 1 or S1) – users who consume methamphetamine less than twice a week and smoke/snort it;
- Frequent users (stage 2 or S2) – users who consume methamphetamine at least three times a week; and
- Problematic users (stage 3 or S3) – individuals with daily or almost daily methamphetamine consumption and inject drugs.
These three stages and their related frequency of use are implemented in the model as attributes. TreatMethHarm also includes users at S0, who are potential ‘experimenters’ or previous users (who can return to use). S0 agents smoke/snort methamphetamine and use methamphetamine infrequently (less than weekly).
One factor shaping the trajectory of methamphetamine users is tolerance. Tolerance of methamphetamine-related effects results from regular and repeated use; as tolerance increases, users consume more of the substance (Feldman et al. 1997) and/or will switch to other routes of administration in order to obtain the desired effects (consistent with the high frequency of use among Australian methamphetamine users in the context of escalating drug purity (AIHW 2014; Scott et al. 2015). Higher consumption as well as more harmful methods of administration increase the risks of adverse health effects, which, in turn, increases the likelihood of seeking treatment.
To integrate tolerance in agents’ decision, we created a stage attribute describing the degree of tolerance experienced by the agents. This attribute defines their frequency of use and affects some of their actions (see below).
To capture the gradual development of tolerance in TreatMethHarm, each virtual user remembers its consumption per day (through the memuse-day attribute) and per week (memuse-week attribute). They check their memuse-week score to evaluate their tolerance and may, in turn, modify their stage attribute weekly, but cannot move more than one stage per week within the model cycle. This constraint means, for example, that S3 users cannot return to S0 before a minimum of three weeks. Agents, therefore, memorise their consumption of the past three weeks (through the memuse-week2, memuse-week3 and memuse-week4 attributes). The stage dynamic is based on the consumption frequency data drawn from our epidemiological data and is presented in Fig.1.
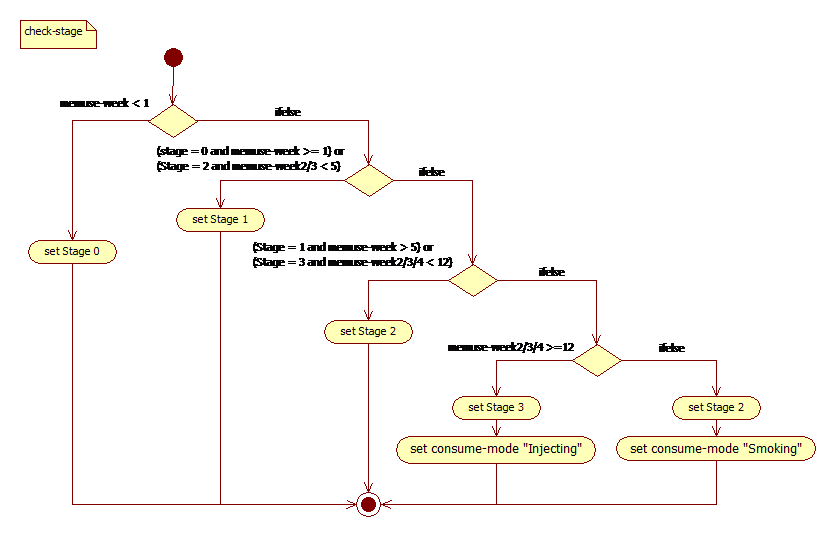
This stage dynamic mainly depends on; (1) the decision to consume, as this determines the frequency of use, and (2) on the perception of related harms, as this determines the frequency with which users seek support services. These two processes are presented in the two next subsections.
Decision to consume
People engage in methamphetamine use for a variety of reasons, including seeking pleasure, avoiding pain and as part of daily routines (e.g., Newton et al. 2009) with tolerance increasing with frequency of use (Cho and Melega 2002). In our epidemiological work, most participants reported that ‘pleasure seeking’ (e.g., for sex or ‘fun’) was a primary motivation for methamphetamine use, along with ‘craving’ and ‘out of habit’. Based on our findings and the relevant literature, methamphetamine use in TreatMethHarm is shaped by pleasure seeking and growing tolerance.
Pleasure seeking
Pleasure seeking is a primary driver of consumption in TreatMethHarm. The user’s decision to consume methamphetamine for pleasure is reproduced by the interaction of three main elements: a degree of curiosity toward methamphetamine; the user’s tolerance; and the general acceptance and opinion of the virtual agent population.
The first element, called initialPleasure (iP), represents an agent's initial interest in the substance. This characteristic is randomly attributed to each user using a normal distribution (μ = 1, σ = 0.4, range [0-2]) and does not change during the simulation. A low value of iP defines a low desire for methamphetamine effects, whereas a high value indicates high interest in its effects. The second element, tolerance, is introduced here to reflect a gradual adaptation to the effects of the substance. Tolerance is characterised by the stage attribute of the agent. To calibrate the model, we introduced a %attempt variable. This value is initially chosen by the modeler and reflects the global patterns of methamphetamine consumption in a given population. The %attempt value is added to the initialPleasure of users, such that the higher the value of %attempt, the more likely users are to engage in methamphetamine use. By integrating these three elements, TreatMethHarm captures psychological and social factors shaping use.
Nevertheless, if pleasure is the main motivation to use methamphetamine, the decision depends ultimately on the meanings or beliefs that users associate with the substance (Blumer 1998). To represent these meanings, users are initially assigned a randomly distributed attribute, named meaningMeth, representing the primary meanings ascribed to methamphetamine (randomised normal distribution with μ = 1, σ = 0.4, and range [0, 2]). A high value of meaningMeth indicates a positive meaning associated with the substance and so a higher chance of consumption, whereas a low value indicates a decreased probability of consumption. In contrast to initialPleasure, this value evolves throughout the simulation to allow users to reevaluate the cultural meanings of methamphetamine on the basis of their own experiences as well as on the basis of their interactions with other Network members (see below). For example, users could negatively reevaluate the meaning of methamphetamine if their physical state deteriorates, if they experience an overdose, or if they observe detrimental effects of methamphetamine consumption among other Network members. Values of meaningMeth can become negative as a consequence of repeated negative experiences or interactions: this allows both cessation of methamphetamine use and subsequent return to use (should the social environment permit) to be represented in the simulation.
Based on these four elements, the overall probability of a user consuming methamphetamine for pleasure (Cp(x)) is equal to: Cp(x) = (iP * (Stage + 1) * meaningMeth * %attempt).
The probability of consumption increases for all users during the weekend (the value of %attempt is added to Cp) and users could ‘binge’ according to their stage attribute (Pbinge = Stage * 10). This consumption for pleasure is constrained by another attribute, %deterrence (see below), which represents the sum of previous bad experiences and the probability of the agents revising their decisions to use due to past negative experiences.
Growing tolerance
The second process of methamphetamine consumption in TreatMethHarm aims to reproduce users’ intensive consumption of methamphetamine. Integrating intensive consumption and growing tolerance was considered important given that 62% of the sample recruited into the epidemiological study were classified as dependent at first interview (Quinn et al. 2013b). We modeled this pattern of methamphetamine use as the interplay between users’ level of tolerance (stage) and the time spent without consuming methamphetamine (tAbstinent).
Research suggests that personal characteristics play a role in the path to dependency (Barr et al. 2006, Sussman et al. 2008). Although such characteristics such as a person’s biological makeup do not account for the full range of methamphetamine-related behaviours, it is important to represent predispositions to consumption in our model. In the absence of clear quantification of how these personal characteristics affect consumption, we integrated these predisposing characteristics [DM1] and their variation across the population by adding an attribute termed personalFactor to each user (randomised normal distribution, μ = 1, σ = 0.4, range [0,2]). This attribute modifies the probability of users consuming methamphetamine because of their personal responses to patterns of intensive use. The final probability to consume due to increased tolerance (Tc(x)) is equal to: Tc(x) = (Stage + 1) * personalFactor * tAbstinent.
Use resulting from tolerance is not subject to the value of meaningMeth, which means that high-stage agents will consume methamphetamine to relieve withdrawal symptoms despite negative opinions of the drug. The use sequence in the model first involves users checking their probability to consume for pleasure and, if this is negative, a check is then made to determine a user’s tolerance which can give rise to continued use despite the user having negative meanings regarding methamphetamine.
The pleasure seeking and tolerance-related use motivations combine to produce the overall methamphetamine use of individual users and the population as a whole. By repetitively consuming methamphetamine, users slowly increase their stage, and the potential for harm, due to more frequent use of larger doses of methamphetamine. The next subsection describes the main process through which users can reduce their consumption and, in turn, their stage in TreatMethHarm.
Individual harm and search for treatment
So far, the conceptual model allows agents to consume methamphetamine regularly, driven by a mix of motivations, depending on their attributes. The consumption of methamphetamine means that users gradually build a tolerance to the drug that induces an increase in both frequency and dosage of use. These increases generate higher risks of harms as well as higher probability of being arrested. In turn, these outcomes modify agents’ future decisions in a dynamic way. TreatMethHarm reproduces the impact of these negative outcomes through three main de-escalation processes: health-related deterrence, social sanction from peers and being arrested.
Health-related deterrence
Methamphetamine use is associated with short- and long-term physical and psychological harms. Methamphetamine users reduce their consumption and/or search for treatment when they become aware of the deleterious outcomes of their drug use (Quinn et al. 2013a). However, there is little evidence about the degree of harm that triggers this reaction, meaning that we have accommodated a large range of possible user health experiences related to methamphetamine in the model. The following subsection describes how TreatMethHarm attempts to capture users’ decisions to search for help through a range of four health-related variables.
In the model, users check their physical/psychological state at the beginning of each time step through a comparison of two attributes: initialHealth and currentHealth. InitialHealth is defined at the creation of the agent and does not vary during the simulation. This initial value is set as the result of a random normal distribution with μ = 60, σ = 5, and range = 45–75. Conversely, the currentHealth attribute indicates an agent’s health value throughout the simulation and could vary within the range 0–75. Users will search for support or treatment if they perceive a significant difference between these two values. This ‘significant difference’ is calculated by combining an individual feature with a global variable. The global variable is named health-threshold (range 1–40) and serves to calibrate the model. The individual element is represented by the %health attribute randomly distributed using a normal law distribution (μ = 1, σ = 0.4, range 0–2) as users are created prior to any simulation. This means that users with a low %health value will search for treatment even if their currentHealth value is only slightly decreased. Conversely, agents with a high %health value will be less inclined to search for treatment. By randomly distributing the %health attribute, we ensure a large range of reactions that is appropriate given the limited knowledge around the triggers for treatment mentioned above, which also avoids an unrealistically large movement of agents to treatment services that would arise if they all had the same attribute values.
Based on these four components, users will reduce their consumption and/or search for treatment according to the following condition: currentHealth < initialHealth – (%health * health-threshold).
Therefore, (%health * health-threshold) fluctuates between 0 and 80.
The value of currentHealth can vary in two ways. First, currentHealth values diminish each time a user consumes methamphetamine. Second, if users do not use methamphetamine for at least one time step, then their currentHealth value is increased by five at the beginning of the next time step.
Furthermore, in TreatMethHarm, methamphetamine consumption produces both short- and long-term changes in the physiology and meanings held by the agents. Research suggests that purity can have a major impact on methamphetamine-related harm users experience (Scott et al. 2015). In the short term, each dose of methamphetamine diminishes a user’s currentHealth value according to the drug's degree of purity (range 0–1) and to a harm-factor (range 0–2). These two variables are set by the modeller: the purity variable aims to represent the impact of drug potency on the behaviour of users, the harm-factor variable is created to calibrate the model. Users consume doses equal to their actual stage (stage + 1), which means that a S3 agent will consume four doses at each time step. We modelled currentHealth-related harm in the following way: currentHealth(t1) = currentHealth(t0) - ((Stage + 1) *Harm-factor)* epurity.
In addition to the harms regular methamphetamine use may induce, TreatMethHarm includes two patterns of consumption that can influence the production of harm. As constructed in TreatMethHarm (and underpinned by our epidemiological data), S3 users are inclined to inject the drug. This route of administration confers the potential for additional harms when compared to others (i.e., snorting, smoking, ingesting), for example, the risk of blood-borne virus infection (Crofts et al. 1999). Hence, the potential physical and psychological harms associated with injecting methamphetamine are multiplied by two in the simulation. Moreover, and as indicated above, any user that decides to consume could ‘binge’ on methamphetamine. If so, users consume twice their stage-related dose, increasing the user’s experiences of methamphetamine-related harms and frequency of use. The link between injecting and the experience of harms, the values for the consumption of users while bingeing, and the probability of bingeing were set arbitrarily and need further information to be accurately calibrated.
In the long term, repeated self-perceived harms generate a deterrent effect, represented by the %deterrence attribute (range 0–100). This attribute gradually increases during the simulation. Each time a given user sees its currentHealth reduced to a value inferior to 10 times its %health value it increases its %deterrence attribute value by 0.5. A high value of %deterrence prevents users from consuming for pleasure, as described previously.
Influences of peer networks
In TreatMethHarm, users are subject to peer influence through their interactions with other agents (Bauman et al. 1992; Ennett et al. 1993, Bahr et al. 2005). As indicated earlier, each user belongs to a network composed of an average of five agents. Networks influence their members by modifying their meaningMeth attributes and by assessing if their currentHealth status does not vary too much from the average health of other members.
If the value of one of these two attributes moves too far from the average value of the network, a given user could decide to (1) leave this network (it will then join a network with attribute values closer to its own), (2) search for ways to reduce methamphetamine use, or (3) cease use altogether. In relation to this, our epidemiological data showed that 30% of the sample who wished to change their use avoided official treatment services, and initiated a ‘self-detoxification’ or ‘detox’ period with the support of their peers (Quinn et al. 2013a). TreatMethHarm captures this as a period of abstinence through the self-detox method described below. So, in the model users will search for treatment or enter into a self-detox period if their currentHealth value is inferior to the mean of other members’ currentHealth minus the health-threshold: currentHealth(agent) < X currentHealth(network) – health-threshold
In the model, network mainly influences the users’ meaningMeth attribute. The accumulated negative outcomes of network members gradually reduce the average meaningMeth value of the network, modifying all members' belief-Meth. This change decreases, in turn, the probability for users to consume for pleasure (Cp(x)). Users re-evaluate their meaningMeth attribute regarding methamphetamine once a week.
Being arrested
In the current implementation of TreatMethHarm it is only perceptions of individual harms and group sanctions that drive users to seek support or treatment. Other rationales (e.g., maturing out, financial problems, negative social impacts linked to methamphetamine use) are not implemented at this stage, as they require further empirical data. Nevertheless, the epidemiological data suggests that approximately 40% of the initial sample was arrested in the 12 months prior to the survey (Quinn et al. 2013b). To reflect this possibility for users, TreatMethHarm includes %arrest, a variable related to the probability of users being arrested (range [0-100]). The probability for a given user to be arrested (Pa(x)) depends upon user stage, so the probability of being arrested is represented as: Pa(x) = %arrest * (Stage + 1).
At the end of any given period, the users have a probability equal to their %deterrence to reduce their meaningMeth attribute value by 0.2 and increase their %deterrence by 2, in order to mirror the impact of such events on users' future choices. Moreover, if arrested, users at a high stage of use are sent to the ‘Prison’ location before being dispatched to a treatment service. The different treatment services are presented below.
Treatment and service interfaces
To address the second objective of this research, namely to reproduce users’ access to service structures referring to or providing treatment, TreatMethHarm displays a set of ‘locations’ to represent potential paths of users searching for treatment. This section presents the features of the different interfaces and describes how users interact with them.
TreatMethHarm includes nine treatment-related locations: three ‘Referring Services’, the ‘Peers’ location, four ‘Treatment Services’ and one 'Prison’. These different options were chosen as a result of discussions between the modeler and illicit drug epidemiologists/experts in Victoria, in the context of Australia’s current treatment system and referral processes with a focus on methamphetamine (Quinn et al. 2013a; AIHW 2014). Each treatment service – namely, individual drug counseling (IDC), psychiatric treatment, rehabilitation and detox – has %success, max-population, %entry and duration attributes. The %success attribute defines the probability that a user will complete the treatment program and reduce its meaningMeth value; the max-population gives the maximum number of users that can be treated at the same time step; the %entry represents the probability for a given user to enter the treatment service; and the duration gives the number of time-steps the given agent will stay in treatment. The duration of each treatment has been calibrated accordingly to our (unpublished) epidemiological data, but the values of the three other variables are set arbitrarily and need further research to set appropriate values. Furthermore, in the actual version of the simulation, each user bears a representation associated to each form of treatment (i.e. beliefIDC, beliefPsyTreat, beliefDetox, and beliefRehab with a range equal to [-1, 1]) that constrains user decision regarding treatment. Users with a negative belief value will not enter related treatment. The other locations do not display these attributes; their main role is to dispatch the users searching for treatment to one of the treatment services described above. The referring services are ‘Gen.Pract’ (GP), ‘Soc.Service’ (social services), and ‘Police’. The agents are dispatched to a treatment service according to their stage and health. Users can enter a treatment service through three different mechanisms: professional help; self-detox; and be-arrested.
In the first instance, the user decides to search for professional help and goes through the referring services before entering a treatment service. The way users are oriented toward the various treatment services is depicted in Figure 2. Once in the appropriate service, the user modifies its Treatment attribute by indicating the type of treatment it is undergoing and its duration (based on the duration attribute of the service). During each time step spent in treatment, users progressively restore their health and, because they cannot consume, progressively decrease their stage. If the treatment is successful (probability based on the %success of the treatment), users reduce their meaningMeth by 0.2 and increase their %deterrence value by 2. They will also modify their perception of the treatment, increasing its value by 0.2. However, if the treatment fails, the different attributes do not change and the belief associated with the treatment (e.g. beliefIDC) is reduced by 0.2. At the moment, these views about treatment are shared across the networks, which implies that if a given agent experienced a negative outcome from a particular service, the belief attached to this treatment will diminish for all members belonging to the same network. If the user displays a negative view towards the support service, it will refuse to resume the treatment and will resort instead to ‘self-detox’.
Users that try to self-detox will spend (Stage + 1) * 7 time steps (equivalent to 7 to 28 days) without using methamphetamine. Again, this value was set arbitrarily and requires further research for validation. In contrast to the mechanism for treatment services described above, the probability of success is equal to the agent's %deterrence value plus 15%. This increase of 15% is arbitrary but aims to reflect the fact that the user has ‘consciously’ decided to reduce or stop consumption. As with treatment services, a successful self-detoxification leads to a reduction of the meaningMeth value and an increase of the %deterrence value.
Users can be arrested while consuming. The first time this happens for a given user they are moved to the ‘Police’ location. Depending on their arrest record, indexed through an attribute termed mem-arrest, as well as on the value of their stage and meaningMeth attributes, users will be oriented to specific treatment services. However, if the max-population of those services is reached, these users will be sent to the ‘Prison’ interface and will remain ‘in jail’ for (Stage +1) * 30 time steps without the possibility to consume methamphetamine. In the similar manner to arrested users (see above), these agents have a probability to reduce their meaningMeth attribute value and increase their %deterrence score at the end of their detention period.
This section has presented the core of TreatMethHarm functioning by detailing the evolution of the synthetic population of methamphetamine users and by describing their potential pathways to search for professional help. The next section presents the implementation of TreatMethHarm and its calibration.
Implementing and calibrating TreatMethHarm
The conceptual model was implemented in the multi-agent platform NetLogo© (Wilensky 1999) using a variation of the StarLogo© programming language (the global architecture of the model and attributes of the agents are presented in Appendix). This platform is widely used by social scientists aiming to reproduce socially complex systems because of the simplicity of the programming language, the user-friendly interface that includes a spatial environment visualisation, the possibility to directly act on the course of a simulation, and the capacity to observe outputs by plotting key parameters. The simulation runs on a daily time-step system to acknowledge the frequency of consumption of high-stage methamphetamine users. TreatMethHarm offers the possibility to run simulations with a ‘closed’ or ‘open’ system population: the modeller can choose to observe either a specific population of agents, or to run the simulation with the addition of newly generated agents at programmed intervals. Furthermore, as implemented, TreatMethHarm can be launched with a ‘non-blank’ population. Here the modeller can recreate a specific population by setting the proportions of users according to their stage at the simulation outset.
We first needed to calibrate the behavioural algorithms presented above. The description of the conceptual model presents a large number of variables, with some based on our epidemiological data and others randomised to favour heterogeneity amongst the users and avoid unrealistically large concurrent changes in the population. Throughout the construction of TreatMethHarm, we run hundreds of simulations to test the model outputs and ponder the effect magnitude of implemented variables. During that process, three of them (attempt, harm-factor and health-threshold) appeared to require particular attention due to their major impact on the trajectories of TreatMethHarm users. The parameter attempt controls the probability with which users increase their consumption for pleasure; harm-factor and health-threshold determine the moment when users will search for help and potentially enter treatment.
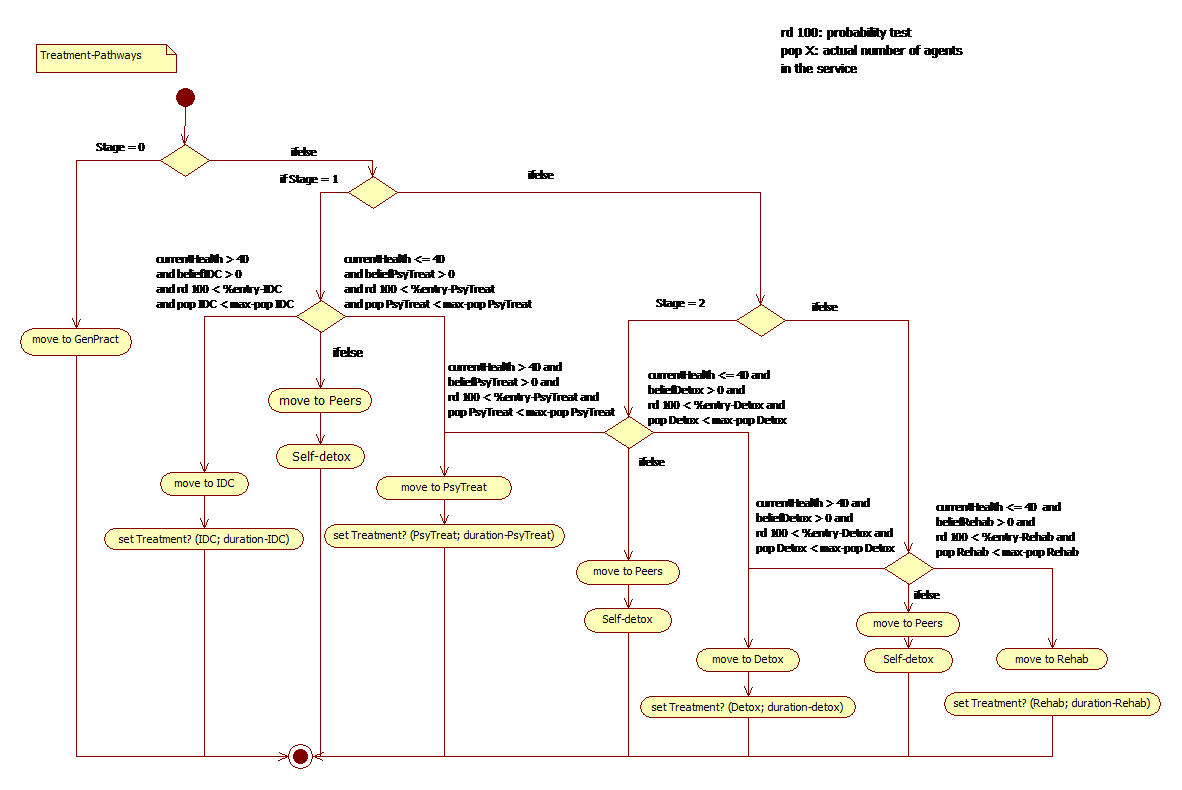
In this study, we used epidemiological data from our baseline and follow-up surveys to calibrate the evolution of the TreatMethHarm population. The original survey (N=255) was conducted in Melbourne from January to October 2010, with the follow-up survey administered 12 months later (n=201) (for more details, please refer to (Quinn et al. 2013a; Quinn et al. 2015). The initial proportions of agents per stage at t and t+1 are based on these empirical findings and are as follows (Table 1).
Stage (use per week) | t | t+365 |
---|---|---|
S0 (use < 1 time) | 15% | 54% |
S1 (5 times < use ≤ 1 time) | 26% | 22% |
S2 (14 times < use ≤ 5 times) | 39% | 15% |
S3 (use ≥ 14 times) | 20% | 9% |
We also aimed to reproduce the ratio of agents that sought professional help and the number abstinent at follow-up. Analyses of the follow-up data showed that 42 (21%) of the participants had accessed drug treatment (potentially for any drug problem) during the follow-up period, while 22% of the cohort indicated past-month abstinence at the follow-up interview.
The calibration scenarios were set with an initial population of 250 agents in a closed system population. Each scenario was repeated 50 times and each simulation ran for 365 time steps to reproduce the follow-up time gap. At the end of each simulation, we measured the mean values and standard deviations of the following output variables: the proportions of users in each stage (S0, S1, S2 and, S3), proportion of users that had sought treatment, and the proportion of users that were abstinent in the last 30 time steps (i.e., a simulated month). Our goal was to tune the parameters of the simulation to obtain outputs similar to the empirical data.
While building the model, we ‘pre-screened’ the reaction of the model by using ‘edge’ values to parameterise simulations (e.g., using values of 0% or 100%; harm-factor values of 0 or 4; health-threshold values of 0 or 60) and scaling down these values until the results approximated the observed empirical data. It rapidly appeared that high values of attempt led to an excessive representation of the S3 agents, while large health-threshold values increased the number of presentations for treatment and, in turn, the number of S0 agents. After these preliminary tests, we reduced the values to be tested to the following (Table 2).
Variables | Values Tested |
---|---|
Attempt | 10, 15, 20, 25, 30 |
Harm-factor | 0.8, 1, 1.2, 1.4 |
Health-Threshold | 10, 15, 20, 25, 30 |
The remaining parameters were unchanged for these calibration scenarios and were set as follows (Table 3).
Parameter | Value | Parameter | Value |
---|---|---|---|
%success-IDC | 66% | %entry-IDC | 66% |
%success-PsyTreat | 66% | %entry-PsyTreat | 66% |
%success-Rehab | 66% | %entry-Rehab | 66% |
%success-Detox | 66% | %entry-Detox | 66% |
Max-pop-IDC | 50 | Duration-IDC | 70 |
Max-pop-PsyTreat | 50 | Duration-PsyTreat | 60 |
Max-pop-Rehab | 50 | Duration-Rehab | 70 |
Max-pop-Detox | 50 | Duration-Detox | 70 |
Purity | 0.15 to 0.45 | Duration-Peers | (Stage+1) x 7 |
%arrest | 1 | Duration-Prison | (Stage+1) x 30 |
Deterrence | 1 |
To acknowledge the changes affecting the Melbourne methamphetamine drug market, we decided to set the purity parameter to mimic the evolution of the methamphetamine purity during the simulated period. The purity of methamphetamine was gradually increased by 2.5% per month to reproduce the changes in methamphetamine seizure purity observed in Victoria over the years 2008-2013 (Scott et al. 2015) from 15% to 45%. The remaining variables were set arbitrarily and require additional research for accurate parameterisation (see Table 5 in the Appendix for more information concerning the value source and importance of these variables).
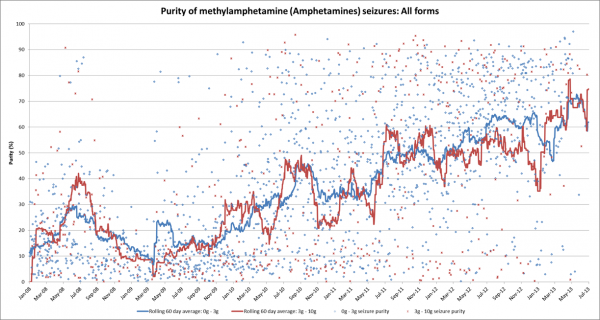
A comparison between TreatMethHarm outputs and empirical data allowed us to designate the scenario with the best goodness of fit. We found that the following set of values approximated the proportions of users in each stage observed in the epidemiological data, as well as the number of users that sought treatment and the number abstinent (i.e., users with no consumption for the last 30 ticks and currently not in treatment): attempt 15%, health-threshold 15, harm-factor 1.2 (see Table 4 for comparison).
Variables | Empirical | Simulated |
---|---|---|
Stage 0 (%) | 54 | 55 (SD 3) |
Stage 1 (%) | 22 | 21 (SD 2.5) |
Stage 2 (%) | 15 | 16 (SD 2.3) |
Stage 3 (%) | 9 | 8 (SD 1.8) |
UsedTreatment (%) | 21 | 22 (SD 3.2) |
Ex-users (%) | 22 | 19 (SD 3.2) |
Based on these values, we ran a base scenario and investigated the evolution of the user population. We used these values to run 100 replications of the simulation with 250 agents over a period of 365 time steps (equivalent to one year).
Figure 4 displays the evolution of our initial population of users. In the first few weeks, the whole population of users consumed methamphetamine regularly, increasing their tolerance to the drug and escalating in stage. After the first month, this trend was inverted and the number of S0 agents increased, while the number of S3 users tended to decline. After a period of initial fluctuation, the proportion of users at each of the different stages tended to equilibrium and stabilised after three months (equivalent to 100 time steps). Until the end of the simulated period, the number of S0 users continued to progressively increase with the number of S1, S2, and S3 decreasing accordingly.
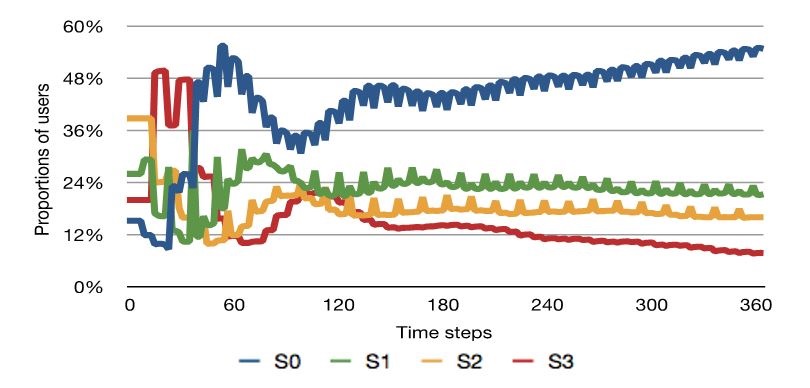
These fluctuations in the user population could be explained by observing the evolution of both meaningMeth and currentHealth values over time. Indeed, the movements between stages follow the evolution of the global health of the agents, shown in Figure 5. The first few weeks of the simulation sees the average health of the users drop markedly at several time-points, leading most of the users to either seek professional support or attempt self-detoxification at these points.
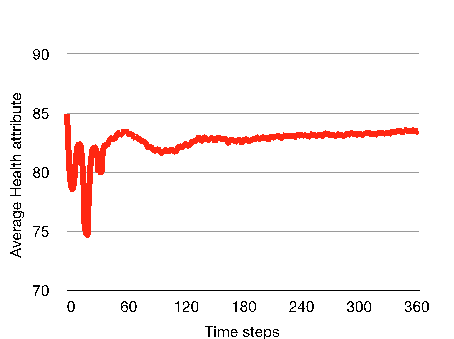
The recurrence of perceived harms induces a progressive shift in the global perception of the users regarding methamphetamine. This is illustrated in Figure 6, where the average value of meaningMeth steadily decreases after the first month.
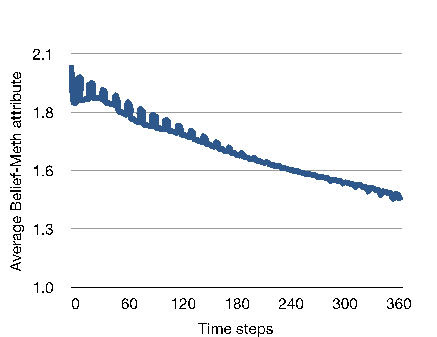
This slow but constant deterioration of meaningMeth is the result of the combined effect of the network (i.e., detrimental outcomes affecting one agent impacting on the global opinion of the other members) and of the increased deterrence toward the substance gradually built throughout the consumption of the users (the initial global averaged %deterrence is equal to 3% and reaches an average of 60.5% at the end of the simulations). This diminution in the global meaningMeth value is the main cause of the constant increase in number of S0 agents after the third month of simulation, as shown in Figure 4.
Discussion
Our agent-based model, TreatMethHarm, simulates the dynamics of methamphetamine use and subsequent use of treatment services in a virtual population of methamphetamine users. To the best of our knowledge, TreatMethHarm is the first simulation that mimics the trajectory of methamphetamine users and their access to treatment services. It is based on a range of variables aiming to reproduce characteristics of real users, such as growing tolerance, frequency/quantity of use and decisions, as well as network interactions and influence, and particularities of treatment settings. By combining these different components, we attempted to recreate the complexity inherent to methamphetamine use and to integrate access to treatment in the ‘careers’ of these users. The three core mechanisms of TreatMethHarm – the decision to consume (both for pleasure and due to growing tolerance), the decision to seek help, and treatment pathways – were detailed and combined to shape the trajectory of virtual agents. By using the right combination of parameters, we were able to generate proportions of users similar to the data collected during our empirical survey. Furthermore, by establishing a dialogue between researchers and modellers (Moore et al. 2009), and using the formal integrative tool of the model itself, we were able to identify gaps in the available literature and the limitations of the model. Future work needs to investigate these missing data to refine and complete TreatMethHarm. Indeed, the ‘abductive’ process (Agar 2005) underpinning the core functioning of this agent-based model allows researchers to reshape behavioural algorithms and modify key parameters as new information and conceptual understanding arises.
In its current iteration, TreatMethHarm has several limitations. The aggregated harms arising from the use of multiple drugs during the specified time period are not implemented despite their potential influence on both physical and psychological states of methamphetamine users. Moreover, the questions of the financial burden of such use as well as the availability of methamphetamine are not addressed in the model, as they require specific data on drug acquisition. The geographical dimension, in terms of distance and remoteness between users' living places and treatment services, is also absent from the model. Furthermore, during the development of the model, the meanings of methamphetamine users about the different support and treatment services appear pivotal to the decisions of the users. We implemented this by assuming that opinions regarding each service would vary according to the success of the treatment, and randomly distributed the treatment-related belief in order to palliate the lack of information regarding the initial representations and to integrate differences existing between ‘service-avoiders’ and ‘service-inclined’ (Quinn et al. 2013a). Nevertheless, further work is needed to collect information about how treatment services are viewed by service consumers, both initially as well as how they evolve during a drug use career. Similarly, it would also be useful to understand how users manage risks associated with use of the drug (including the extent of ‘risk denial’) that underpins not only decisions to use, but also decisions to avoid support services (Peretti-Watel 2003).
At this stage of its implementation, TreatMethHarm has been verified (i.e., debugged and calculation verified) and calibrated. Once the simulation has been developed further with better-informed values, we will move to further validate the model. However, such validation requires comparing simulated outputs with real-world data. In this context, there are few prospective studies of methamphetamine use, particularly outside of treatment service settings (Quinn et al. 2013a), meaning that further data collection on the trajectories of methamphetamine use in the community are required, which limits the actual capacity of TreatMethHarm to evaluate treatment strategies.
Conclusions
TreatMethHarm is an agent-based simulation that reproduces the trajectories of methamphetamine users with respect to their drug consumption and their experience of treatment services. Methamphetamine use and any related treatment utilisation is complex and results from the interplay of several key factors. Our model attempts to encompass these various factors by integrating diverse sources of data and best estimates. It is able to reproduce empirical data accurately but also highlights major gaps in knowledge around methamphetamine treatment in particular, and how users respond to meanings and harm, that need further research. In conclusion, TreatMethHarm is a mediator model, aiming to increase the knowledge concerning one specific phenomenon and offer a formal representation of a complex phenomenon (Heath et al. 2009). The next step of this project will consist of collecting and inserting the aforementioned missing data and recalibrating the model to validate it, once similar data, independent from our research, is available.
Acknowledgements
The research reported in this paper was funded by NHMRC Project Grant 479208. The National Drug Research Institute at Curtin University is supported by funding from the Australian Government under the Substance Misuse Prevention and Service Improvement Grants Fund. The authors gratefully acknowledge the contribution to this work of the Victorian Operational Infrastructure Support Program, the staff from Burnet Institute who assisted with the recruitment of the participants (especially, Cerissa Papanastasiou), Professor Pascal Perez and Georgiy Bobashev for their advices, as well as all the participants of this surveyAppendix
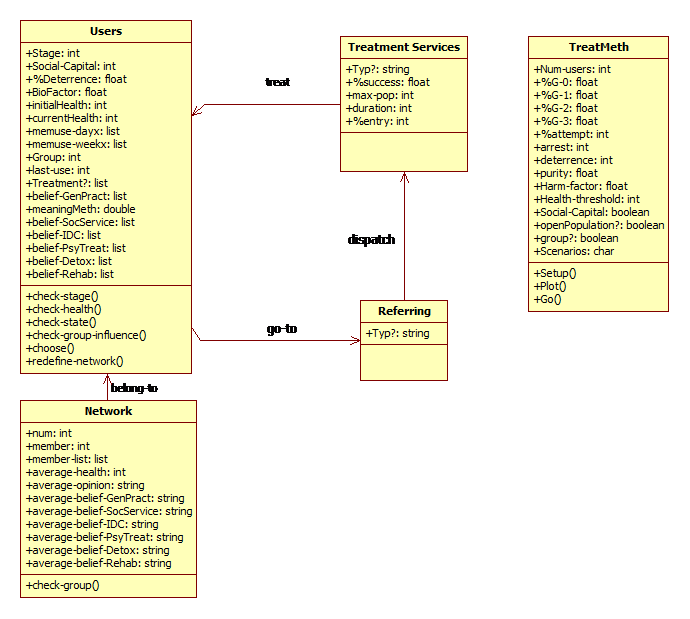
Variables | Degree of criticality | Source |
---|---|---|
%entry-IDC | Low | Arbitrary |
%entry-PsyTreat | Low | Arbitrary |
>%entry-Rehab | Low | Arbitrary |
>%entry-Detox | Low | Arbitrary |
>Duration-Peers | Low | Arbitrary |
Duration-Prison | Low | Arbitrary |
Duration-IDC | Low | Empirical |
Duration-PsyTreat | Low | Empirical |
Duration-Rehab | Low | Empirical |
%success-IDC | Moderate | Arbitrary |
%success-PsyTreat | Moderate | Arbitrary |
%success-Rehab | Moderate | Arbitrary |
%success-Detox | Moderate | Arbitrary |
Max-pop-IDC | Moderate | Arbitrary |
Max-pop-PsyTreat | Moderate | Arbitrary |
Max-pop-Rehab | Moderate | Arbitrary |
Max-pop-Detox | Moderate | Arbitrary |
%arrest | Moderate | Arbitrary |
Deterrence | Moderate | Arbitrary |
Attempt | Critical | Calibrated |
References
ABBOTT, A. (2001). Time Matters: On Theory and Method. Chicago, University of Chicago Press.
AGAR, M. (2005). Agents in Living Color: Towards Emic Agent-Based Models. Journal of Artificial Societies and Social Simulation, 8(1), 4: https://www.jasss.org/8/1/4.html
AIHW (2014). National Drug Strategy Household Survey detailed report: 2013. Drug statistics. Canberra, AIWH. 28.
BAHR, S. J., J. P. Hoffmann and X. Yang (2005). Parental and Peer Influences on the Risk of Adolescent Drug Use. The Journal of Primary Prevention 26(6), pp. 529-551.
BARR, A. M., W. J. Panenka, G. W. MacEwan, A. E. Thornton, D. J. Lang, W. G. Honer and T. Lecomte (2006). The need for speed: an update on methamphetamine addiction. Journal of Psychiatry and Neuroscience, 31(5), p. 301.
BAUMAN, K. E., G. J. Botvin, E. M. Botvin and E. Baker (1992). Normative expectations and the behavior of significant others: an integration of traditions in research on adolescents' cigarette smoking. Psychological Reports, 71, pp. 568-570.
BECKER, H. S. (1953). Becoming a Marihuana User. The American Journal of Sociology, 59(3), pp. 235-242. [doi:10.1086/221326]
BLUMER, H. (1998). Symbolic Interactionism: Perspective and Method. Berkeley, University of California Press.
BOBASHEV, G. V., E. Solano and L. Hoffer (2014). An agent-based model to support measuring drug choice and switch between drug types in rural populations. SIMUL, The Sixth International Conference on Advances in System Simulation. Nice, France.
CHO, A. K. and W. P. Melega (2002). Patterns of methamphetamine abuse and their consequences. Journal of Addictive Diseases, 21(1), pp. 21-34.
CROFTS, N., C. K. Aitken and J. M. Kaldor (1999). The force of numbers: why hepatitis C is spreading among Australian injecting drug users while HIV is not. Medical Journal of Australia, 170(5), pp. 220-221.
DUFF, C. and D. Moore (2014). Counterpublic health and the design of drug services for methamphetamine consumers in Melbourne. Health, 19(1), pp. 51-66.
ENNETT, S. T. and K. E. Bauman (1993). Peer Group Structure and Adolescent Cigarette Smoking: A Social Network Analysis. Joumal of Health and Social Behavior, 34, pp. 226-236. [doi:10.2307/2137204]
FELDMAN, R. S., J. S. Meyer and L. F. Quenzer (1997). Principles of Neuropsychopharmacology. Sunderland, Sinauer Associated.
FERBER, J. (1998). Multi-agents systems. MA, Addisson-Wesley.
GILBERT, N. (2008). Agent-based Models. Los Angeles, SAGE Publications.
GORMAN, D. M., J. Mezic, I. Mezic and P. J. Gruenewald (2006). Agent-Based Modeling of Drinking Behavior: A Preliminary Model and Potential Applications to Theory and Practice. American Journal of Public Health, 96(11), pp. 2055-2060. [doi:10.2105/AJPH.2005.063289]
HEATH, B., R. Hill and F. Ciarallo (2009). A Survey of Agent-Based Modeling Practices (January 1998 to July 2008). Journal of Artificial Societies and Social Simulation, 12(4), 9: https://www.jasss.org/8/1/4.html
HELLBROON, C., C. Gao, B. Lloyd, K. Smith, D. Best and D. Lubman (2013). Trends in amphetamine-related harms in Victoria. Medical Journal of Australia, 199(6), p. 395. [doi:10.5694/mja13.10149]
HOFFER, L., G. Bobashev and R. J. Morris (2012). Simulating patterns of heroin addiction within the social context of a local heroin market. Computational Neuroscience of Drug Addiction, Springer, pp. 313-331.
JULIEN, R. M., C. D. Advokat and J. E. Comaty (2008). A primer in drug action: a comprehensive guide to the actions, uses, and side effect of psychoactive drugs. New York, Worth Oublishers.
LAMY, F., P. Perez, A. Ritter and M. Livingston (2011). SimARC: An Ontology-driven Behavioural Model of Alcohol Abuse. SIMUL, The Third Conference, Barcelona, Spain.
LIM, M., S. Cogger, B. Quinn, M. Hellard and P. Dietze (2015). 'Ice epidemic'? Trends in methamphetamine use from three Victorian surveillance systems. Australian and New Zealand Journal of Public Health, 39(2), pp. 194-195. [doi:10.1111/1753-6405.12322]
McKETIN, R., J. McLaren and E. Kelly (2005). Estimating the number of regular and dependent methamphetamine users in Australia, National Drug and Alcohol Research Centre.
MOORE, D., A. Dray, R. Green, S. L. Hudson, R. Jenkinson, C. Siokou, P. Perez, G. Bammer, L. Maher and P. Dietze (2009). Extending drug ethno-epidemiology using agent-based modelling. Addiction, 104, pp. 1991-1997. [doi:10.1111/j.1360-0443.2009.02709.x]
NEWTON, T.F., R. De La Garza, A.D. Kalechstein, D. Tziortzis, C.A. Jacobsen (2009). Theories of addiction: methamphetamine users' explanations for continuing drug use and relapse. American Journal of Addiction, 18(4), pp. 294-300.
PERETTI-WATEL, P. (2003). Neutralization theory and the denial of risk: some evidence from cannabis use among French adolescents. British Journal of Sociology, 54(1), pp. 21-42. [doi:10.1080/0007131032000045888]
PEREZ, P., A. Dray, D. Moore, P. Dietze, G. Bammer, R. Jenkinson, C. Siokou, R. Green, S. L. Hudson and L. Maher (2012). SimAmph: An agent-based simulation model for exploring the use of psychostimulants and related harm amongst young Australians. International Journal of Drug Policy 23: 62-71.
PEREZ, P., A. Dray, A. Ritter, P. Dietze, T. Moore and L. Mazerolle (2005). Simdrug exploring the complexity of heroin use in Melbourne. Drug Policy Modelling Project Monograph Series, 11.
QUINN, B., M. Stoové and P. Dietze (2015). One-year changes in methamphetamine use, dependence and remission in a community-recruited cohort. Journal of Substance Use, pp. 1-8.
QUINN, B., M. Stoove, C. Papanastasiou and P. Dietze (2013a). An exploration of self-perceived non-problematic use as a barrier to professional support for methamphetamine users. International Journal of Drug Policy, 24, pp. 619-623. [doi:10.1016/j.drugpo.2013.05.015]
QUINN, B., M. Stoove, C. Papanastasiou and P. Dietze (2013b). Methamphetamine use in Melbourne, Australia: Baseline characteristics of a prospective methamphetamine-using cohort and correlates of methamphetamine dependence. Journal of Substance Use, 18(5), pp. 349-362.
SCOTT, N., J. P. Caulkins, A. Ritter, C. Quinn and P. Dietze (2015). High-frequency drug purity and price series as tools for explaining drug trends and harms in Victoria, Australia. Addiction, 110(1), pp. 120-128. [doi:10.1111/add.12740]
SUSSMAN, S. and S. L. Ames (2008). Drug Abuse: concepts, prevention, and cessation. Cambridge University Press.
WILENSKY, U. (1999). NetLogo. Northwestern University, Evanston, IL, Center for Connected Learning and Computer-Based Modeling.
WOOLDRIDGE, M. (1995). Intelligent Agents: Theory and Practice. Knowledge Engineering Review, 10(2), pp. 115-152.